Knowledge-Enhanced Multi-semantic Fusion for Concept Similarity Measurement in Continuous Vector Space
2015 IEEE 12th Intl Conf on Ubiquitous Intelligence and Computing and 2015 IEEE 12th Intl Conf on Autonomic and Trusted Computing and 2015 IEEE 15th Intl Conf on Scalable Computing and Communications and Its Associated Workshops (UIC-ATC-ScalCom)(2015)
摘要
Semantic similarity measurement is one of the significant components in the fields of information retrieval, artificial intelligence, etc. Also, it has been used in Internet of Things (IoT) to resolve the problems of inter-operability and integration of the heterogeneous resources such as ubiquitous services and distributed data, while increasing the accuracy of semantic similarity measurement is still a challenge. In this work, we propose a novel Multiple Semantic Fusion (MSF) model where various semantic properties and relationship information from knowledge base are encoded into the continuous distributed vectors derived from corpus. To capture and understand latent semantics of words, this model retrofits the semantic features in distributed representations and carries out a sense vector per word. On basis of the MSF model, we evaluate the semantic similarity between concepts by calculating the cosine similarity of the corresponding sense vectors. Our evaluation has been validated against different similarity methods in terms of Pearson correlation coefficient between computational results with human judgments. Finally, empirical evaluation results and discussions on various benchmark datasets are provided. Our finding provides a new perspective to understand and evaluated the word semantics.
更多查看译文
关键词
concept similarity,multiple semantic fusion,knowledge augmentation,continuous distributed vector
AI 理解论文
溯源树
样例
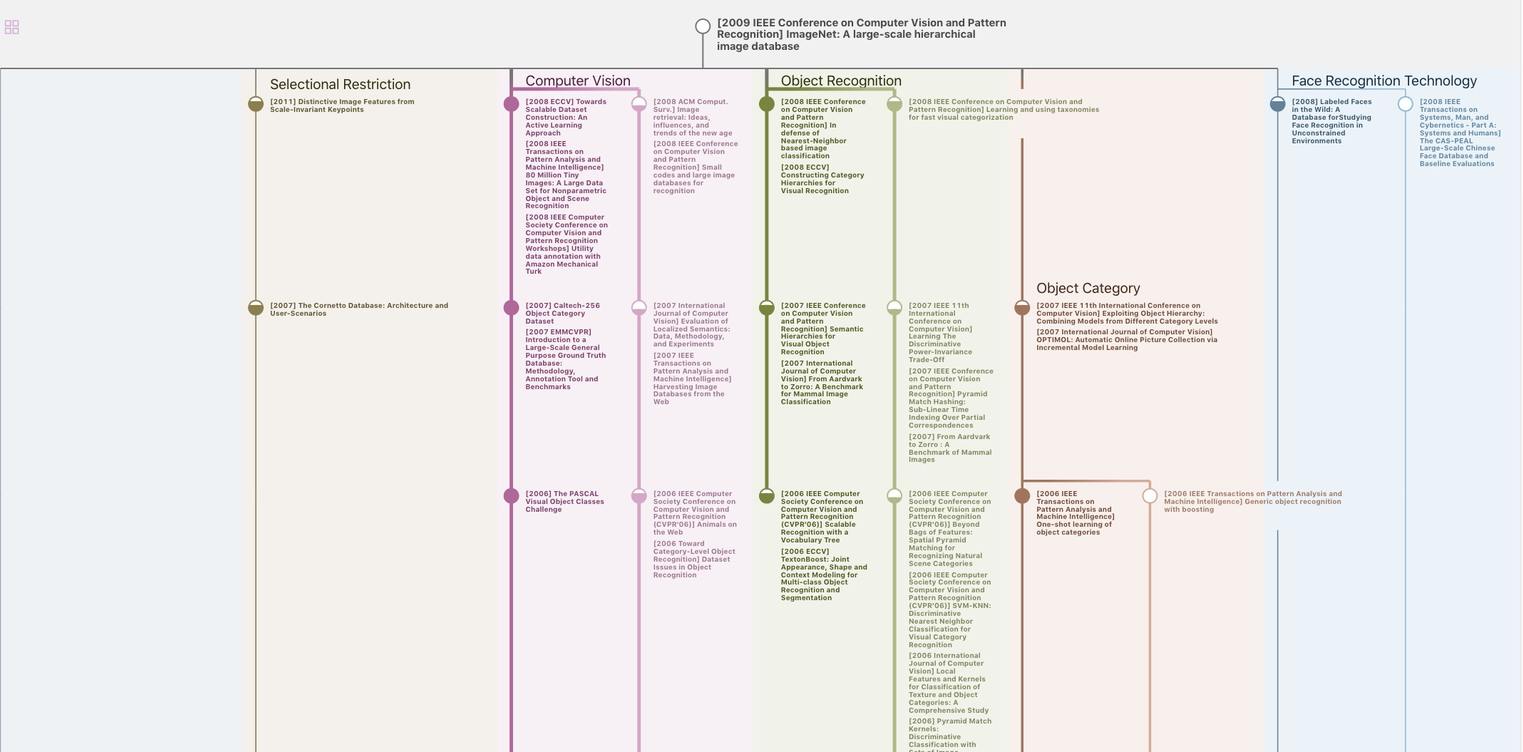
生成溯源树,研究论文发展脉络
Chat Paper
正在生成论文摘要