Motion Deblurring And Depth Estimation From Multiple Images
2016 IEEE International Conference on Image Processing (ICIP)(2016)
摘要
Scene depth variation is an important factor that leads to spatially-varying camera motion blur. Most of the previous methods require auxiliary cameras or user interaction to make depth-aware deblurring tractable. In this work, we propose to use a noisy/blurred/noisy image sequence and simultaneously recorded inertial measurements to jointly estimate scene depth and remove spatially-varying blur caused by depth variation and camera in-plane motion. The inertial data could provide initialization of camera motion parameters, while the noisy image pair preserve large-scale sharp edges from which a coarse disparity map can be generated. However, this initial estimate is not accurate enough to produce a high-quality clean image Therefore, we develop an optimization scheme to refine depth, motion parameters and latent image alternately. A Markov Random Field (MRF) framework is formulated to solve for the depth map by exploiting both stereo cues and motion blur cues and the residual Richardson-Lucy algorithm is used to effectively suppress deconvolution ringing artifacts. Experimental results demonstrate that our approach can address both depth estimation as well as image deblurring.
更多查看译文
关键词
Blur Kernel,Inertial Sensor,Disparity,Denoise,Deconvolution
AI 理解论文
溯源树
样例
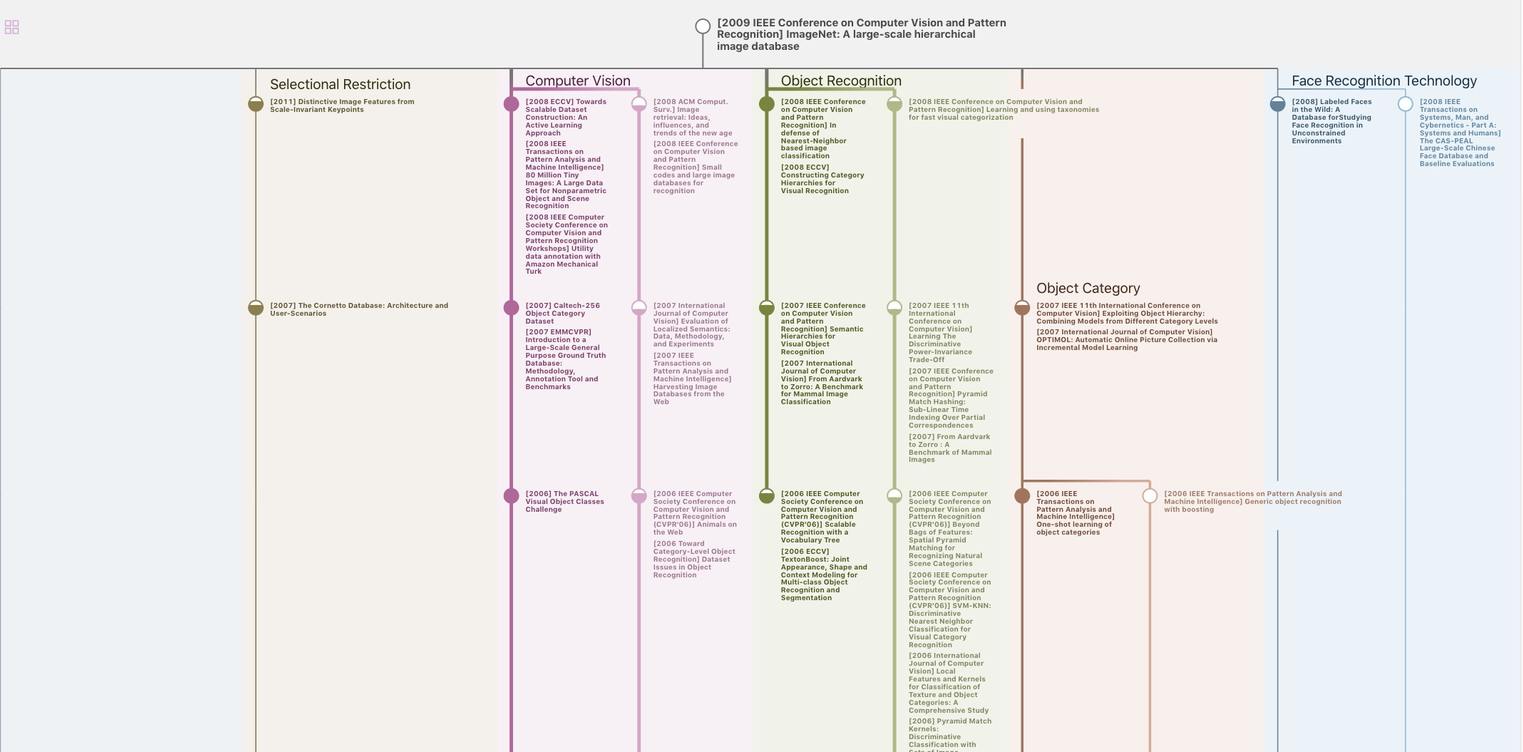
生成溯源树,研究论文发展脉络
Chat Paper
正在生成论文摘要