Relative Attribute Guided Dictionary Learning
2016 IEEE International Conference on Image Processing (ICIP)(2016)
摘要
A discriminative dictionary learning algorithm is proposed to find sparse signal representations using relative attributes as the available semantic information. In contrast, existing (discriminative) dictionary learning (DDL) approaches mostly utilize binary label information to enhance the discriminative property of the signal reconstruction residual, the sparse coding vectors or both. Compared to binary attributes or labels, relative attributes contain richer semantic information where the data is annotated with the attributes' strength. In this paper we use the relative attributes of training data indirectly to learn a discriminative dictionary. Precisely, we incorporate a rank function for the attributes in the dictionary learning process. In order to assess the quality of the obtained signals, we apply k-means clustering and measure the clustering performance. Experimental results conducted on three datasets, namely the PubFig [1], OSR [2] and Shoes [3] confirm that the proposed approach outperforms the state-of-the-art label based dictionary learning algorithms.
更多查看译文
关键词
Relative Attributes,Dictionary Learning,Clustering
AI 理解论文
溯源树
样例
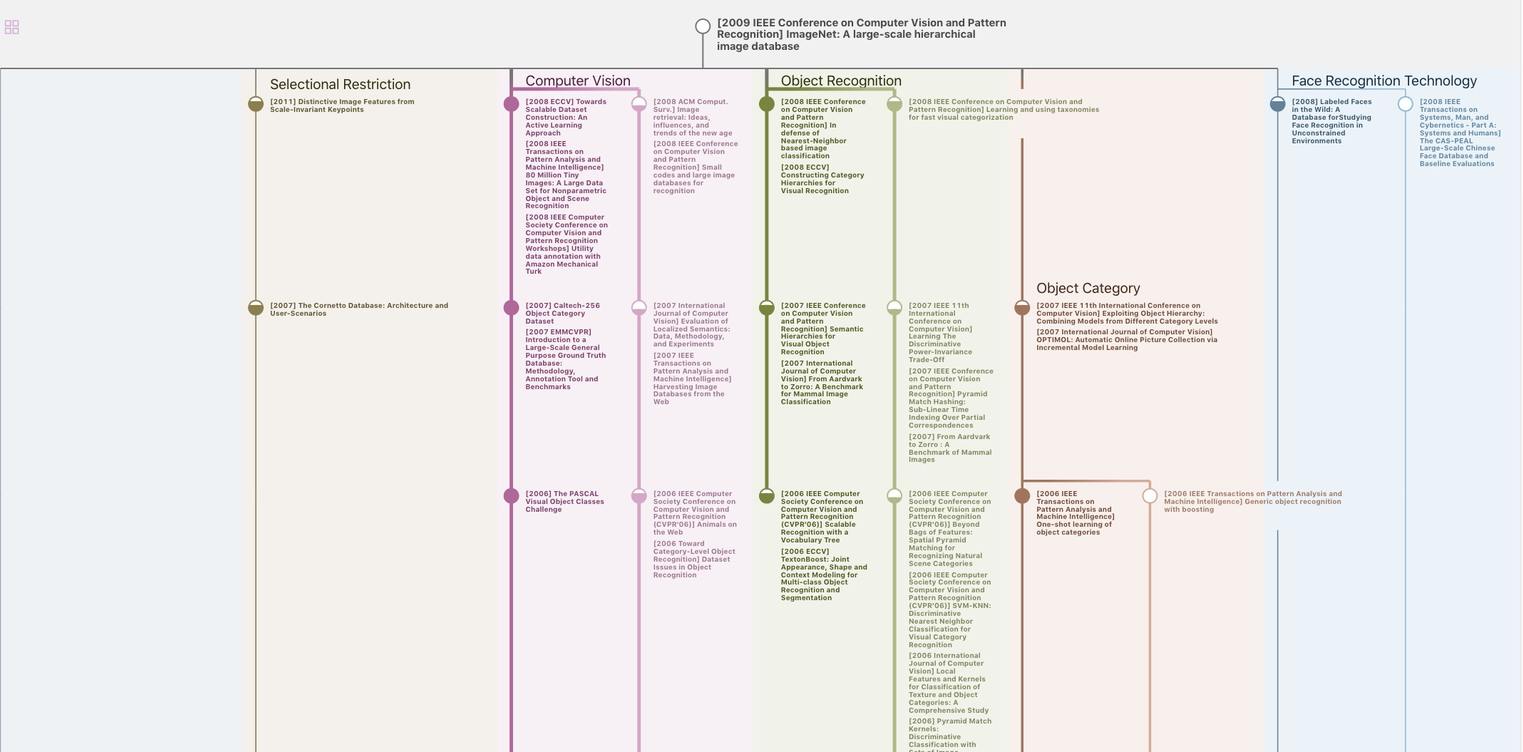
生成溯源树,研究论文发展脉络
Chat Paper
正在生成论文摘要