Towards Realizing Sign Language-to-Speech Conversion by Combining Deep Learning and Statistical Parametric Speech Synthesis.
ICYCSEE(2016)
摘要
This paper realizes a sign language-to-speech conversion system to solve the communication problem between healthy people and speech disorders. 30 kinds of different static sign languages are firstly recognized by combining the support vector machine (SVM) with a restricted Boltzmann machine (RBM) based regulation and a feedback fine-tuning of the deep model. The text of sign language is then obtained from the recognition results. A context-dependent label is generated from the recognized text of sign language by a text analyzer. Meanwhile, a hidden Markov model (HMM) based Mandarin-Tibetan bilingual speech synthesis system is developed by using speaker adaptive training. The Mandarin speech or Tibetan speech is then naturally synthesized by using context-dependent label generated from the recognized sign language. Tests show that the static sign language recognition rate of the designed system achieves 93.6 %. Subjective evaluation demonstrates that synthesized speech can get 4.0 of the mean opinion score (MOS).
更多查看译文
关键词
Deep learning, Support vector machine, Static sign language recognition, Context-dependent label, Hidden Markov model, Mandarin-Tibetan bilingual speech synthesis
AI 理解论文
溯源树
样例
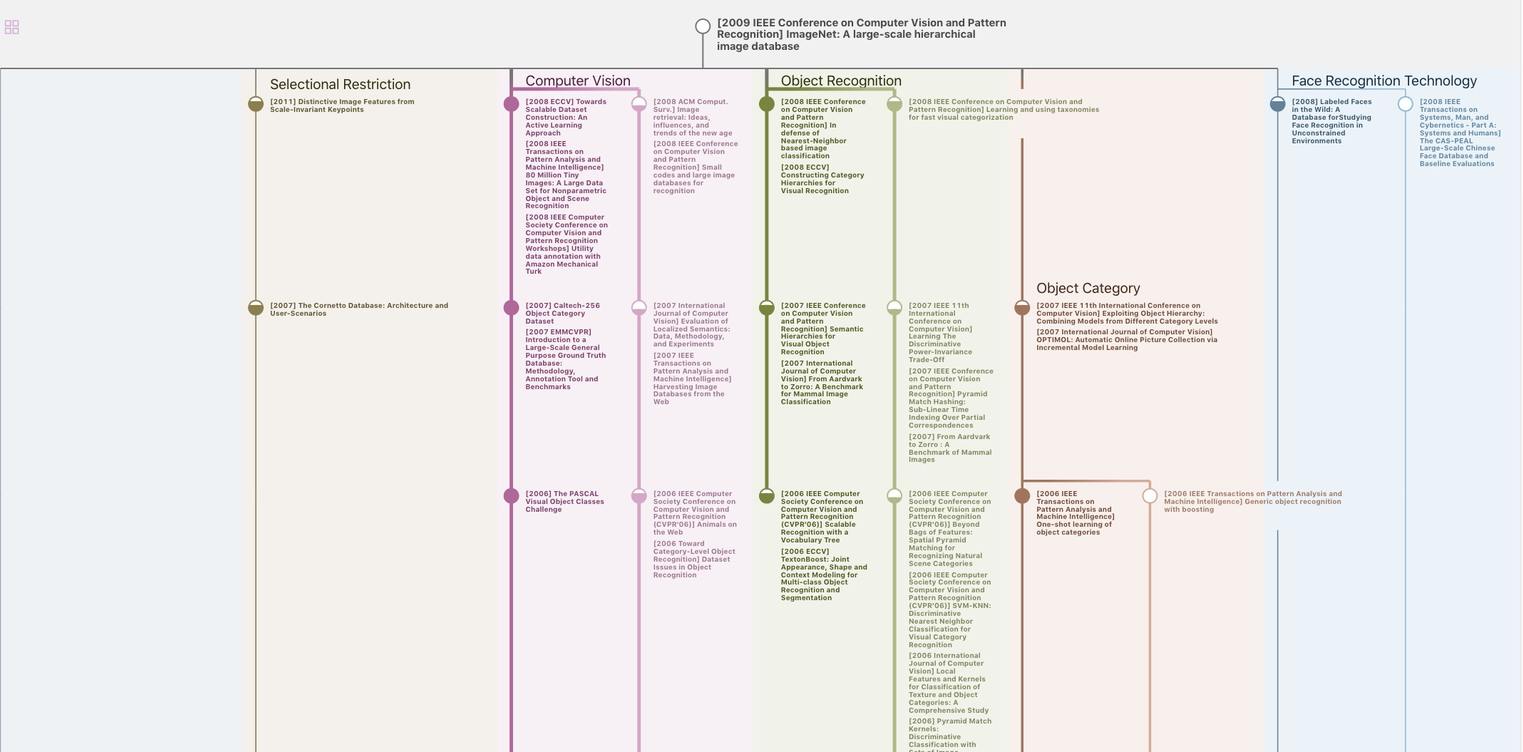
生成溯源树,研究论文发展脉络
Chat Paper
正在生成论文摘要