A Key Volume Mining Deep Framework For Action Recognition
2016 IEEE Conference on Computer Vision and Pattern Recognition (CVPR)(2016)
摘要
Recently, deep learning approaches have demonstrated remarkable progresses for action recognition in videos. Most existing deep frameworks equally treat every volume i.e. spatial-temporal video clip, and directly assign a video label to all volumes sampled from it. However, within a video, discriminative actions may occur sparsely in a few key volumes, and most other volumes are irrelevant to the labeled action category. Training with a large proportion of irrelevant volumes will hurt performance.To address this issue, we propose a key volume mining deep framework to identify key volumes and conduct classification simultaneously. Specifically, our framework is trained is optimized in an alternative way integrated to the forward and backward stages of Stochastic Gradient Descent (SGD). In the forward pass, our network mines key volumes for each action class. In the backward pass, it updates network parameters with the help of these mined key volumes. In addition, we propose "Stochastic out" to model key volumes from multi-modalities, and an effective yet simple "unsupervised key volume proposal" method for high quality volume sampling. Our experiments show that action recognition performance can be significantly improved by mining key volumes, and we achieve state-of-the-art per-formance on HMDB51 and UCF101 (93.1%).
更多查看译文
关键词
key volume mining deep framework,action recognition,deep learning,spatial-temporal video clip,video label,stochastic gradient descent,SGD,stochastic out method,unsupervised key volume proposal method,high quality volume sampling
AI 理解论文
溯源树
样例
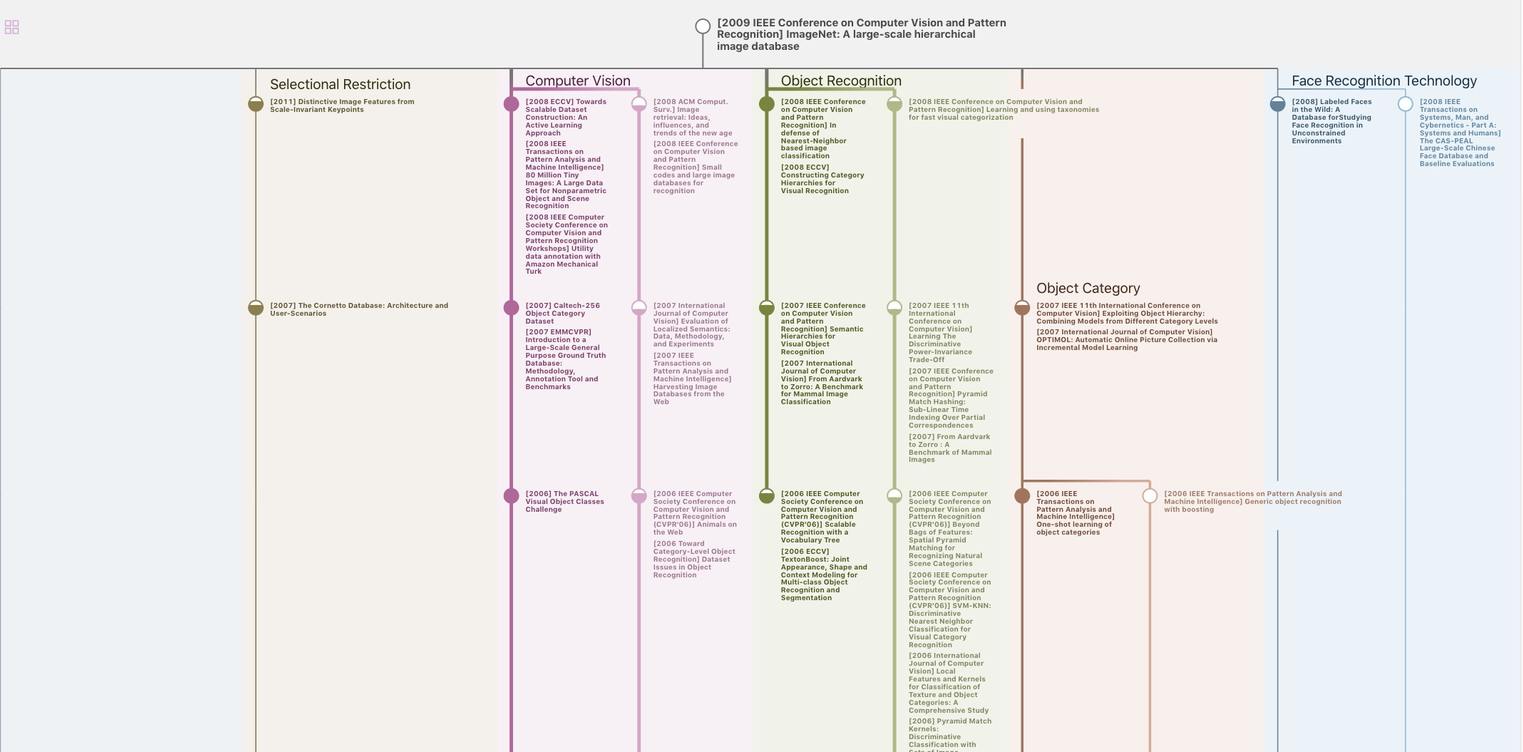
生成溯源树,研究论文发展脉络
Chat Paper
正在生成论文摘要