Robust Visual Tracking with Deep Convolutional Neural Network Based Object Proposals on PETS
IEEE Computer Society Conference on Computer Vision and Pattern Recognition Workshops(2016)
摘要
Tracking by detection based object tracking methods encounter numerous complications including object appearance changes, size and shape deformations, partial and full occlusions, which make online adaptation of classifiers and object models a substantial challenge. In this paper, we employ an object proposal network that generates a small yet refined set of bounding box candidates to mitigate the this object model refitting problem by concentrating on hard negatives when we update the classifier. This helps improving the discriminative power as hard negatives are likely to be due to background and other distractions. Another intuition is that, in each frame, applying the classifier only on the refined set of object-like candidates would be sufficient to eliminate most of the false positives. Incorporating an object proposal makes the tracker robust against shape deformations since they are handled naturally by the proposal stage. We demonstrate evaluations on the PETS 2016 dataset and compare with the state-of-the-art trackers. Our method provides the superior results.
更多查看译文
关键词
robust visual tracking,deep convolutional neural network based object proposals,PETS,detection based object tracking,object appearance,object shape deformations,object size,occlusions,online adaptation,classifiers,bounding box candidates,object model refitting,discriminative power,hard negatives,object-like candidates,false positives
AI 理解论文
溯源树
样例
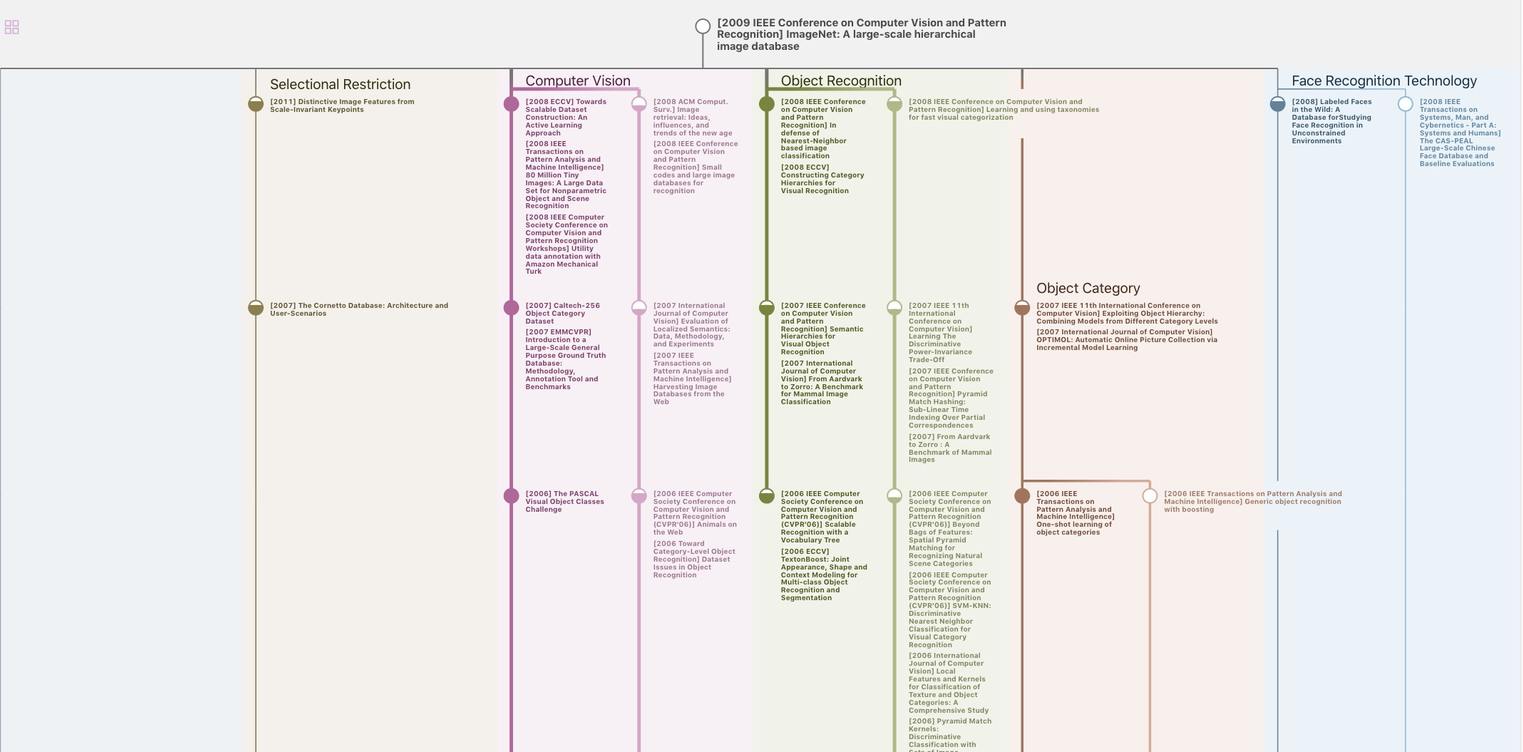
生成溯源树,研究论文发展脉络
Chat Paper
正在生成论文摘要