Effective Reward Function In Discernment Behavior Reinforcement Learning Based On Categorization Progress
2016 IEEE-RAS 16TH INTERNATIONAL CONFERENCE ON HUMANOID ROBOTS (HUMANOIDS)(2016)
摘要
In object categorization tasks, the behavior trough that a robot observes an object is important. In order to categorize an object well, multiple observations through multiple behaviors are required in many cases. In this paper, we propose a class of methods, Discernment Behavior Reinforcement Learning with Adaptive Reward, which allows a robot to learn the multiple behaviors. In DBRL-AR, the observation results in previously performed behaviors/observations are took into account in the latter behaviors/observations. The effectiveness of the proposed algorithms was validated using a humanoid robot under the criteria of Between-Class Non-coincidence (BCN) and Within-Class Coincidence (WCC) that were calculated from actual data that the humanoid robot had been observed through learned behaviors. In the experiment, DBRL-AR improved the values of BCN and WCC that were small in the first observation by the latter observation. This means that the robot was able to perform the behaviors that effectively standing out the features that was not observed in the first observation in the latter observation.
更多查看译文
关键词
reward function,discernment behavior reinforcement learning,categorization progress,object categorization,behavior trough,adaptive reward,robot learning,DBRL-AR,humanoid robot,between-class noncoincidence,BCN,within-class coincidence,WCC
AI 理解论文
溯源树
样例
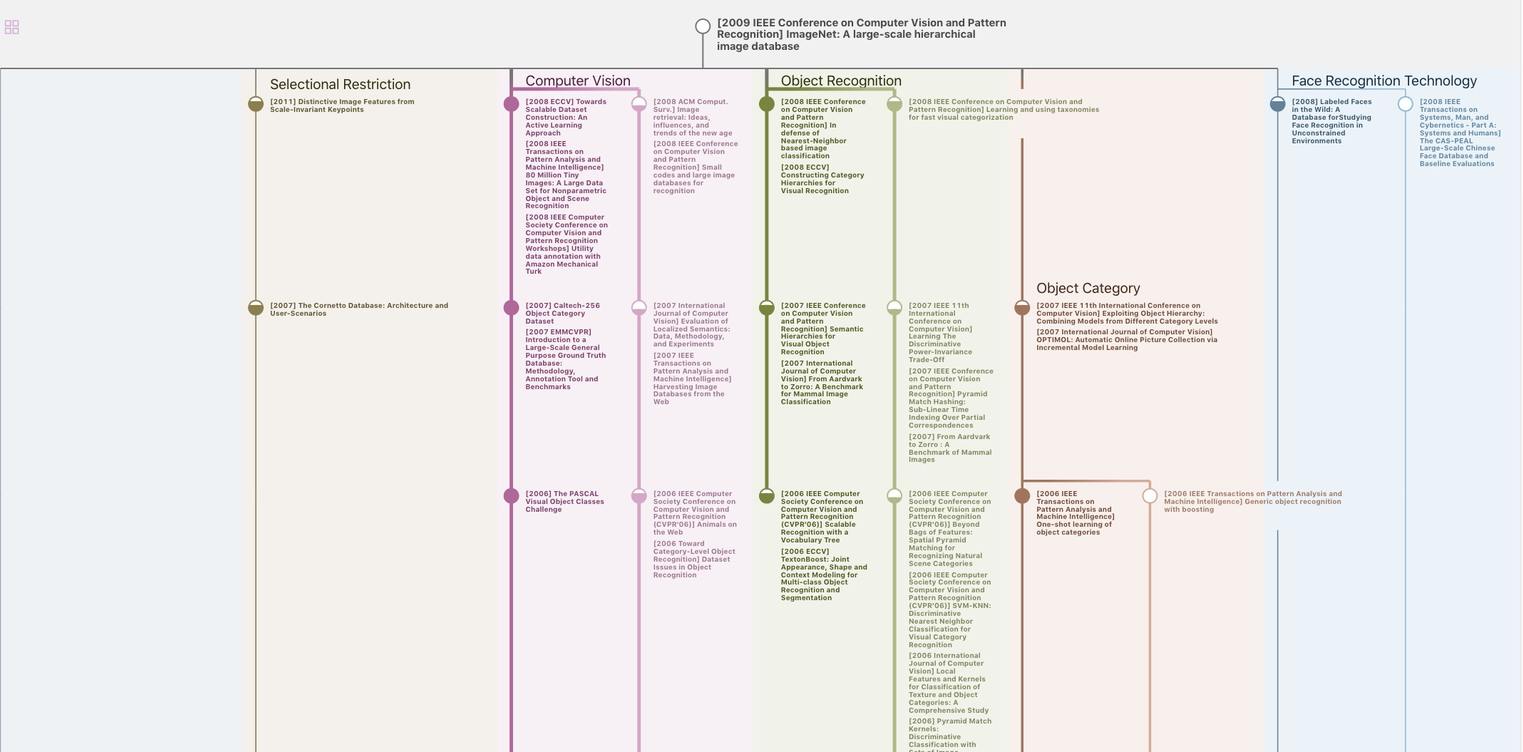
生成溯源树,研究论文发展脉络
Chat Paper
正在生成论文摘要