Rbf Neural Network Combined With Self-Adaptive Mode And Genetic Algorithm To Identify Velocity Profile Of Swimmers
2016 IEEE Symposium Series on Computational Intelligence (SSCI)(2016)
摘要
On the side of enhancing the execution of skills, specialists in sports are adopting analysis of kinematics to correct actions of an athlete. By means of technological resources used to measure physical variables and to supply relevant data to trainers, results related to improvements on athletes' performance are being achieved. In this context, this work uses the Radial Basis Function Neural Networks (RBF-NNs) combined in cascade with a Genetic Algorithm (GA) as a cross-correlation method, likewise Multiobjective Differential Evolution (MODE) and self-adaptive MODE (using JADE - Adaptive Differential Evolution with Optional External Archive - self-adaptation method) for optimization. The RBF-NNs have been applied to predict the swimmers velocity profile being multiple correlation coefficient (R-2) adopted to evaluate the optimization techniques during both estimation and validation stages. A data acquisition system was used to capture the para-swimming athletes' instantaneous velocity data, swimming 25 meters in crawl, breaststroke, backstroke, and butterfly strokes. Looking at the results achieved, self-adaptive MODE outperforms classical MODE in approach point of view considering all cases studied, finding the best RBFNN framework to identify the profile of speed in swimming.
更多查看译文
关键词
Multiobjective optimization,RBF neural networks,self-adaptive,swim,time series forecasting
AI 理解论文
溯源树
样例
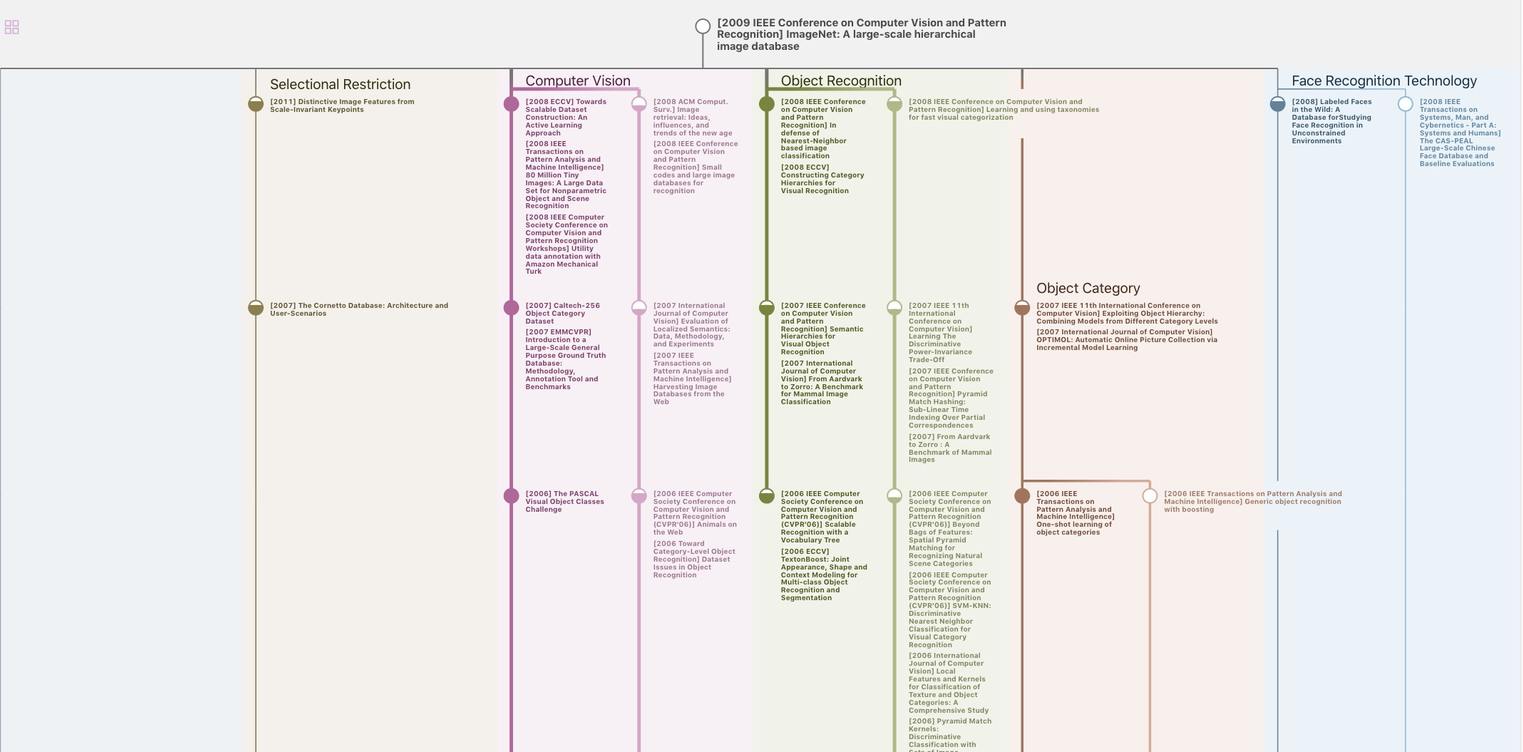
生成溯源树,研究论文发展脉络
Chat Paper
正在生成论文摘要