Score Look-Alike Audiences
2016 IEEE 16TH INTERNATIONAL CONFERENCE ON DATA MINING WORKSHOPS (ICDMW)(2016)
Abstract
Look-alike models, which are efficient tools for finding similar users from a smaller user set, are quickly revolutionizing the online programmatic advertising industry. The datasets in these contexts exhibit extremely sparse feature spaces on a massive scale, so traditionally, the state-of-the-art look-alike models have used pairwise similarities to construct these similar user sets. One of the key challenges of the similarity-based models is that they do not provide a way to measure the potential value of the users to an advertiser, which is crucial in an advertising context. We propose methods to score users within the expanded audience in a way which relates directly to the business metric that the advertiser wants to optimize. We present three scoring models and show that, through empirical evaluation using real-world, large-scale data, by incorporating the potential value of a user to an advertiser into our scoring model, we can significantly improve the performance of the look-alike models over methods which only use pairwise similarities of users.
MoreTranslated text
Key words
look-alike model,user scoring,user ranking,online advertising,spark
AI Read Science
Must-Reading Tree
Example
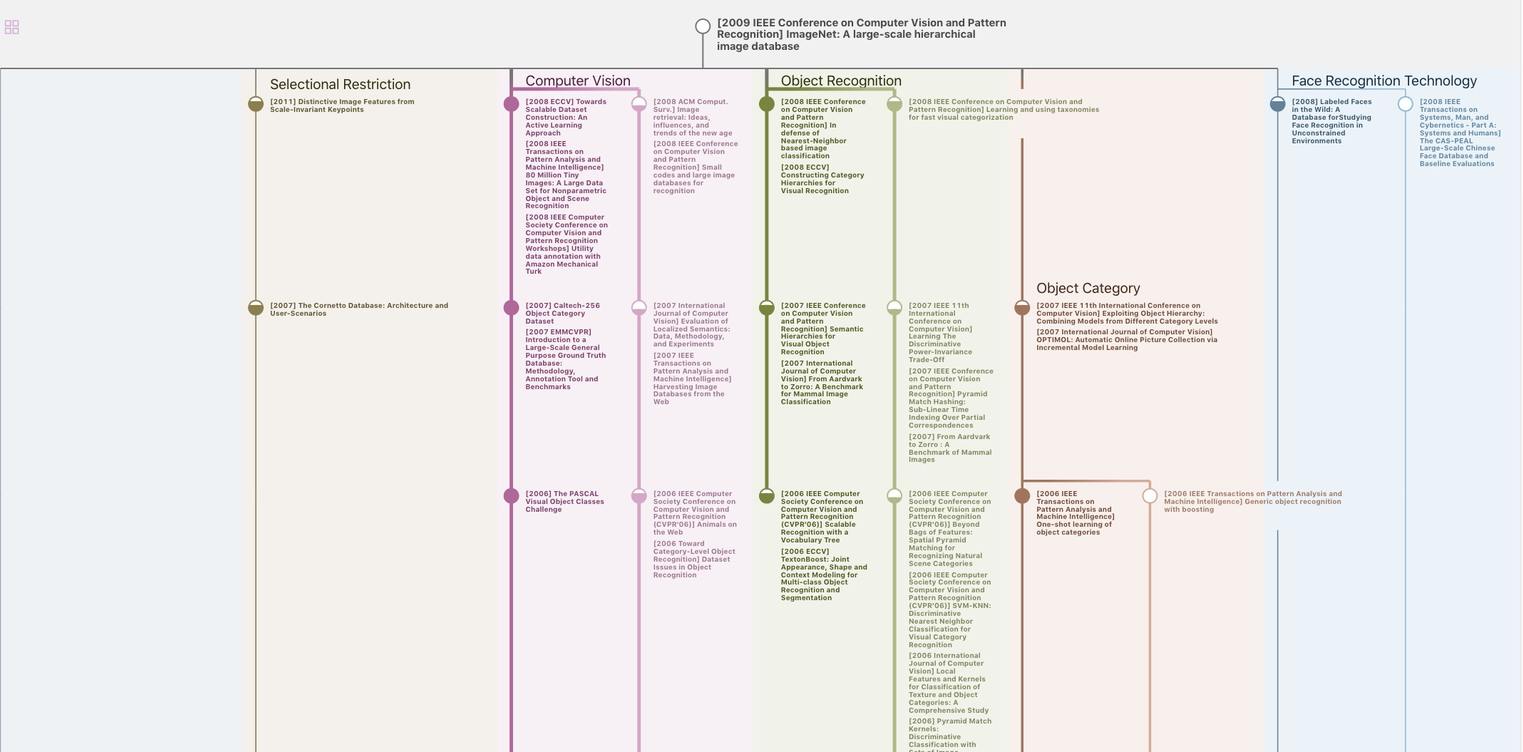
Generate MRT to find the research sequence of this paper
Chat Paper
Summary is being generated by the instructions you defined