Improved Multilingual Training of Stacked Neural Network Acoustic Models for Low Resource Languages
17TH ANNUAL CONFERENCE OF THE INTERNATIONAL SPEECH COMMUNICATION ASSOCIATION (INTERSPEECH 2016), VOLS 1-5: UNDERSTANDING SPEECH PROCESSING IN HUMANS AND MACHINES(2016)
摘要
This paper proposes several improvements to multilingual training of neural network acoustic models for speech recognition and keyword spotting in the context of low-resource languages. We concentrate on the stacked architecture where the first network is used as a bottleneck feature extractor and the second network as the acoustic model. We propose to improve multilingual training when the amount of data from different languages is very different by applying balancing scalers to the training examples. We also explore how to exploit multilingual data to train the second neural network of the stacked architecture. An ensemble training method that can take advantage of both unsupervised pretraining as well as multilingual training is found to give the best speech recognition performance across a wide variety of languages, while system combination of differently trained multilingual Models results in further improvements in keyword search performance.
更多查看译文
关键词
speech recognition, keyword spotting, multilingual training, deep learning, system combination
AI 理解论文
溯源树
样例
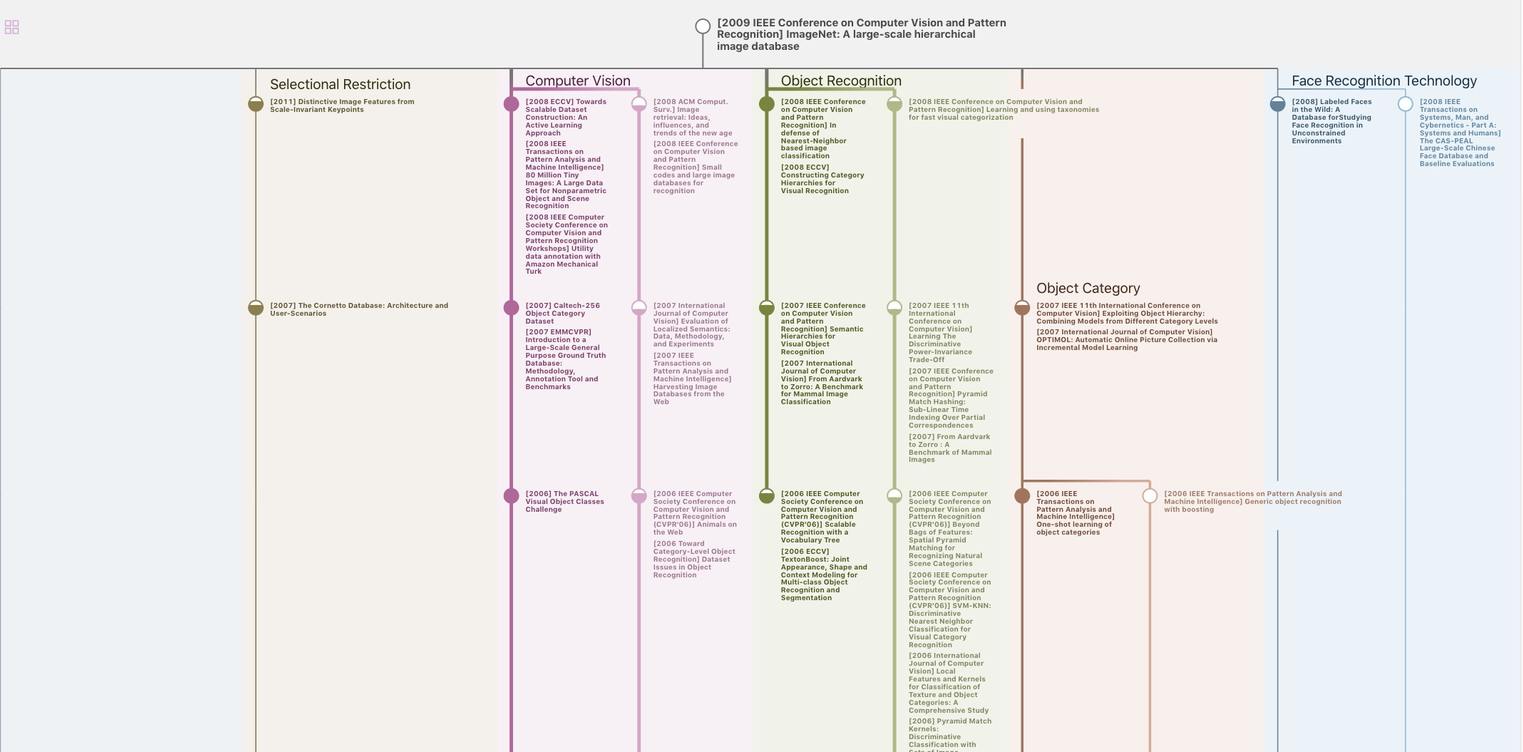
生成溯源树,研究论文发展脉络
Chat Paper
正在生成论文摘要