Blind Non-Intrusive Speech Intelligibility Prediction using Twin-HMMs
17TH ANNUAL CONFERENCE OF THE INTERNATIONAL SPEECH COMMUNICATION ASSOCIATION (INTERSPEECH 2016), VOLS 1-5: UNDERSTANDING SPEECH PROCESSING IN HUMANS AND MACHINES(2016)
摘要
Automatic prediction of speech intelligibility is highly desirable in the speech research community, since listening tests are time-consuming and can not be used online. Most of the available objective speech intelligibility measures are intrusive methods, as they require a clean reference signal in addition to the corresponding noisy/processed signal at hand. In order to overcome the problem of predicting the speech intelligibility in the absence of the clean reference signal, we have proposed in [1] to employ a recognition/synthesis framework called twin hidden Markov model (THMM) for synthesizing the clean features, required inside an intrusive intelligibility prediction method. The new framework can predict the speech intelligibility equally well as well-known intrusive methods like the short-time objective intelligibility (STOI). The original THMM, however, requires the correct transcription for synthesizing the clean reference features, which is not always available. In this paper, we go one step further and investigate the use of the recognized transcription instead of the oracle transcription for obtaining a more widely applicable speech intelligibility prediction. We show that the output of the newly-proposed blind approach is highly correlated with the human speech recognition results, collected via crowdsourcing in different noise conditions.
更多查看译文
关键词
Speech intelligibility prediction, twin-HMM, speech recognition, speech synthesis, non-intrusive methods, objective measures
AI 理解论文
溯源树
样例
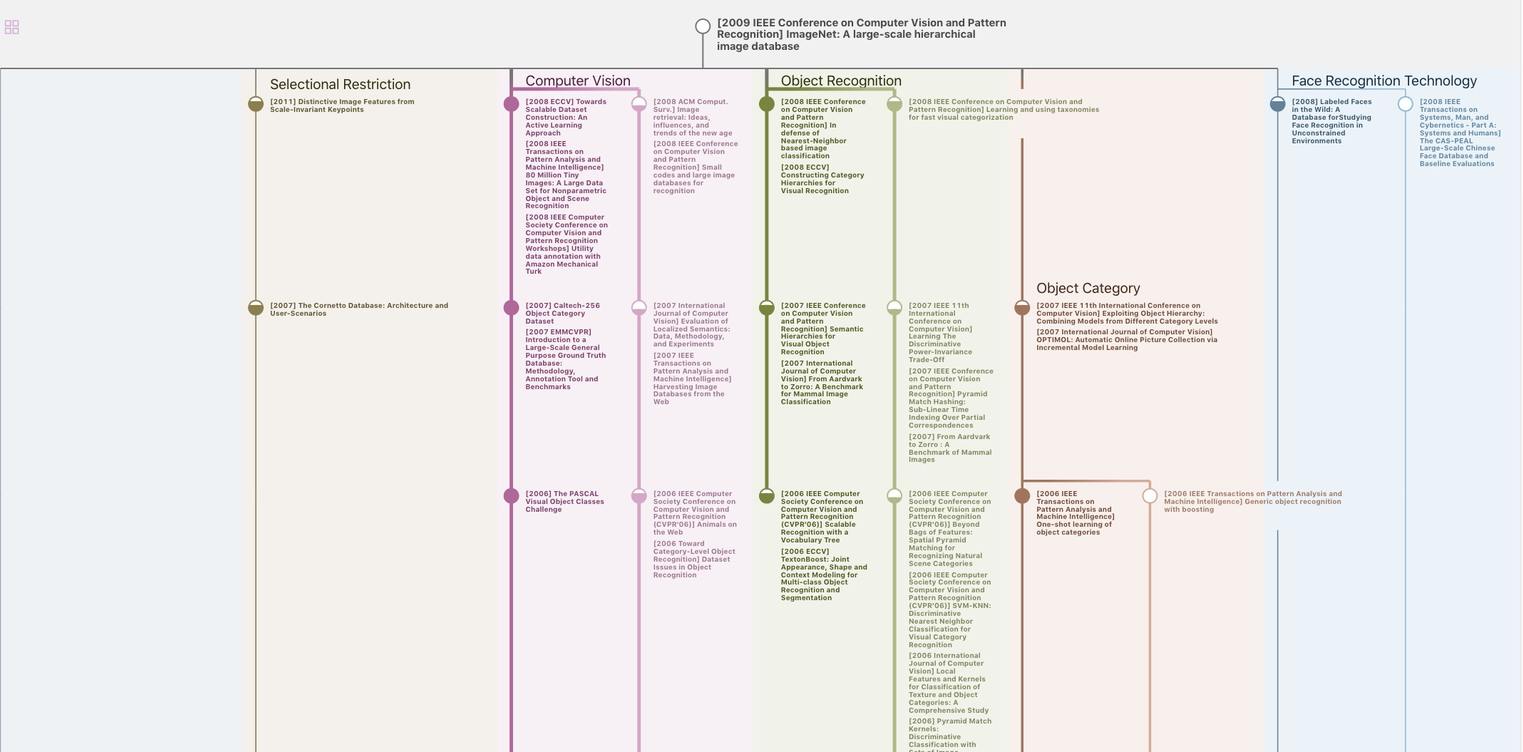
生成溯源树,研究论文发展脉络
Chat Paper
正在生成论文摘要