Discrete Multi-agent Plan Recognition: Recognizing Teams, Goals, and Plans from Action Sequences.
AGENTS AND ARTIFICIAL INTELLIGENCE, ICAART 2016(2017)
摘要
Multi-agent Plan Recognition (MPAR) infers teams and their goals from observed actions of individual agents. The complexity of creating a priori plan libraries significantly increases to account for diversity of action sequences different team structures may exhibit. A key challenge in MPAR is effectively pruning the joint search space of agent to team compositions and goal to team assignments. Here, we describe discrete Multi-agent Plan Recognition as Planning (MAPRAP), which extends Ramirez and Geffner's Plan Recognition as Planning (PRAP) approach to multi-agent domains. Instead of a plan library, MAPRAP uses the planning domain and synthesizes plans to achieve hypothesized goals with additional constraints for suspected team composition and previous observations. By comparing costs of plans, MAPRAP identifies feasible interpretations that explain the teams and plans observed. We establish a performance profile for discrete MAPRAP in a multi-agent blocks-world domain. We evaluated precision, accuracy, and recall after each observation. We compare two pruning strategies to dampen the explosion of hypotheses tested. Aggressive pruning averages 1.05 plans synthesized per goal per time step for multi-agent scenarios vice 0.56 for single agent scenarios.
更多查看译文
关键词
Multi-agent systems,Plan recognition
AI 理解论文
溯源树
样例
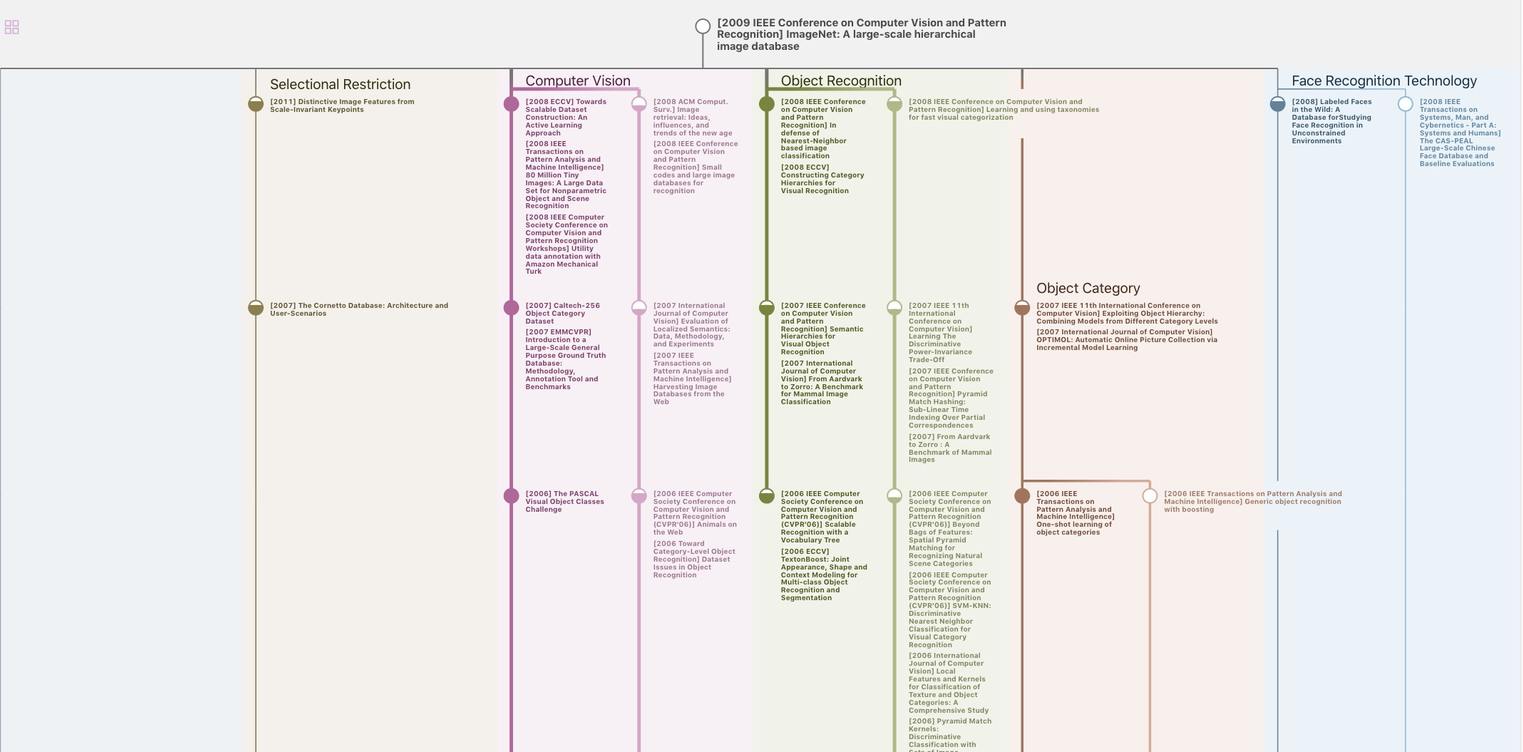
生成溯源树,研究论文发展脉络
Chat Paper
正在生成论文摘要