An efficient multi-objective PSO algorithm assisted by Kriging metamodel for expensive black-box problems
Journal of Global Optimization(2016)
摘要
The huge computational overhead is the main challenge in the application of community based optimization methods, such as multi-objective particle swarm optimization and multi-objective genetic algorithm, to deal with the multi-objective optimization involving costly simulations. This paper proposes a Kriging metamodel assisted multi-objective particle swarm optimization method to solve this kind of expensively black-box multi-objective optimization problems. On the basis of crowding distance based multi-objective particle swarm optimization algorithm, the new proposed method constructs Kriging metamodel for each expensive objective function adaptively, and then the non-dominated solutions of the metamodels are utilized to guide the update of particle population. To reduce the computational cost, the generalized expected improvements of each particle predicted by metamodels are presented to determine which particles need to perform actual function evaluations. The suggested method is tested on 12 benchmark functions and compared with the original crowding distance based multi-objective particle swarm optimization algorithm and non-dominated sorting genetic algorithm-II algorithm. The test results show that the application of Kriging metamodel improves the search ability and reduces the number of evaluations. Additionally, the new proposed method is applied to the optimal design of a cycloid gear pump and achieves desirable results.
更多查看译文
关键词
Multi-objective optimization,Kriging metamodel,Particle swarm optimization,Black-box function
AI 理解论文
溯源树
样例
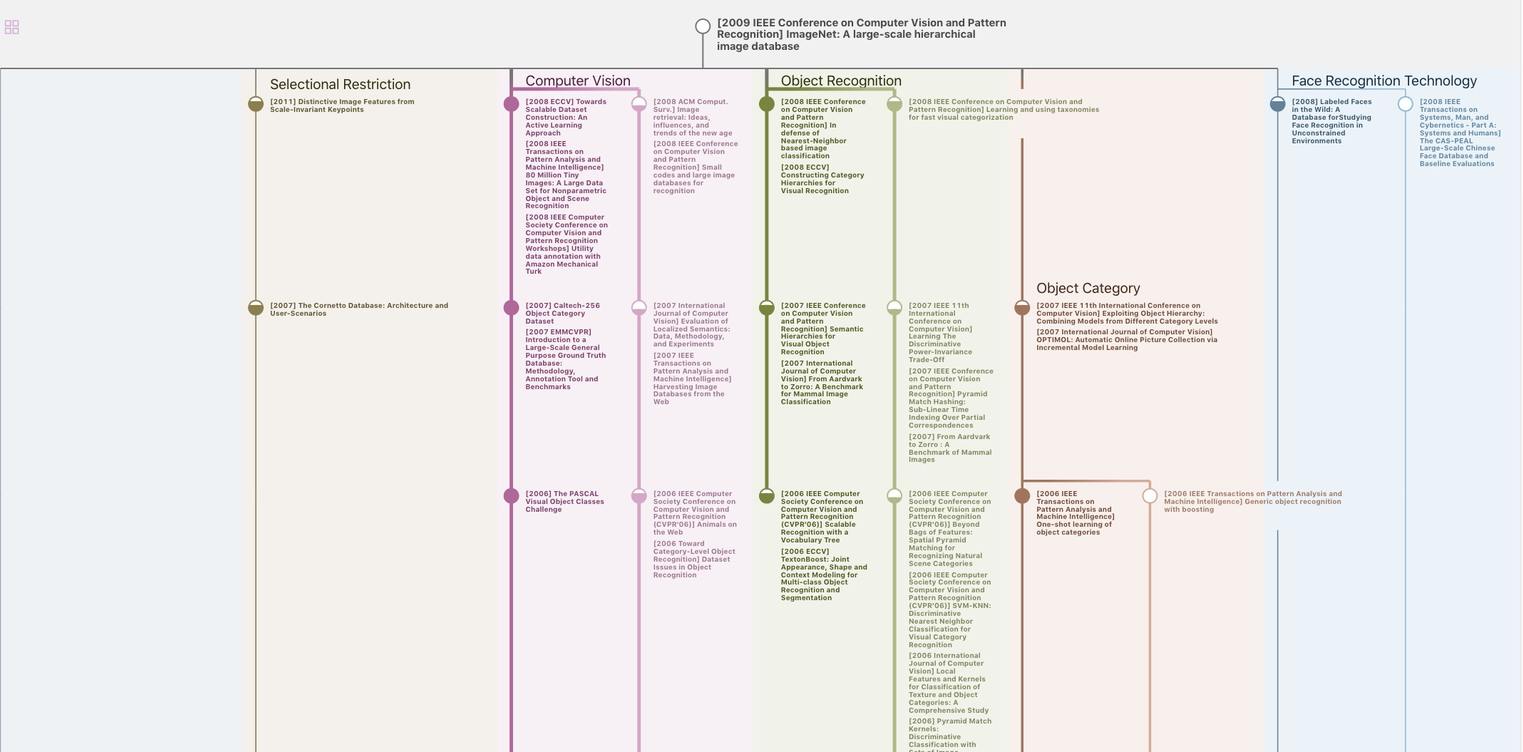
生成溯源树,研究论文发展脉络
Chat Paper
正在生成论文摘要