Towards faster convergence of evolutionary multi-criterion optimization algorithms using Karush Kuhn Tucker optimality based local search.
Computers & OR(2017)
摘要
Evolutionary multi-criterion optimization (EMO) algorithms emphasize non-dominated and less crowded solutions in a population iteratively until the population converges close to the Pareto optimal set. During the search process, non-dominated solutions are differentiated only by their local crowding or contribution to hypervolume or using a similar other metric. Thus, during evolution and even at the final iteration, the true convergence behavior of each non-dominated solutions from the Pareto optimal set is unknown. Recent studies have used Karush Kuhn Tucker (KKT) optimality conditions to develop a KKT Proximity Measure (KKTPM) for estimating proximity of a solution from Pareto optimal set for a multi-objective optimization problem. In this paper, we integrate KKTPM with a recently proposed EMO algorithm to enhance its convergence properties towards the true Pareto optimal front. Specifically, we use KKTPM to identify poorly converged non-dominated solutions in every generation and apply an achievement scalarizing function based local search procedure to improve their convergence. Assisted by the KKTPM, the modified algorithm is designed in a way that maintains the total number of function evaluations as low as possible while making use of local search where it is most needed. Simulations on both constrained and unconstrained multi- and many objectives optimization problems demonstrate that the hybrid algorithm significantly improves the overall convergence properties. This study brings evolutionary optimization closer to mainstream optimization field and should motivate researchers to utilize KKTPM measure further within EMO and other numerical optimization algorithms. Graphical abstractDisplay Omitted HighlightsUtilization of the recently proposed KKTPM to identify poorly converged solutions in multiobjective optimization problems.Scalarizing the multiobjective problem using an Augmented Scalarization Function (ASF), and applying local search on the identified points to enhance overall convergence.Proposing VarDens, a new bi-objective optimization problem with arbitrary number of variables. VarDens represents a category of problems where the Pareto front is disjoint and some sections are easier to attain that others.Weaving KKTPM, NSGA-III and local search into one algorithm that outperforms the original NSGA-III in terms of convergence.
更多查看译文
关键词
NSGA-III,Local,Search,KKT,EMO
AI 理解论文
溯源树
样例
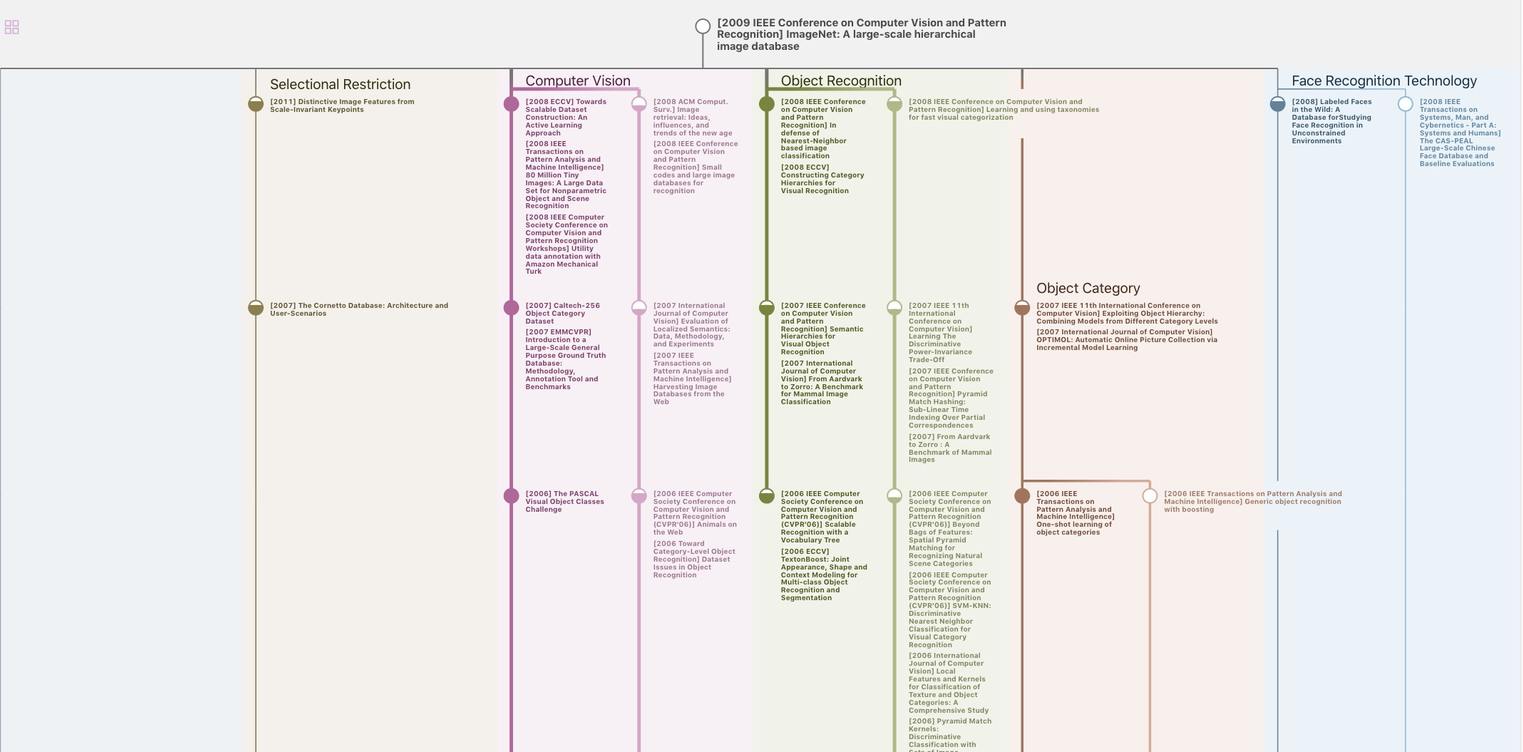
生成溯源树,研究论文发展脉络
Chat Paper
正在生成论文摘要