Deep Motion Features for Visual Tracking
2016 23rd International Conference on Pattern Recognition (ICPR)(2016)
摘要
Robust visual tracking is a challenging computer vision problem, with many real-world applications. Most existing approaches employ hand-crafted appearance features, such as HOG or Color Names. Recently, deep RGB features extracted from convolutional neural networks have been successfully applied for tracking. Despite their success, these features only capture appearance information. On the other hand, motion cues provide discriminative and complementary information that can improve tracking performance. Contrary to visual tracking, deep motion features have been successfully applied for action recognition and video classification tasks. Typically, the motion features are learned by training a CNN on optical flow images extracted from large amounts of labeled videos. This paper presents an investigation of the impact of deep motion features in a tracking-by-detection framework. We further show that hand-crafted, deep RGB, and deep motion features contain complementary information. To the best of our knowledge, we are the first to propose fusing appearance information with deep motion features for visual tracking. Comprehensive experiments clearly suggest that our fusion approach with deep motion features outperforms standard methods relying on appearance information alone.
更多查看译文
关键词
deep motion features,robust visual tracking,computer vision problem,deep RGB feature extraction,convolutional neural networks,action recognition,video classification,optical flow,tracking-by-detection framework
AI 理解论文
溯源树
样例
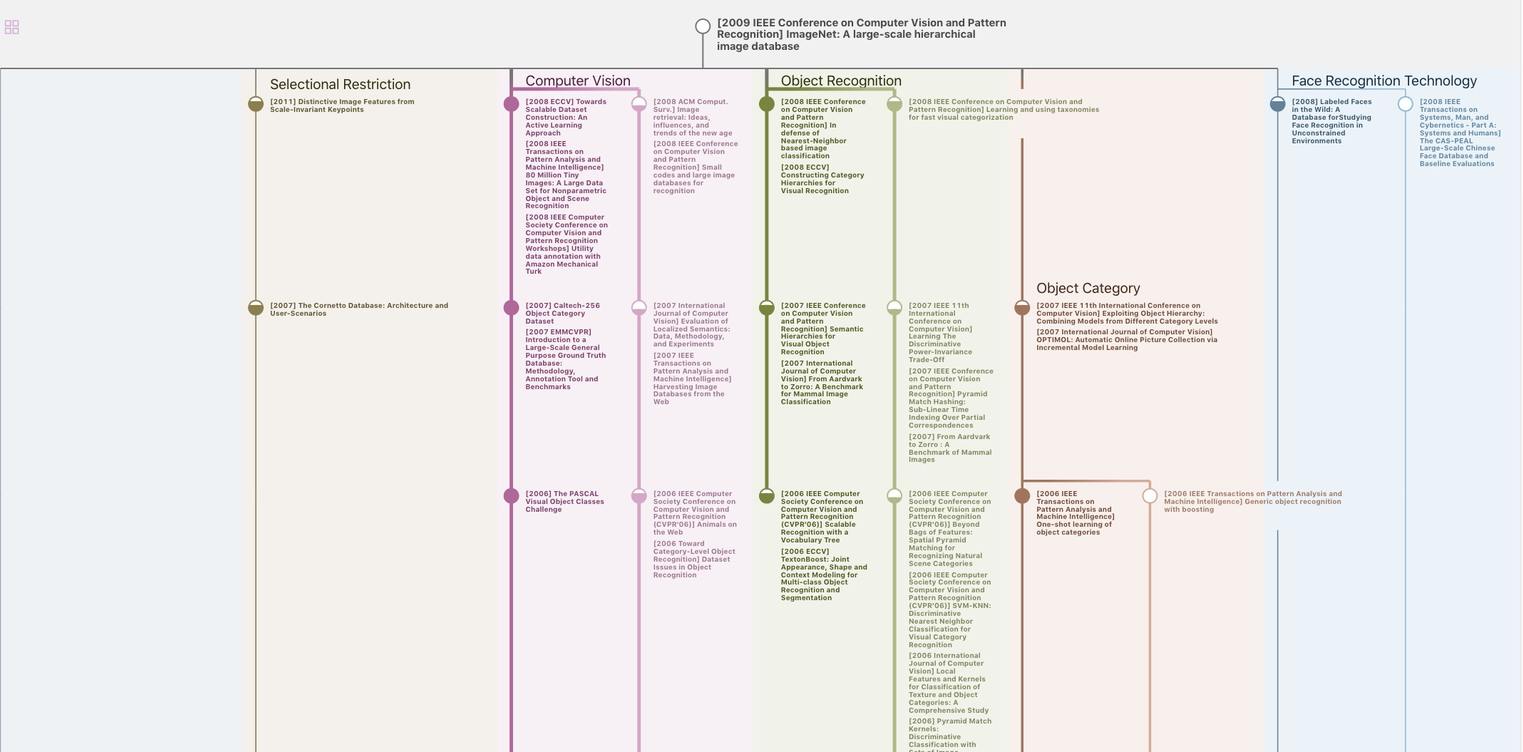
生成溯源树,研究论文发展脉络
Chat Paper
正在生成论文摘要