Unsupervised Learning of Sentence Representations using Convolutional Neural Networks.
arXiv: Computation and Language(2016)
摘要
We propose a new encoder-decoder approach to learn distributed sentence representations from unlabeled sentences. The word-to-vector representation is used, and convolutional neural networks are employed as sentence encoders, mapping an input sentence into a fixed-length vector. This representation is decoded using long short-term memory recurrent neural networks, considering several tasks, such as reconstructing the input sentence, or predicting the future sentence. We further describe a hierarchical encoder-decoder model to encode a sentence to predict multiple future sentences. By training our models on a large collection of novels, we obtain a highly generic convolutional sentence encoder that performs well in practice. Experimental results on several benchmark datasets, and across a broad range of applications, demonstrate the superiority of the proposed model over competing methods.
更多查看译文
AI 理解论文
溯源树
样例
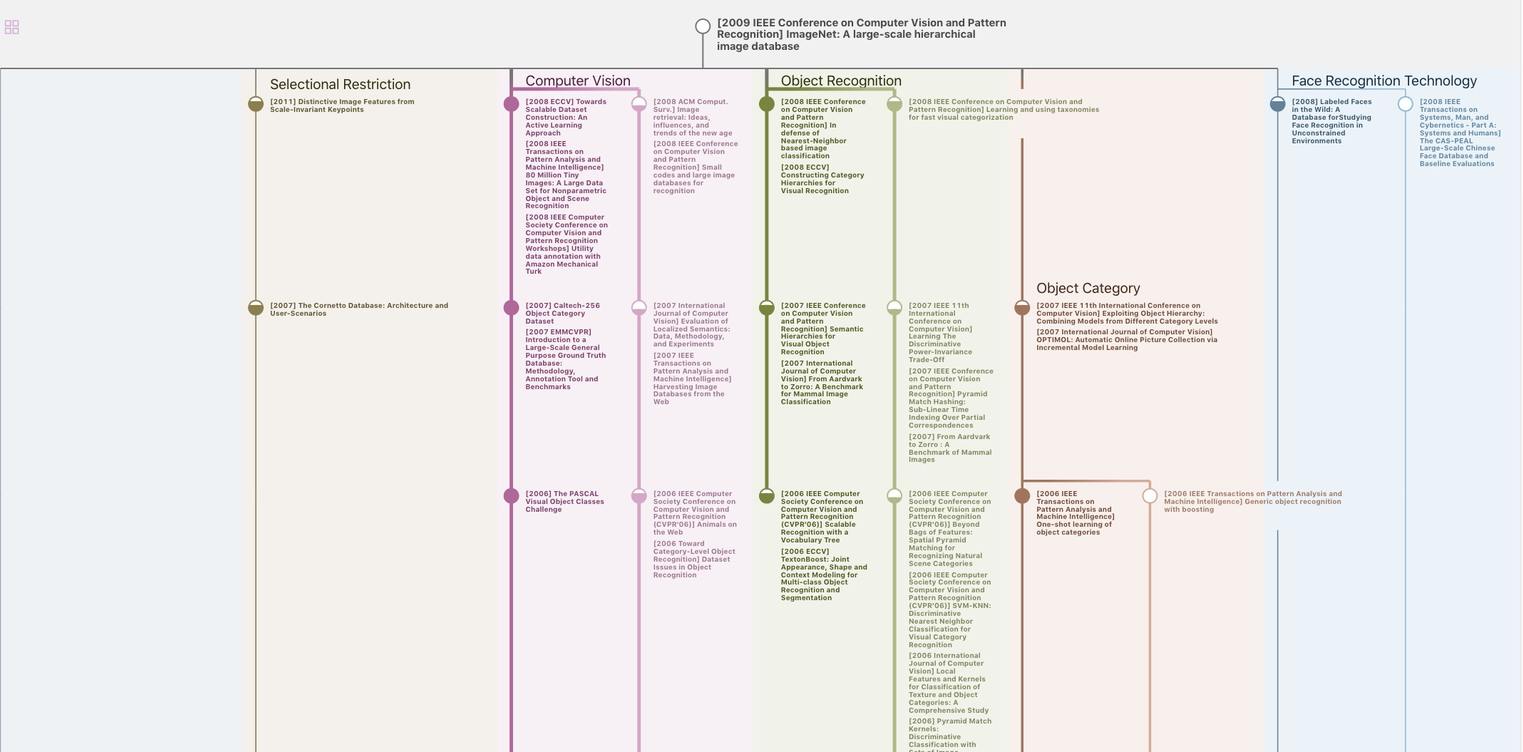
生成溯源树,研究论文发展脉络
Chat Paper
正在生成论文摘要