Semantic Video Segmentation by Gated Recurrent Flow Propagation
arXiv (Cornell University)(2017)
摘要
Semantic video segmentation is challenging due to the sheer amount of data that needs to be processed and labeled in order to construct accurate models. In this paper we present a deep, end-to-end trainable methodology to video segmentation that is capable of leveraging information present in unlabeled data in order to improve semantic estimates. Our model combines a convolutional architecture and a spatio-temporal transformer recurrent layer that are able to temporally propagate labeling information by means of optical flow, adaptively gated based on its locally estimated uncertainty. The flow, the recognition and the gated temporal propagation modules can be trained jointly, end-to-end. The temporal, gated recurrent flow propagation component of our model can be plugged into any static semantic segmentation architecture and turn it into a weakly supervised video processing one. Our extensive experiments in the challenging CityScapes and Camvid datasets, and based on multiple deep architectures, indicate that the resulting model can leverage unlabeled temporal frames, next to a labeled one, in order to improve both the video segmentation accuracy and the consistency of its temporal labeling, at no additional annotation cost and with little extra computation.
更多查看译文
关键词
video segmentation,convolutional architecture,gated temporal propagation,gated recurrent flow propagation,multiple deep architectures,weakly supervised video processing,static semantic segmentation architecture,optical flow,spatiotemporal transformer recurrent layer,semantic video segmentation
AI 理解论文
溯源树
样例
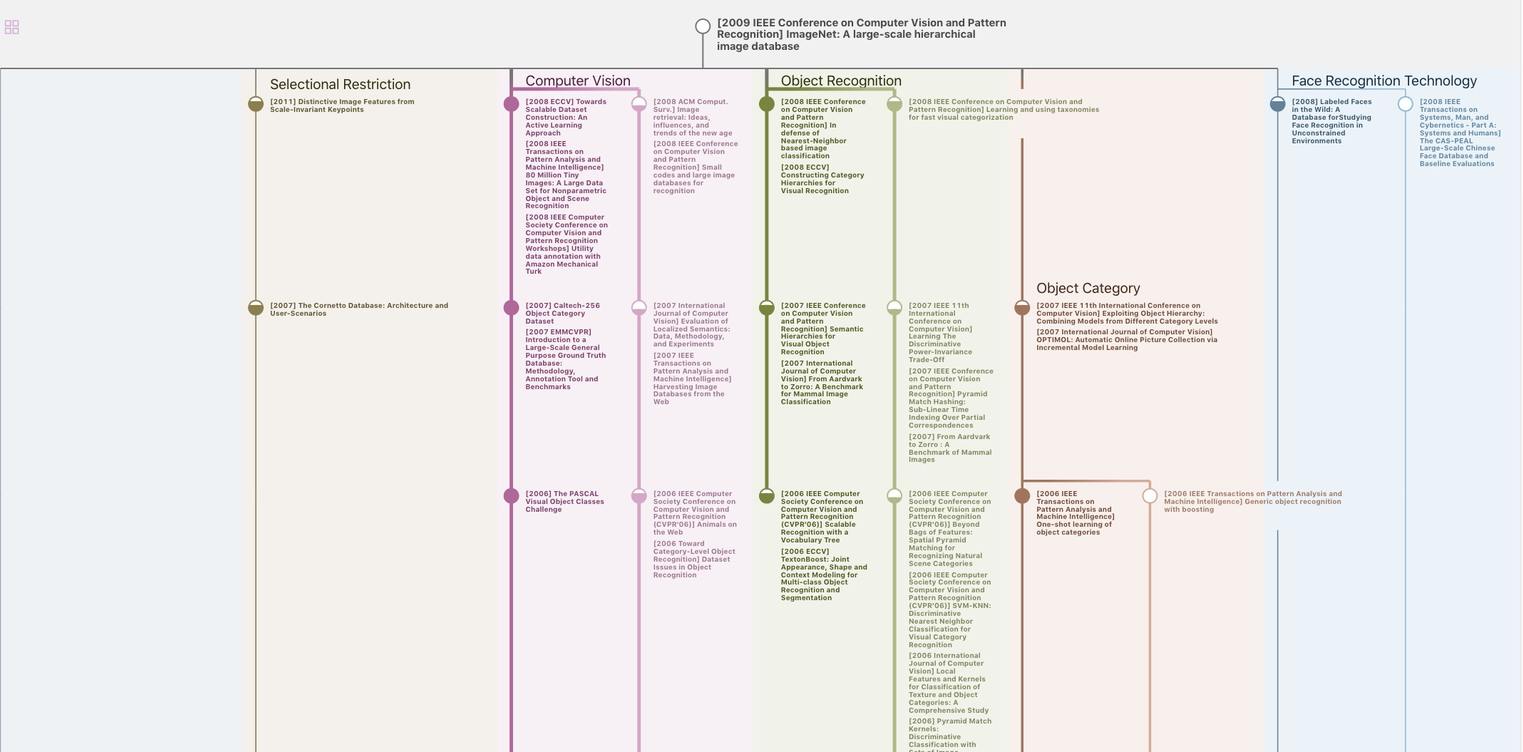
生成溯源树,研究论文发展脉络
Chat Paper
正在生成论文摘要