Image features for visual teach-and-repeat navigation in changing environments.
Robotics and Autonomous Systems(2017)
摘要
We present an evaluation of standard image features in the context of long-term visual teach-and-repeat navigation of mobile robots, where the environment exhibits significant changes in appearance caused by seasonal weather variations and daily illumination changes. We argue that for long-term autonomous navigation, the viewpoint-, scale- and rotation- invariance of the standard feature extractors is less important than their robustness to the mid- and long-term environment appearance changes. Therefore, we focus our evaluation on the robustness of image registration to variable lighting and naturally-occurring seasonal changes. We combine detection and description components of different image extractors and evaluate their performance on five datasets collected by mobile vehicles in three different outdoor environments over the course of one year. Moreover, we propose a trainable feature descriptor based on a combination of evolutionary algorithms and Binary Robust Independent Elementary Features, which we call GRIEF (Generated BRIEF). In terms of robustness to seasonal changes, the most promising results were achieved by the SpG/CNN and the STAR/GRIEF feature, which was slightly less robust, but faster to calculate.
更多查看译文
关键词
Visual navigation,Mobile robotics,Long-term autonomy
AI 理解论文
溯源树
样例
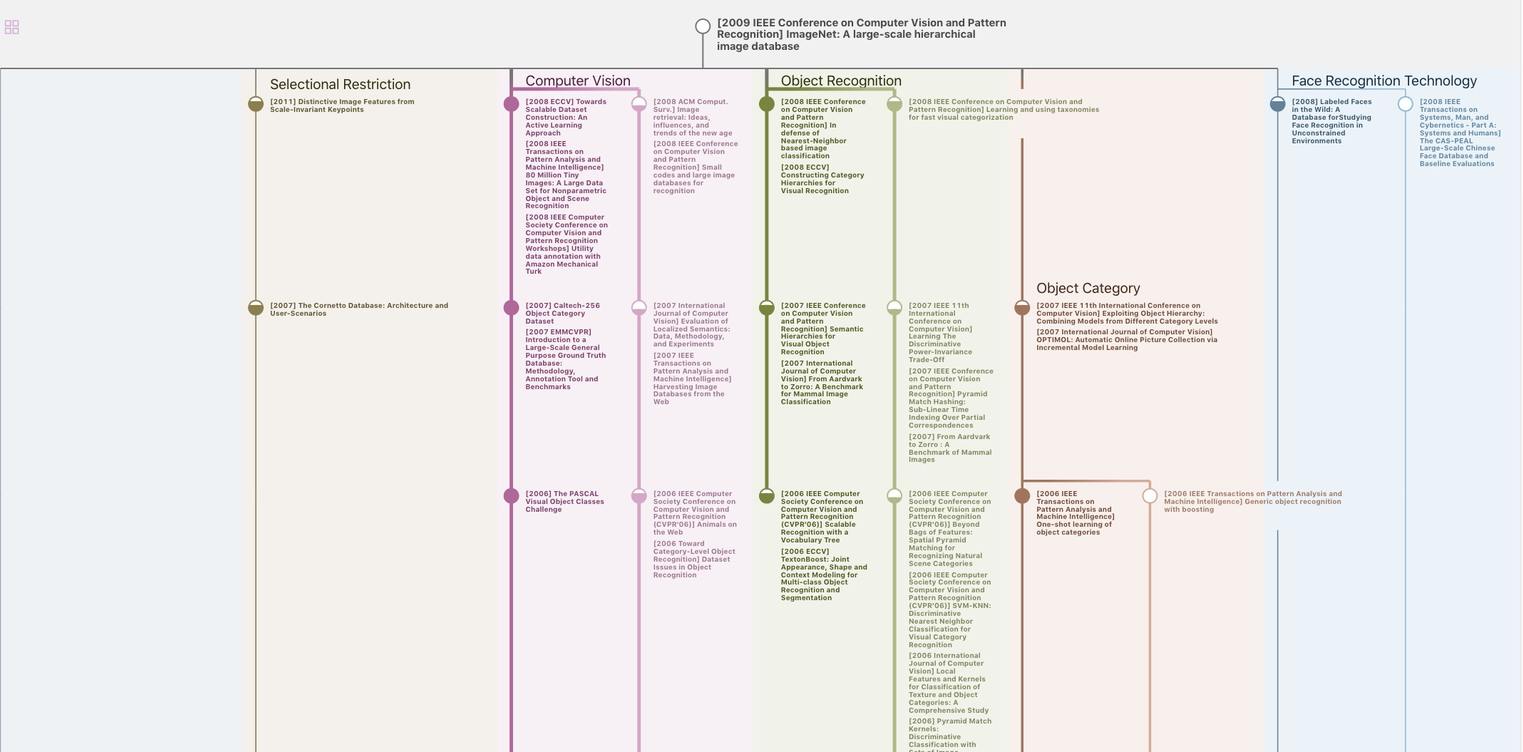
生成溯源树,研究论文发展脉络
Chat Paper
正在生成论文摘要