Learning from Collective Intelligence: Feature Learning Using Social Images and Tags.
TOMCCAP(2016)
摘要
Feature representation for visual content is the key to the progress of many fundamental applications such as annotation and cross-modal retrieval. Although recent advances in deep feature learning offer a promising route towards these tasks, they are limited in application domains where high-quality and large-scale training data are expensive to obtain. In this article, we propose a novel deep feature learning paradigm based on social collective intelligence, which can be acquired from the inexhaustible social multimedia content on the Web, in particular, largely social images and tags. Differing from existing feature learning approaches that rely on high-quality image-label supervision, our weak supervision is acquired by mining the visual-semantic embeddings from noisy, sparse, and diverse social image collections. The resultant image-word embedding space can be used to (1) fine-tune deep visual models for low-level feature extractions and (2) seek sparse representations as high-level cross-modal features for both image and text. We offer an easy-to-use implementation for the proposed paradigm, which is fast and compatible with any state-of-the-art deep architectures. Extensive experiments on several benchmarks demonstrate that the cross-modal features learned by our paradigm significantly outperforms others in various applications such as content-based retrieval, classification, and image captioning.
更多查看译文
关键词
Representation learning,visual-semantic embedding,cross-media analysis
AI 理解论文
溯源树
样例
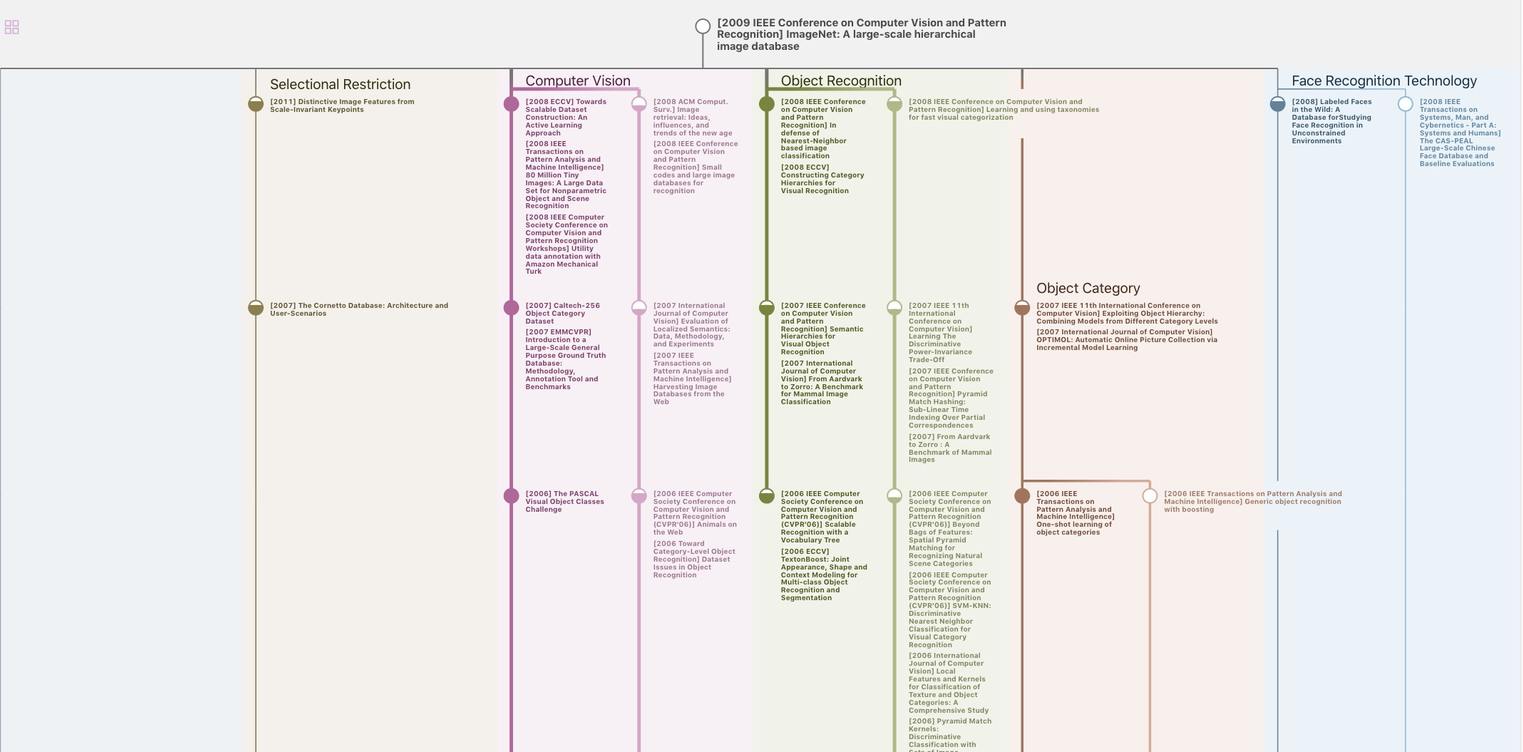
生成溯源树,研究论文发展脉络
Chat Paper
正在生成论文摘要