STT-RAM Buffer Design for Precision-Tunable General-Purpose Neural Network Accelerator
IEEE Trans. VLSI Syst.(2017)
摘要
Multilevel spin toque transfer RAM (STT-RAM) is a suitable storage device for energy-efficient neural network accelerators (NNAs), which relies on large-capacity on-chip memory to support brain-inspired large-scale learning models from conventional artificial neural networks to current popular deep convolutional neural networks. In this paper, we investigate the application of multilevel STT-RAM to general-purpose NNAs. First, the error-resilience feature of neural networks is leveraged to tolerate the read/write reliability issue in multilevel cell STT-RAM using approximate computing. The induced read/write failures at the expense of higher storage density can be effectively masked by a wide spectrum of NN applications with intrinsic forgiveness. Second, we present a precision-tunable STT-RAM buffer for the popular general-purpose NNA. The targeted STT-RAM memory design is able to transform between multiple working modes and adaptable to meet the varying quality constraint of approximate applications. Lastly, the reconfigurable STT-RAM buffer not only enables precision scaling in NNA but also provides adaptiveness to the demand for different learning models with distinct working-set sizes. Particularly, we demonstrate the concept of capacity/precision-tunable STT-RAM memory with the emerging reconfigurable deep NNA and elaborate on the data mapping and storage mode switching policy in STT-RAM memory to achieve the best energy efficiency of approximate computing.
更多查看译文
关键词
Artificial neural networks,Computer architecture,Random access memory,Biological neural networks,Approximate computing,Microprocessors,Computational modeling
AI 理解论文
溯源树
样例
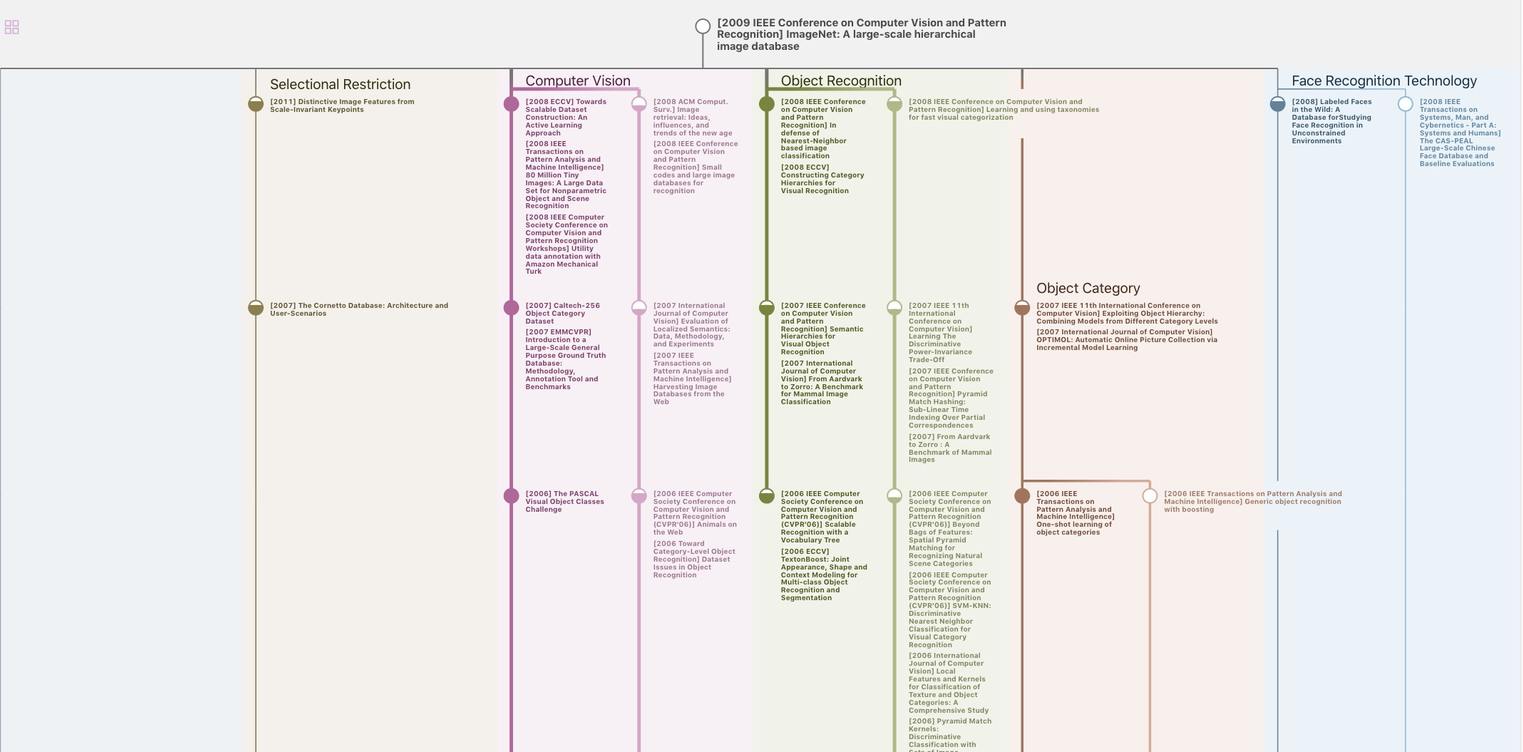
生成溯源树,研究论文发展脉络
Chat Paper
正在生成论文摘要