Forecasting of Multivariate Time Series via Complex Fuzzy Logic
IEEE Trans. Systems, Man, and Cybernetics: Systems(2017)
摘要
Multivariate time series consist of sequential vector-valued observations of some phenomenon over time. Time series forecasting (for both univariate and multivariate case) is a well-known, high-value machine learning problem, in which the goal is to predict future observations of the time series based on prior ones. Several learning algorithms based on complex fuzzy logic have recently been shown to be very accurate and compact forecasting models. However, these models have only been tested on univariate and bivariate datasets. There has as yet been no investigation of more general multivariate datasets. We report on the extension of the adaptive neuro-complex-fuzzy inferential system learning architecture to the multivariate case. We investigate single-input-single-output, multiple-input-single-output, and multiple-input-multiple-output variations of the architecture, exploring their performance on four multivariate time series. We also explore modifications to the forward- and backward-pass computations in the architecture. We find that our best designs are superior to the published results on these datasets, and at least as accurate as kernel-based prediction algorithms.
更多查看译文
关键词
Time series analysis,Forecasting,Fuzzy logic,Fuzzy sets,Machine learning algorithms,Computer architecture,MIMO
AI 理解论文
溯源树
样例
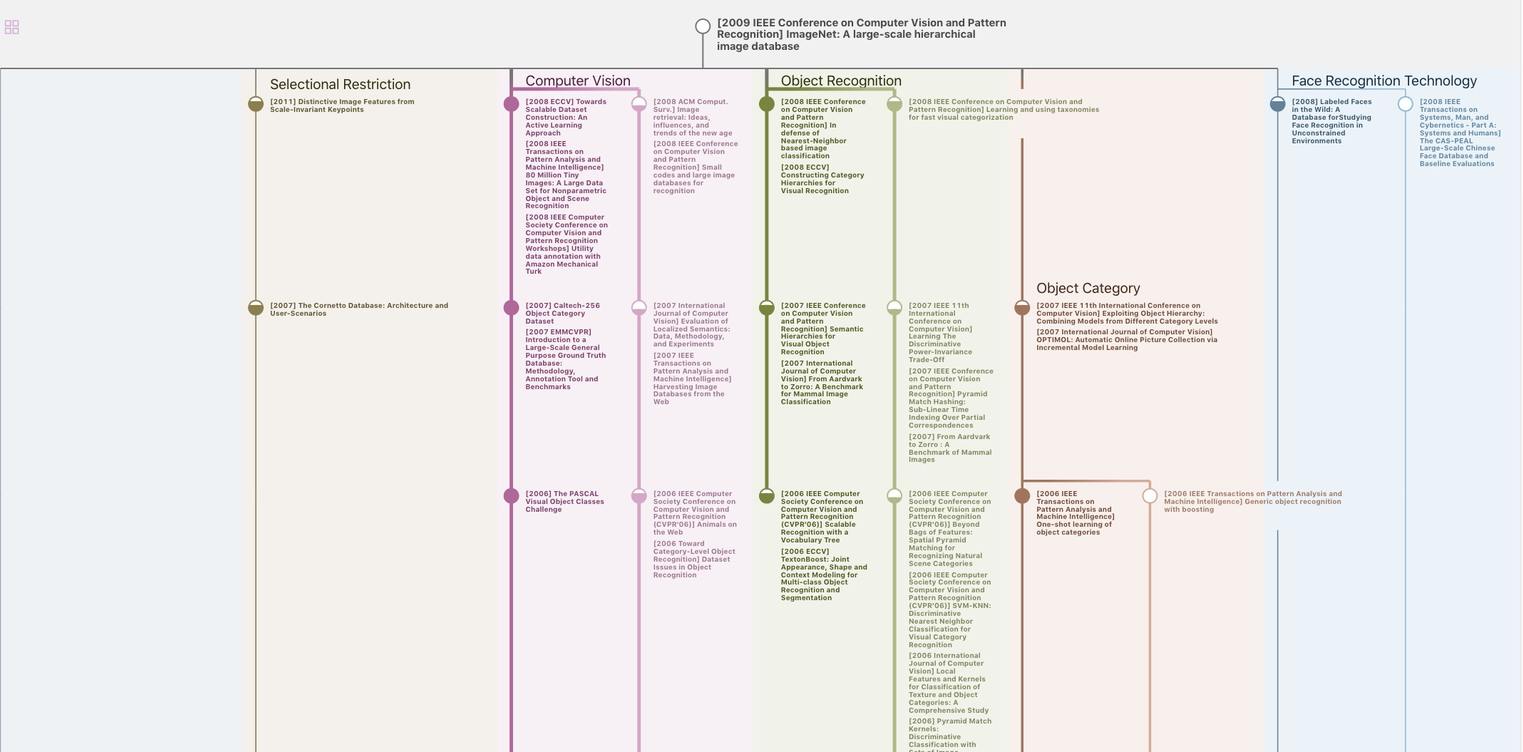
生成溯源树,研究论文发展脉络
Chat Paper
正在生成论文摘要