Mean response estimation with missing response in the presence of high-dimensional covariates
COMMUNICATIONS IN STATISTICS-THEORY AND METHODS(2017)
Abstract
This paper studies the problem of mean response estimation where missingness occurs to the response but multiple-dimensional covariates are observable. Two main challenges occur in this situation: curse of dimensionality and model specification. The non parametric imputation method relieves model specification but suffers curse of dimensionality, while some model-based methods such as inverse probability weighting (IPW) and augmented inverse probability weighting (AIPW) methods are the opposite. We propose a unified non parametric method to overcome the two challenges with the aiding of sufficient dimension reduction. It imposes no parametric structure on propensity score or conditional mean response, and thus retains the non parametric flavor. Moreover, the estimator achieves the optimal efficiency that a double robust estimator can attain. Simulations were conducted and it demonstrates the excellent performances of our method in various situations.
MoreTranslated text
Key words
SLICED INVERSE REGRESSION,SINGLE-INDEX MODELS,EMPIRICAL LIKELIHOOD,REDUCTION ESTIMATION,ASYMPTOTICS,INFERENCE,SCORE
AI Read Science
Must-Reading Tree
Example
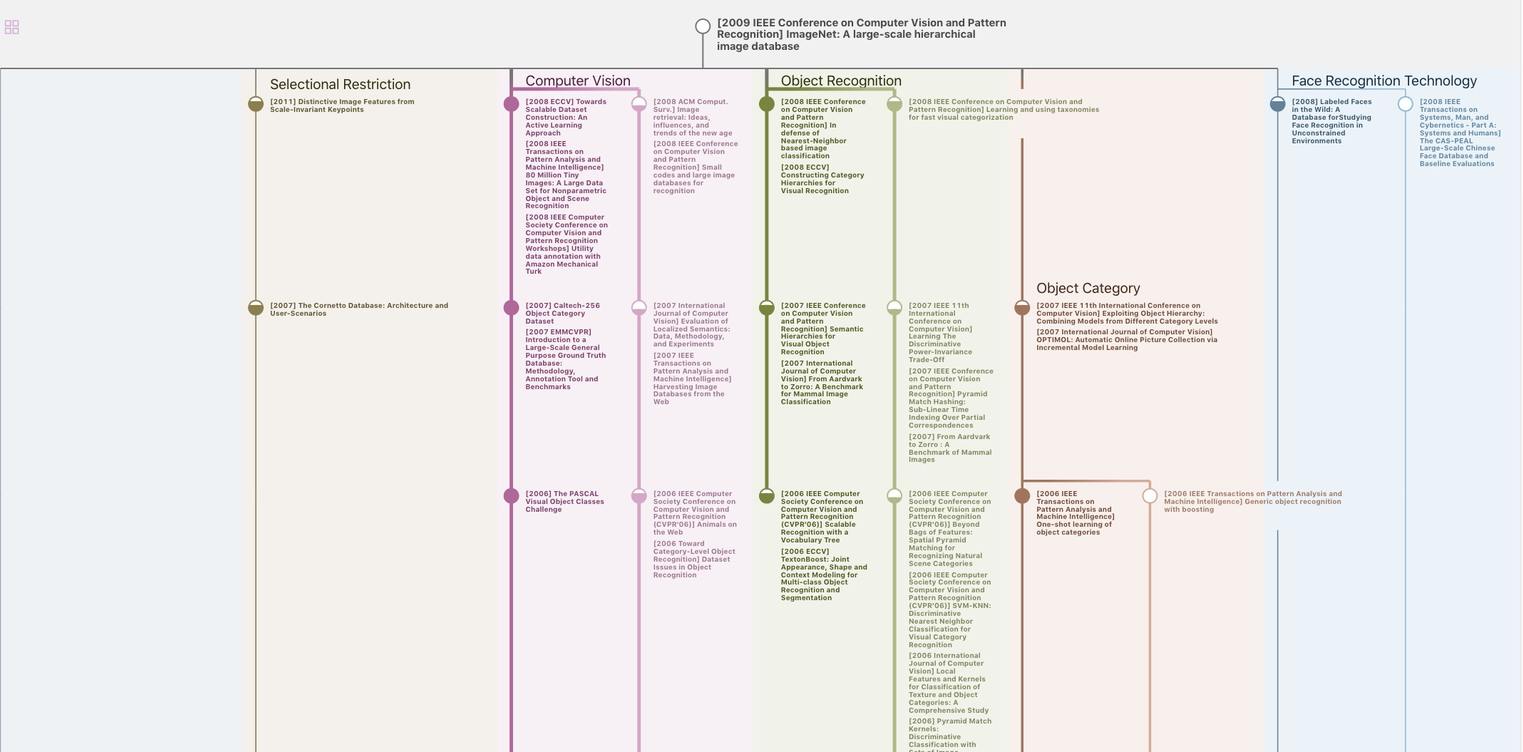
Generate MRT to find the research sequence of this paper
Chat Paper
Summary is being generated by the instructions you defined