Semi-Supervised Auto-Encoder Based On Manifold Learning
2016 International Joint Conference on Neural Networks (IJCNN)(2016)
摘要
Auto-encoder is a popular representation learning technique which can capture the generative model of data via a encoding and decoding procedure typically driven by reconstruction errors in an unsupervised way. In this paper, we propose a semi-supervised manifold learning based auto-encoder (named semAE). semAE is based on a regularized auto-encoder framework which leverages semi-supervised manifold learning to impose regularization based on the encoded representation. Our proposed approach suits more practical scenarios in which a small number of labeled data are available in addition to a large number of unlabeled data. Experiments are conducted on several well-known benchmarking datasets to validate the efficacy of semAE from the aspects of both representation and classification. The comparisons to state-of-the-art representation learning methods on classification performance in semi-supervised settings demonstrate the superiority of our approach.
更多查看译文
关键词
representation learning technique,data generative model,encoding procedure,decoding procedure,reconstruction errors,semisupervised manifold learning based auto-encoder,semAE,regularized auto-encoder framework,encoded representation,labeled data,unlabeled data,benchmarking datasets,classification performance
AI 理解论文
溯源树
样例
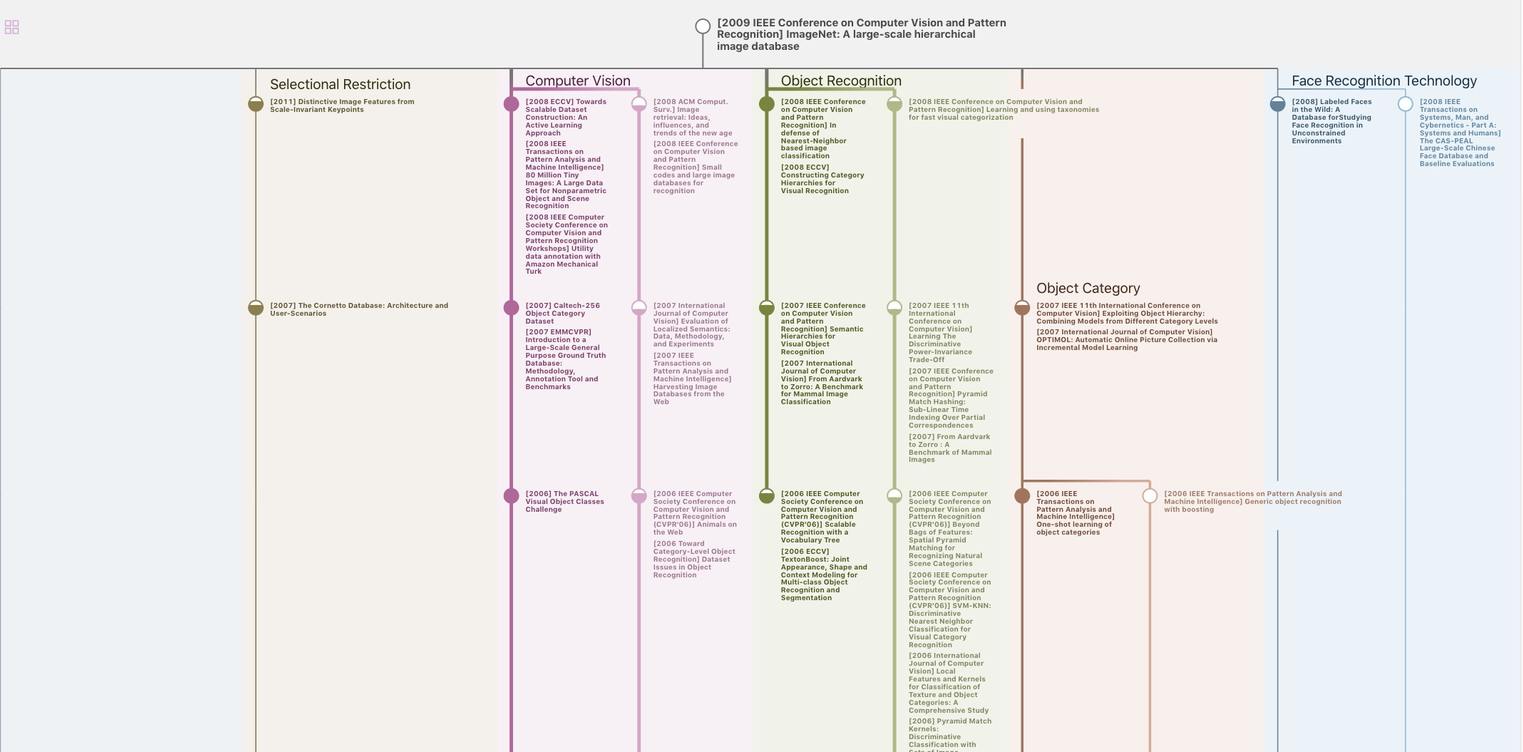
生成溯源树,研究论文发展脉络
Chat Paper
正在生成论文摘要