Classification From Generation: Recognizing Deep Grammatical Information During Reading From Rapid Event-Related Fmri
2016 International Joint Conference on Neural Networks (IJCNN)(2016)
摘要
A novel fMRI classification method designed for rapid event related fMRI experiments is described and applied to the classification of loud reading of isolated words in Hebrew. Three comparisons of different grammatical complexity were performed: (i) words versus asterisks (ii) "with diacritics versus without diacritics" and (iii) "with root versus no root". We discuss the most difficult task and, for comparison, the easiest one. Earlier work using more standard classification techniques (machine learning and statistical) succeeded fully only in the simplest of these tasks (i), but produced only partial results on (ii) and failed completely, even on the training set on the deepest task (iii).The method performs a "best match" between pre-processed data and computing a full library of artificially generated examples. The method involves a deconvolution of the rapid events on the data and performing a convolution on the generated data. The best-match is performed over all "words" constructed by convolving the response functions of each value of each event performed in a "windowed" sequence. This is accomplished separately for all voxels and then a voting procedure defines the outcome.Using the same feature selection (ANOVA) as in the earlier methods, (i) there is a dramatic increase in the accuracy rate for the third (most difficult task) on the intra-run level (88%) as well as the first task (ii) Unlike the earlier methods training and testing over all runs (within subject) achieves a significant level of classification (64% accuracy) for the training set. This shows the information for this "deeper" cognitive task can in fact be extracted from the fMRI information.
更多查看译文
关键词
Functional magnetic resonance imaging (fMRI),Multivoxel pattern analysis (MVPA),Machine Learning,Pattern Matching,Neural Networks,Cognitive Processing,Classification
AI 理解论文
溯源树
样例
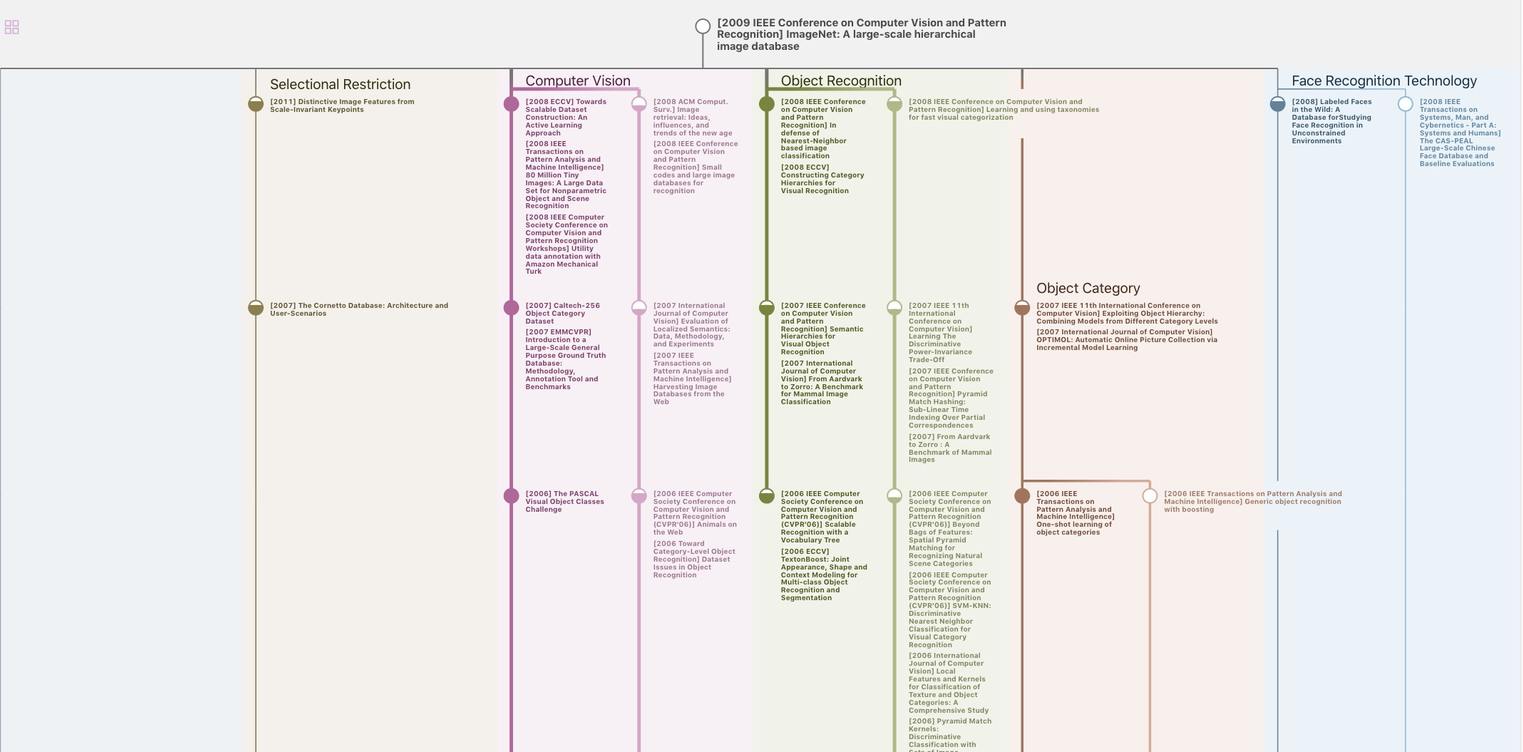
生成溯源树,研究论文发展脉络
Chat Paper
正在生成论文摘要