Dynamic group sparsity for non-negative matrix factorization with application to unsupervised source separation
2016 IEEE International Workshop on Acoustic Signal Enhancement (IWAENC)(2016)
摘要
Non-negative matrix factorization (NMF) is an appealing technique for audio source separation. Sparsity constraints are commonly used on the NMF model to discover a small number of dominant patterns. Recently, group sparsity has been proposed for NMF based methods, in which basis vectors belonging to a same group are permitted to activate together, while activations across groups are suppressed. However, most group sparsity functions activate the groups in a global manner without considering the dynamics of the speech spectra in different frames. In this paper, we propose dynamic group sparsity to model both the spectral dynamics and the temporal continuity of the speech signal and investigate its potential benefit to separate speech from other sound sources. Experimental results show that the proposed dynamic group sparsity improves the performance over regular group sparsity in unsupervised settings where neither the speaker identity nor the type of noise is known in advance.
更多查看译文
关键词
Non-negative matrix factorization,source separation,group sparsity
AI 理解论文
溯源树
样例
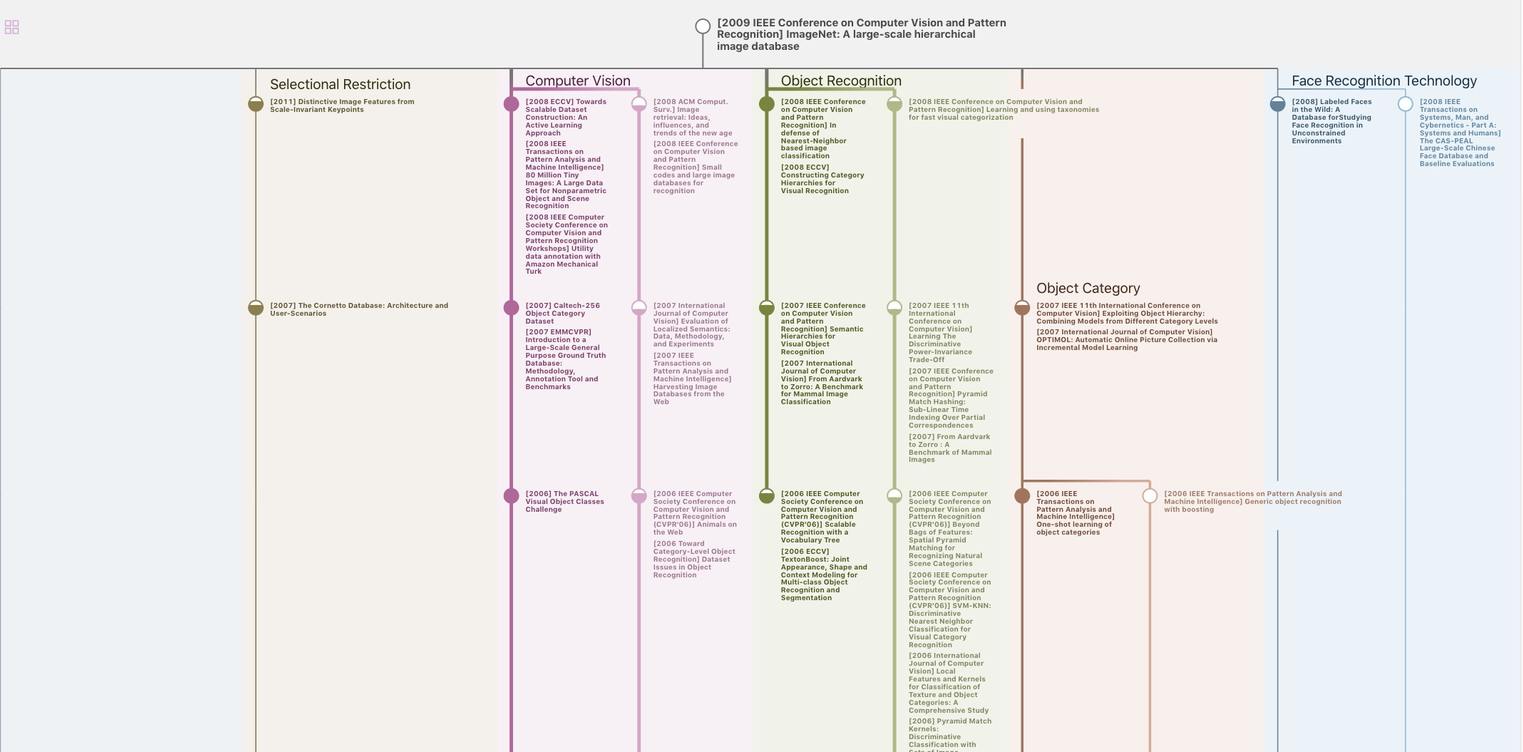
生成溯源树,研究论文发展脉络
Chat Paper
正在生成论文摘要