Trustingness & trustworthiness: A pair of complementary trust measures in a social network
ASONAM '16: Advances in Social Networks Analysis and Mining 2016 Davis California August, 2016(2016)
摘要
The increase in analysis of real life social networks has led to a better understanding of the ways humans socialize in a group. Since trust is an important part of any social interaction, researchers use such networks to understand the nuances of trust relationships. One of the major requirements in trust applications is identifying the trustworthy actors in these networks. This paper proposes a pair of complementary measures that can be used to measure trust scores of actors in a social network using involvement of social networks. Based on the proposed measures, an iterative matrix convergence algorithm is developed that calculates the trustingness and the trustworthiness of each actor in the network. Trustingness of an actor is defined as the propensity of an actor to trust his neighbors in the network. Trustworthiness, on the other hand, is defined as the willingness of the network to trust an individual actor. The algorithm is proposed based on the idea that a person having higher trustingness score contributes to the trustworthiness of its neighbors to a lower degree. Conversely, a higher trustworthiness score is a result of lots of neighbors linked to the actor having low trustingness scores. The algorithm runs in O(k × |E|) time where k denotes the number of iterations and |E| denotes the number of edges in the network. Moreover, the paper shows that the algorithm converges to a finite value quickly. Finally the proposed scores for trust prediction is implemented for various social networks and is shown that the proposed algorithm performs better (average 5%) than the state of the art trust scoring algorithms. The full version of the paper along with the coding implementation can be found at [3].
更多查看译文
关键词
social network,iterative matrix convergence algorithm,trustingness,trustworthiness,trust scoring algorithms
AI 理解论文
溯源树
样例
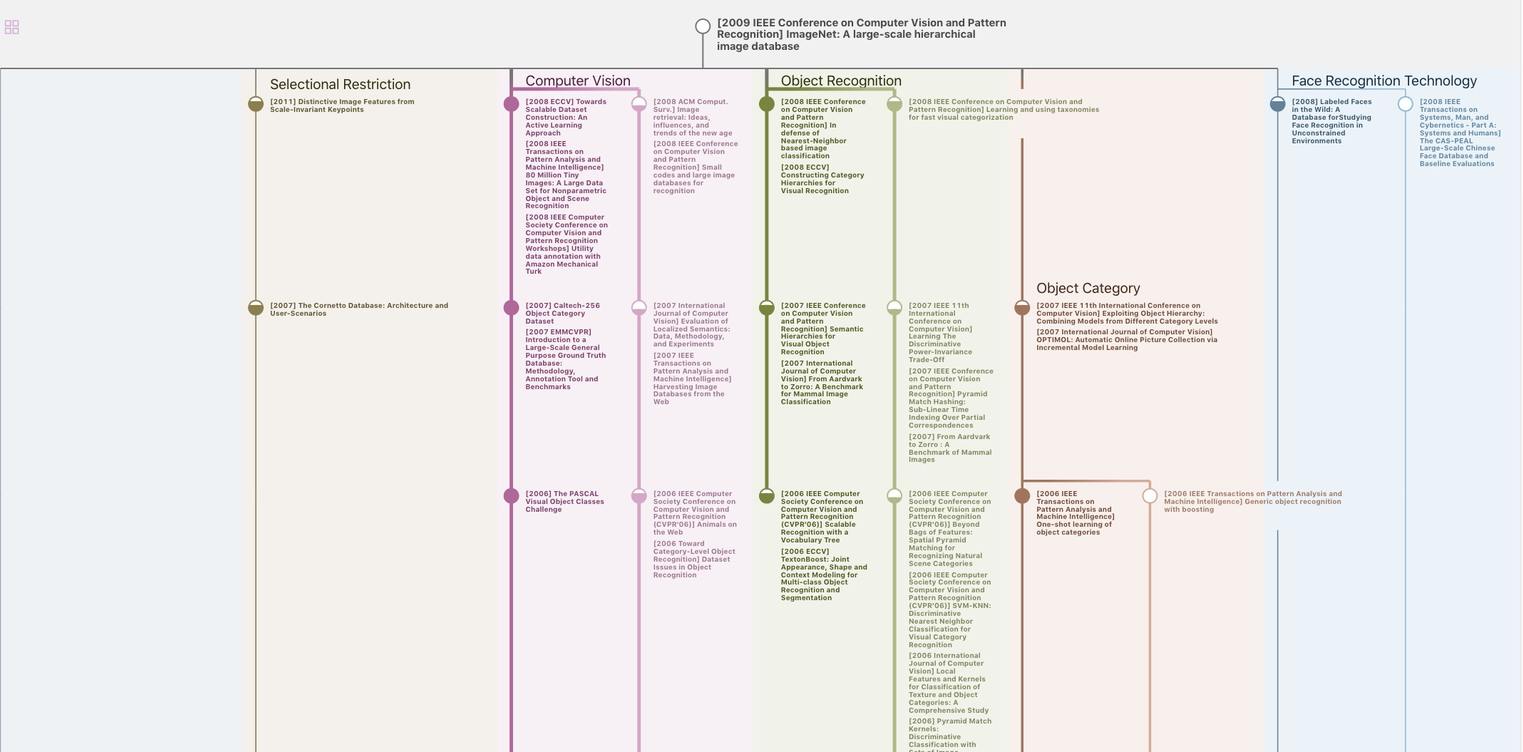
生成溯源树,研究论文发展脉络
Chat Paper
正在生成论文摘要