Cross-Modality Anatomical Landmark Detection Using Histograms of Unsigned Gradient Orientations and Atlas Location Autocontext.
Lecture Notes in Computer Science(2016)
摘要
A proof of concept is presented for cross-modality anatomical landmark detection using histograms of unsigned gradient orientations (HUGO) as machine learning image features. This has utility since an existing algorithm trained on data from one modality may be applied to data of a different modality, or data from multiple modalities may be pooled to train one modality-independent algorithm. Landmark detection is performed using a random forest trained on HUGO features and atlas location autocontext features. Three-way cross-modality detection of 20 landmarks is demonstrated in diverse cohorts of CT, MRI T1 and MRI T2 scans of the head. Each cohort is made up of 40 training and 20 test scans, making 180 scans in total. A cross-modality mean landmark error of 5.27mm is achieved, compared to single-modality error of 4.07 mm.
更多查看译文
关键词
Anatomical landmarks,Random forest,Cross-modality,Histograms of oriented gradients
AI 理解论文
溯源树
样例
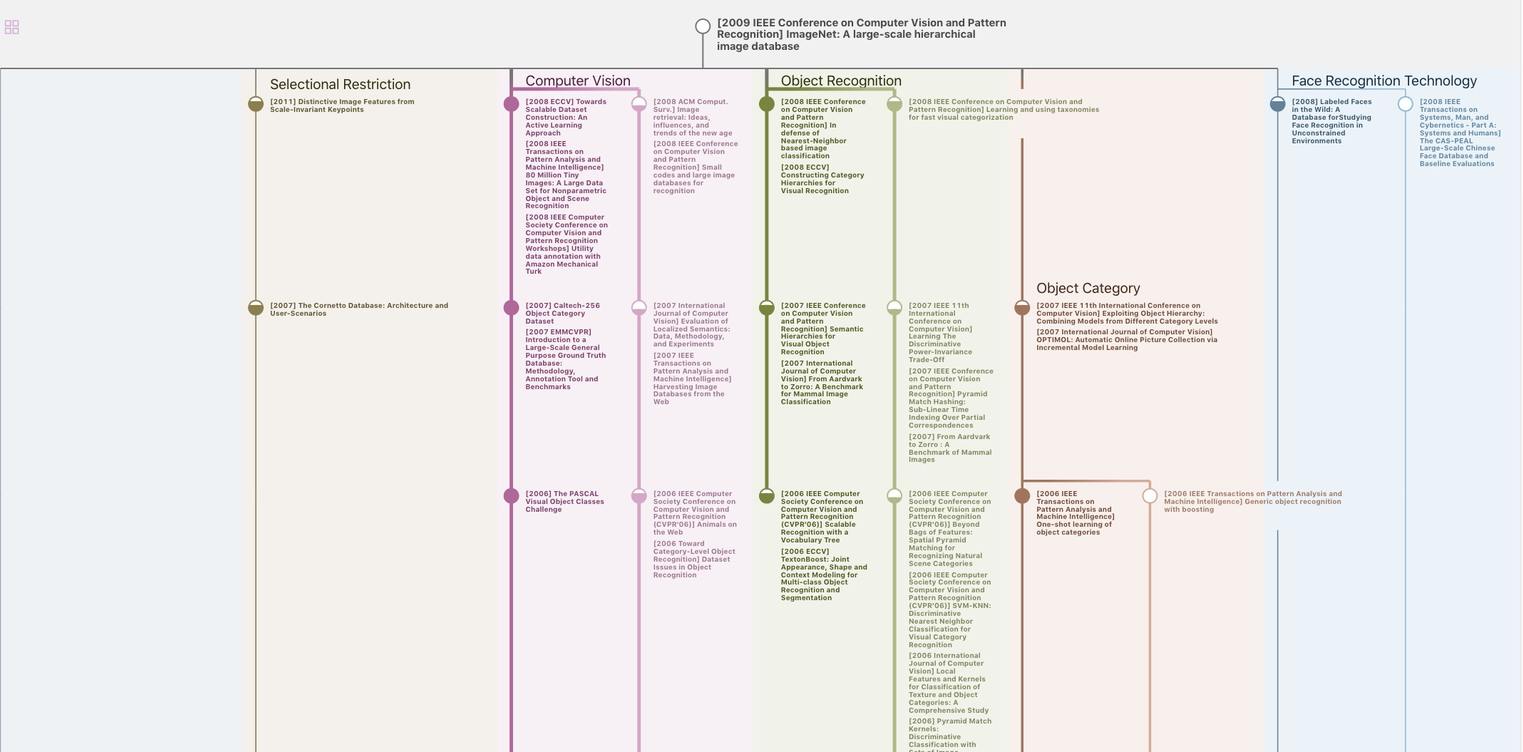
生成溯源树,研究论文发展脉络
Chat Paper
正在生成论文摘要