Structure-Aware Rank-1 Tensor Approximation for Curvilinear Structure Tracking Using Learned Hierarchical Features.
MICCAI(2016)
摘要
Tracking of curvilinear structures (CS), such as vessels and catheters, in X-ray images has become increasingly important in recent interventional applications. However, CS is often barely visible in low-dose X-ray due to overlay of multiple 3D objects in a 2D projection, making robust and accurate tracking of CS very difficult. To address this challenge, we propose a new tracking method that encodes the structure prior of CS in the rank-1 tensor approximation tracking framework, and it also uses the learned hierarchical features via a convolutional neural network (CNN). The three components, i.e., curvilinear prior modeling, high-order information encoding and automatic feature learning, together enable our algorithm to reduce the ambiguity rising from the complex background, and consequently improve the tracking robustness. Our proposed approach is tested on two sets of X-ray fluoroscopic sequences including vascular structures and catheters, respectively. In the tests our approach achieves a mean tracking error of 1.1 pixels for vascular structure and 0.8 pixels for catheter tracking, significantly outperforming state-of-the-art solutions on both datasets.
更多查看译文
AI 理解论文
溯源树
样例
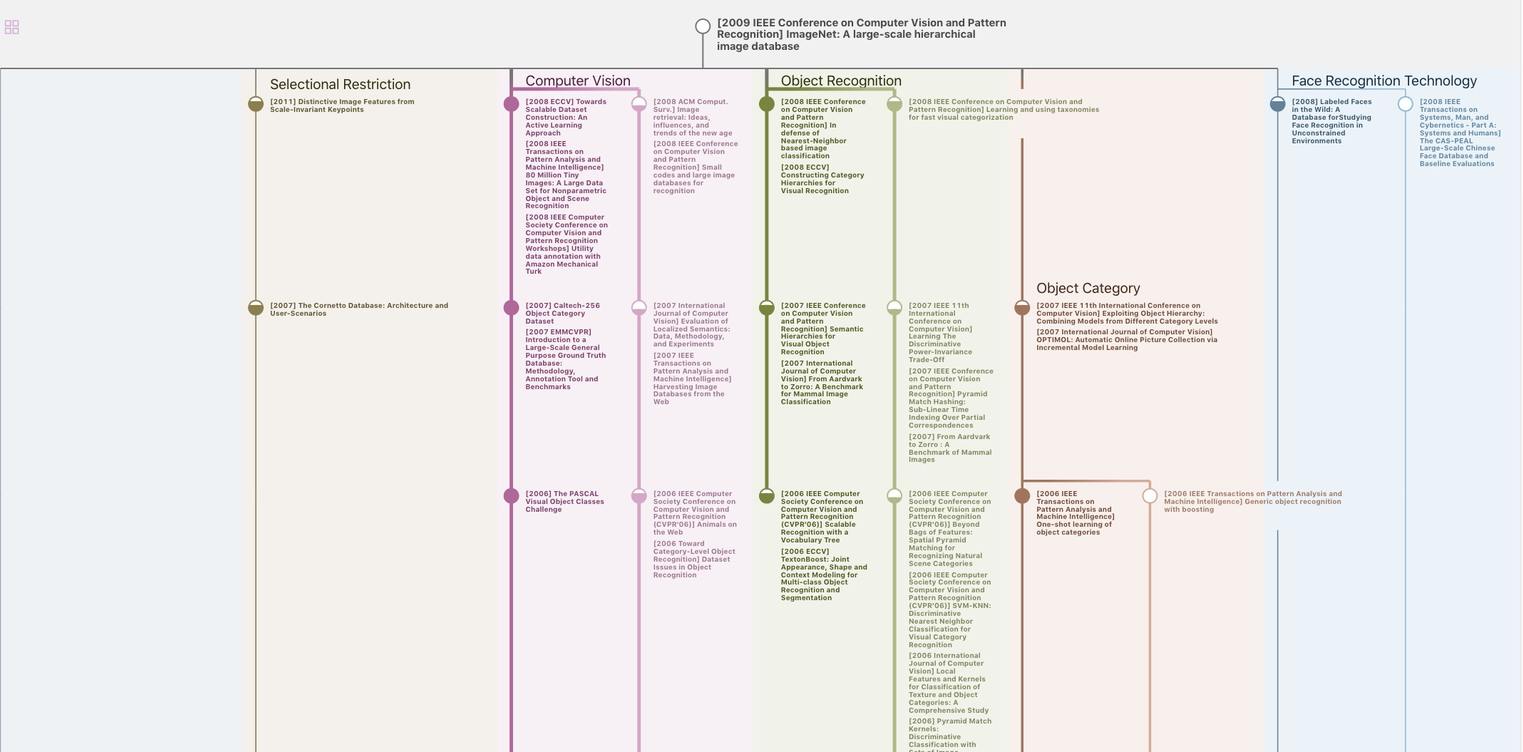
生成溯源树,研究论文发展脉络
Chat Paper
正在生成论文摘要