Fast scale-invariant lateral lumbar vertebrae detection and segmentation in X-ray images.
2016 38th Annual International Conference of the IEEE Engineering in Medicine and Biology Society (EMBC)(2016)
摘要
Fully automatic localization of lumbar vertebrae from clinical X-ray images is very challenging due to the variation of X-ray quality, scale, contrast, number of visible vertebrae, etc. To overcome these challenges, we present a novel framework, where we accelerate a scale-invariant object detection method using Support Vector Machines (SVM) trained on Histogram of Oriented Gradients (HOG) features and segmenting a fine vertebra contour using Gradient Vector Flow (GVF) based snake model. Support Vector Machines trained on HOG features are now an object detection standard in many perception fields and have demonstrated good performance on medical images as well. However, the computational complexity and lack of robustness brought by rescaling the original images have prevented its applicability. The proposed multistage detection framework uses lower-level detection result to determine the rescaling regions to reduce the region of interest, thereby decreasing the execution time. We further refine the detection result by segmenting the contour of vertebra using GVF snake, where we use edge detection techniques to increase the robustness of the GVF snake. Finally, we experimentally demonstrate the effectiveness of this framework using a large set of clinical X-ray images.
更多查看译文
关键词
Algorithms,Humans,Image Processing, Computer-Assisted,Lumbar Vertebrae,Radiography,Support Vector Machine
AI 理解论文
溯源树
样例
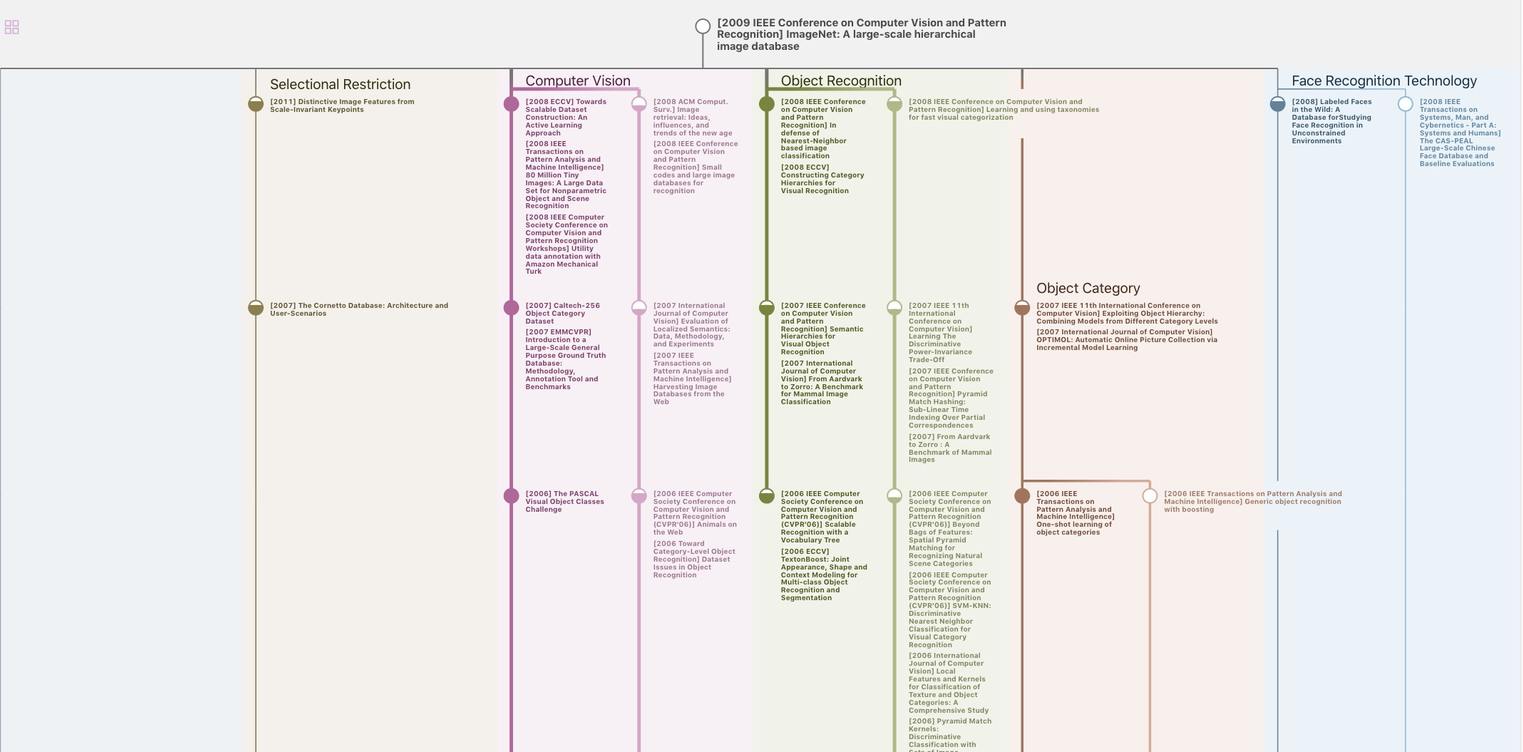
生成溯源树,研究论文发展脉络
Chat Paper
正在生成论文摘要