Multi-task transfer learning for in-hospital-death prediction of ICU patients.
2016 38TH ANNUAL INTERNATIONAL CONFERENCE OF THE IEEE ENGINEERING IN MEDICINE AND BIOLOGY SOCIETY (EMBC)(2016)
摘要
Multi-Task Transfer Learning (MTTL) is an efficient approach for learning from inter-related tasks with small sample size and imbalanced class distribution. Since the intensive care unit (ICU) data set (publicly available in Physionet) has subjects from four different ICU types, we hypothesize that there is an underlying relatedness amongst various ICU types. Therefore, this study aims to explore MTTL model for in-hospital mortality prediction of ICU patients. We used single-task learning (STL) approach on the augmented data as well as individual ICU data and compared the performance with the proposed MTTL model. As a performance measurement metrics, we used sensitivity (Sens), positive predictivity (+Pred), and Score. MTTL with class balancing showed the best performance with score of 0.78, 0.73, o.52 and 0.63 for ICU type 1 (Coronary care unit), 2 (Cardiac surgery unit), 3 (Medical ICU) and 4 (Surgical ICU) respectively. In contrast the maximum score obtained using STL approach was 0.40 for ICU type 1 & 2. These results indicates that the performance of in-hospital mortality can be improved using ICU type information and by balancing the `non-survivor' class. The findings of the study may be useful for quantifying the quality of ICU care, managing ICU resources and selecting appropriate interventions.
更多查看译文
关键词
Critical Care,Databases, Factual,Decision Support Techniques,Hospital Mortality,Humans,Intensive Care Units,Length of Stay,Models, Theoretical
AI 理解论文
溯源树
样例
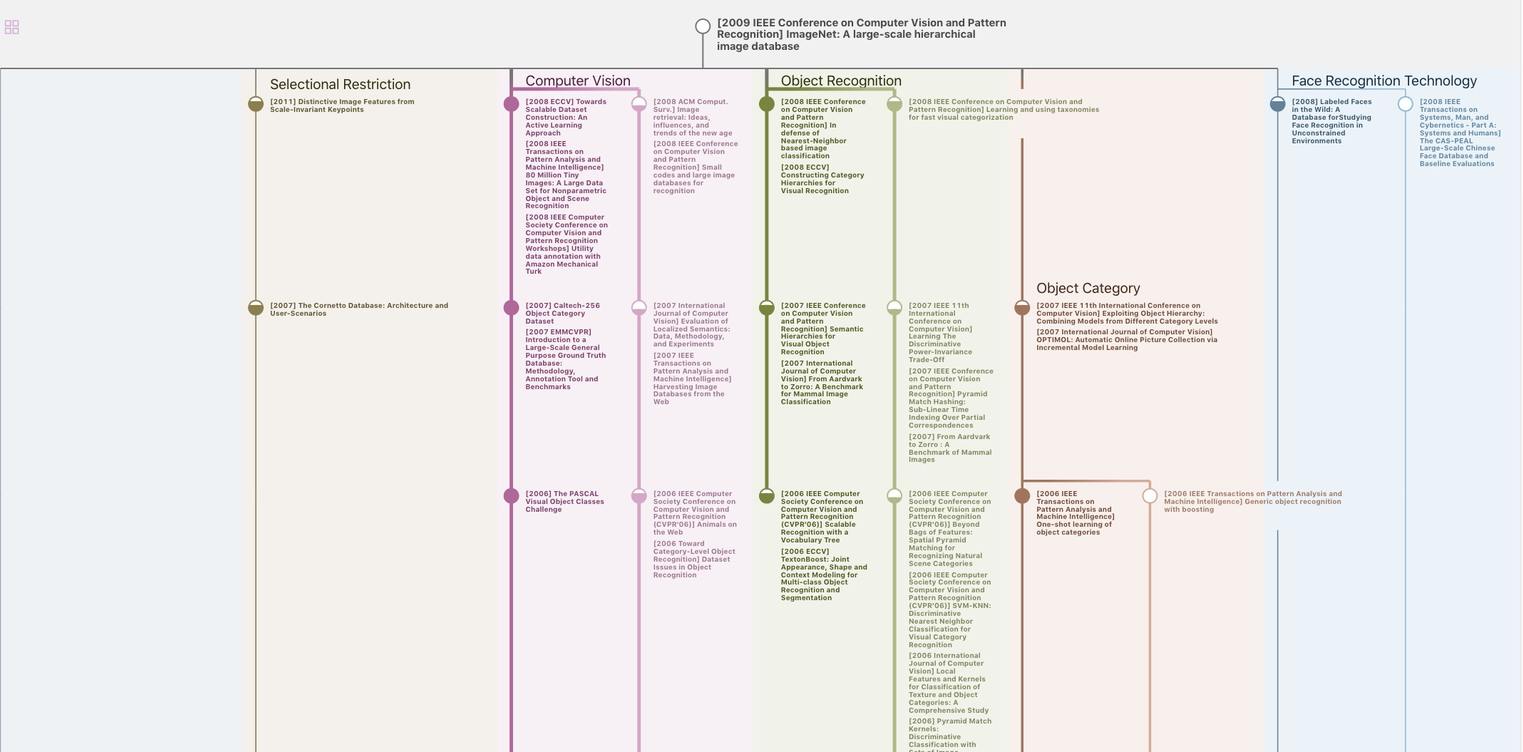
生成溯源树,研究论文发展脉络
Chat Paper
正在生成论文摘要