Semi-supervised Learning for Mongolian Morphological Segmentation.
CCL(2016)
Abstract
Unlike previous Mongolian morphological segmentation methods based on large labeled training data or complicated rules concluded by linguists, we explore a novel semi-supervised method for a practical application, i.e., statistical machine translation (SMT), based on a low-resource learning setting, in which a small amount of labeled data and large amount of unlabeled data are available. First, a CRF-based supervised learning is exploited to predict morpheme boundaries by using small labeled data. Then, a lexicon-based segmentation model with small labeled data as the heuristic information is used to compensate the weakness in the first step by the abundant unlabeled data. Finally, we present some error correction models to revise segmentation results. Experimental results show that our method can improve the segmentation results compared with the pure supervised learning. Besides, we integrate the morphological segmentation result into Chinese-Mongolian SMT and achieve the satisfactory performance compared with the baseline.
MoreTranslated text
Key words
Semi-supervised learning, Morphological segmentation, Statistical machine translation, Low-resource language
AI Read Science
Must-Reading Tree
Example
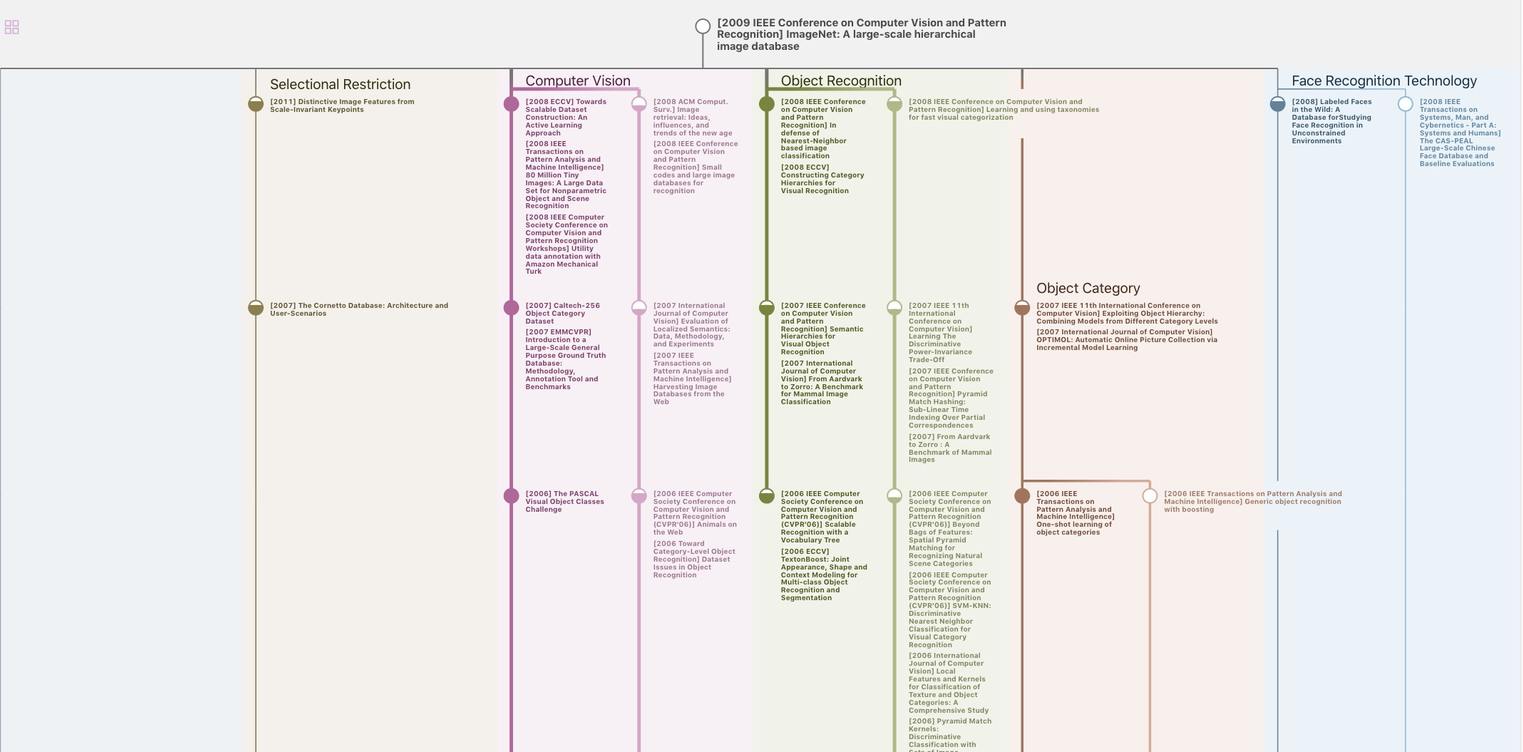
Generate MRT to find the research sequence of this paper
Chat Paper
Summary is being generated by the instructions you defined