Weighted similarity-based clustering of chemical structures and bioactivity data in early drug discovery.
JOURNAL OF BIOINFORMATICS AND COMPUTATIONAL BIOLOGY(2016)
摘要
The modern process of discovering candidate molecules in early drug discovery phase includes a wide range of approaches to extract vital information from the intersection of biology and chemistry. A typical strategy in compound selection involves compound clustering based on chemical similarity to obtain representative chemically diverse compounds (not incorporating potency information). In this paper, we propose an integrative clustering approach that makes use of both biological (compound efficacy) and chemical (structural features) data sources for the purpose of discovering a subset of compounds with aligned structural and biological properties. The datasets are integrated at the similarity level by assigning complementary weights to produce a weighted similarity matrix, serving as a generic input in any clustering algorithm. This new analysis work flow is semi-supervised method since, after the determination of clusters, a secondary analysis is performed wherein it finds differentially expressed genes associated to the derived integrated cluster(s) to further explain the compound-induced biological effects inside the cell. In this paper, datasets from two drug development oncology projects are used to illustrate the usefulness of the weighted similarity-based clustering approach to integrate multi-source high-dimensional information to aid drug discovery. Compounds that are structurally and biologically similar to the reference compounds are discovered using this proposed integrative approach.
更多查看译文
关键词
Bioactivity,chemical structure,clustering,transcriptomic
AI 理解论文
溯源树
样例
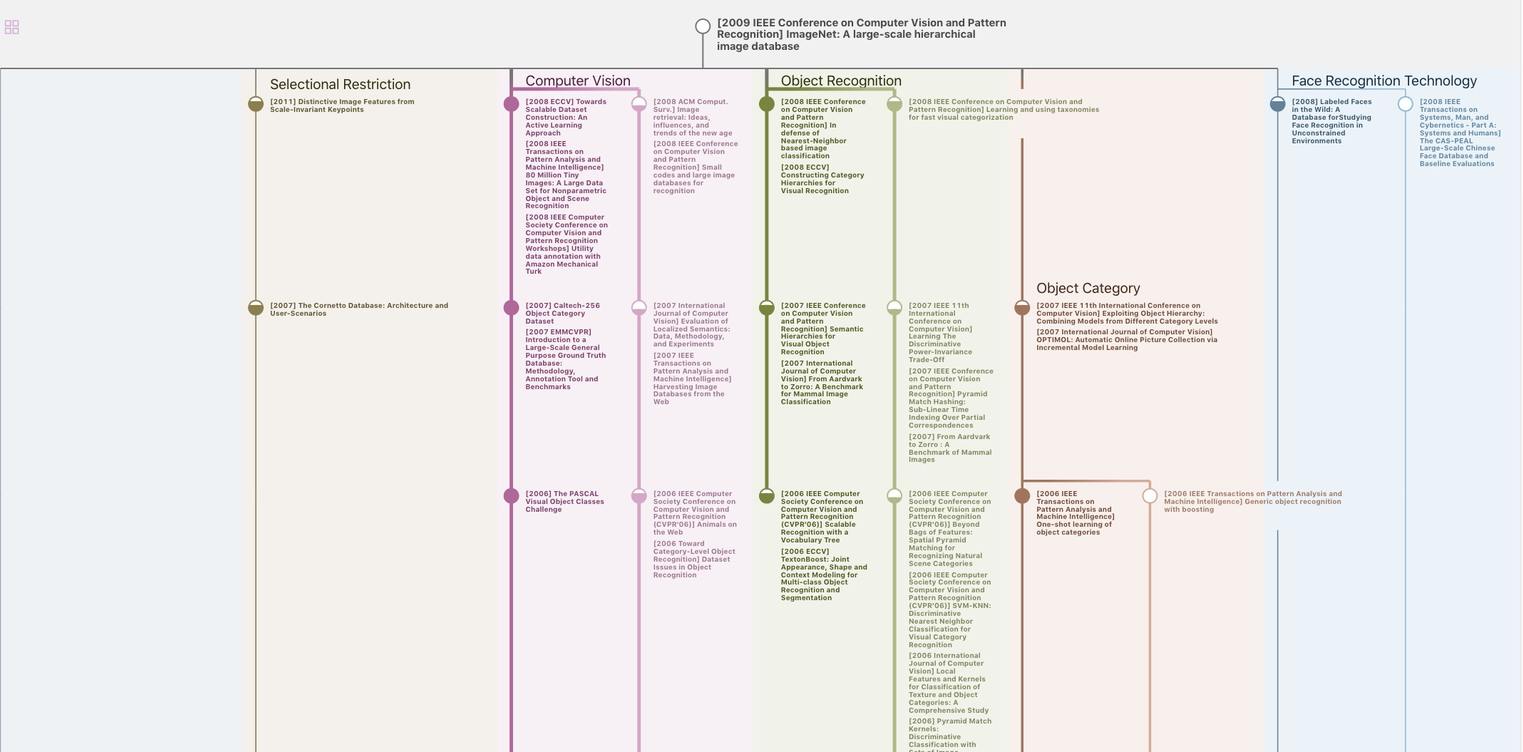
生成溯源树,研究论文发展脉络
Chat Paper
正在生成论文摘要