Deep Multitask Metric Learning for Offline Signature Verification.
Pattern Recognition Letters(2016)
摘要
A new deep multitask learning based metric learning method is proposed.An appropriate architecture is proposed for offline signature verification.The knowledge of other signers' samples is transfered for better accuracy.The proposed method outperforms SVM and Discriminative Deep Metric Learning.It outperforms the accuracy of other published reports. Display Omitted This paper presents a novel classification method, Deep Multitask Metric Learning (DMML), for offline signature verification. Unlike existing methods that to verify questioned signatures of an individual merely consider the training samples of that class, DMML uses the knowledge from the similarities and dissimilarities between the genuine and forged samples of other classes too. To this end, using the idea of multitask and transfer learning, DMML train a distance metric for each class together with other classes simultaneously. DMML has a structure with a shared layer acting as a writer-independent approach, that is followed by separated layers which learn writer-dependent factors. We compare the proposed method against SVM, writer-dependent and writer-independent Discriminative Deep Metric Learning method on four offline signature datasets (UTSig, MCYT-75, GPDSsynthetic, and GPDS960GraySignatures) using Histogram of Oriented Gradients (HOG) and Discrete Radon Transform (DRT) features. Results of our experiments show that DMML achieves better performance compared to other methods in verifying genuine signatures, skilled and random forgeries.
更多查看译文
关键词
Signature verification,Metric Learning,Multitask Learning,Transfer Learning
AI 理解论文
溯源树
样例
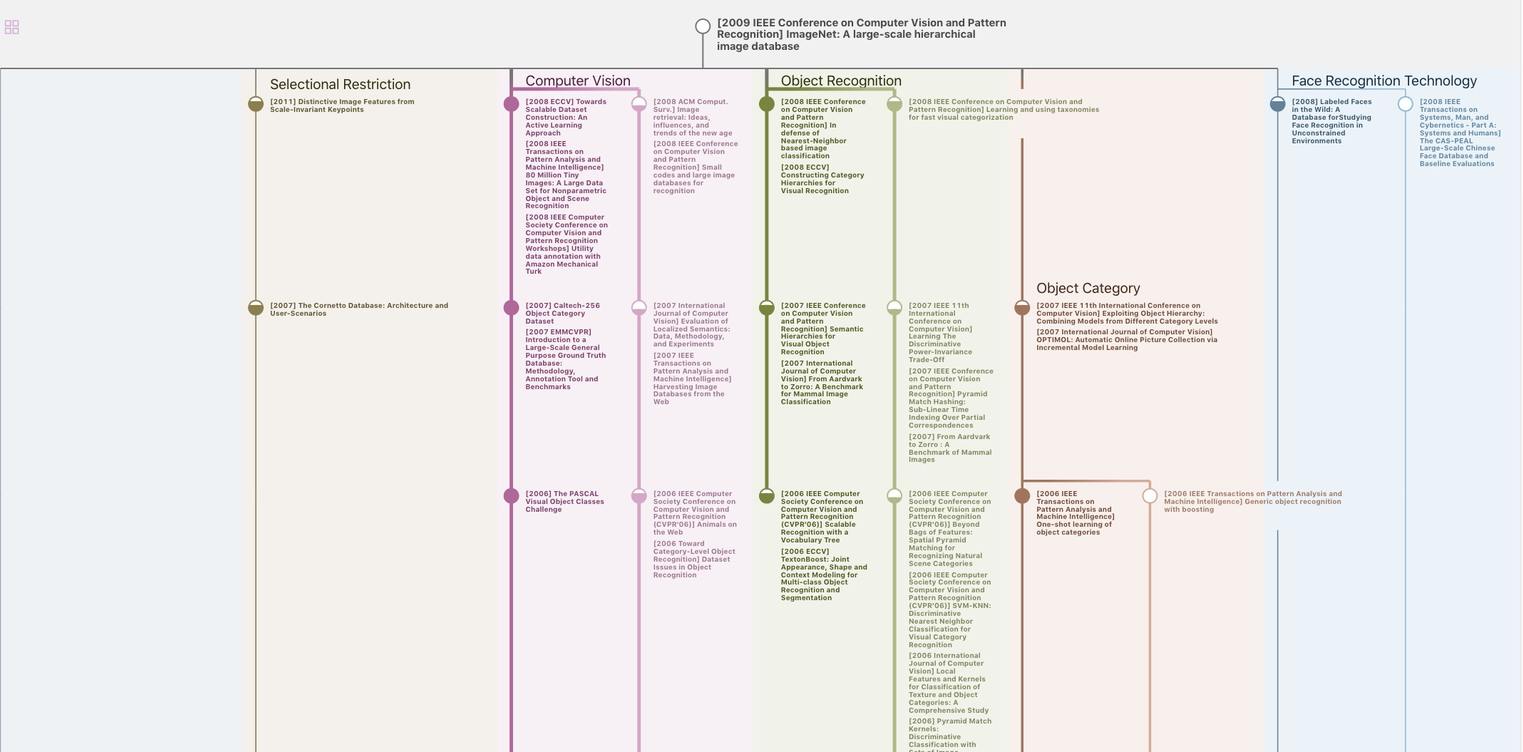
生成溯源树,研究论文发展脉络
Chat Paper
正在生成论文摘要