Toward semantic attributes in dictionary learning and non-negative matrix factorization.
Pattern Recognition Letters(2016)
摘要
We proposed new algorithms for dictionary learning and non-negative matrix factorization.We utilize relative attributes as semantic information to enhance the discriminative property of obtained signals.We apply our method to several datasets to show the efficiency of proposed algorithms.k-means clustering is used to measure the efficiency of new features.Experimental results show the efficiency of relative attributes instead of binary labels in providing discriminative dictionary or subspace. Display Omitted Binary label information is widely used semantic information in discriminative dictionary learning and non-negative matrix factorization. A Discriminative Dictionary Learning (DDL) algorithm uses the label of some data samples to enhance the discriminative property of sparse signals. A discriminative Non-negative Matrix Factorization (NMF) utilizes label information in learning discriminative bases. All these technique are using binary label information as semantic information. In contrast to such binary attributes or labels, relative attributes contain richer semantic information where the data is annotated with the strength of the attributes. In this paper, we utilize the relative attributes of training data in non-negative matrix factorization and dictionary learning. Precisely, we learn rank functions (one for each predefined attribute) to rank the images based on predefined semantic attributes. The strength of each attribute in a data sample is used as semantic information. To assess the quality of the obtained signals, we apply k-means clustering and measure the performance for clustering. Experimental results conducted on three datasets, namely PubFig (16), OSR (24) and Shoes (15) confirm that the proposed approach outperforms the state-of-the-art discriminative algorithms.
更多查看译文
关键词
Dictionary,Matrix factorization,Attributes,Learning
AI 理解论文
溯源树
样例
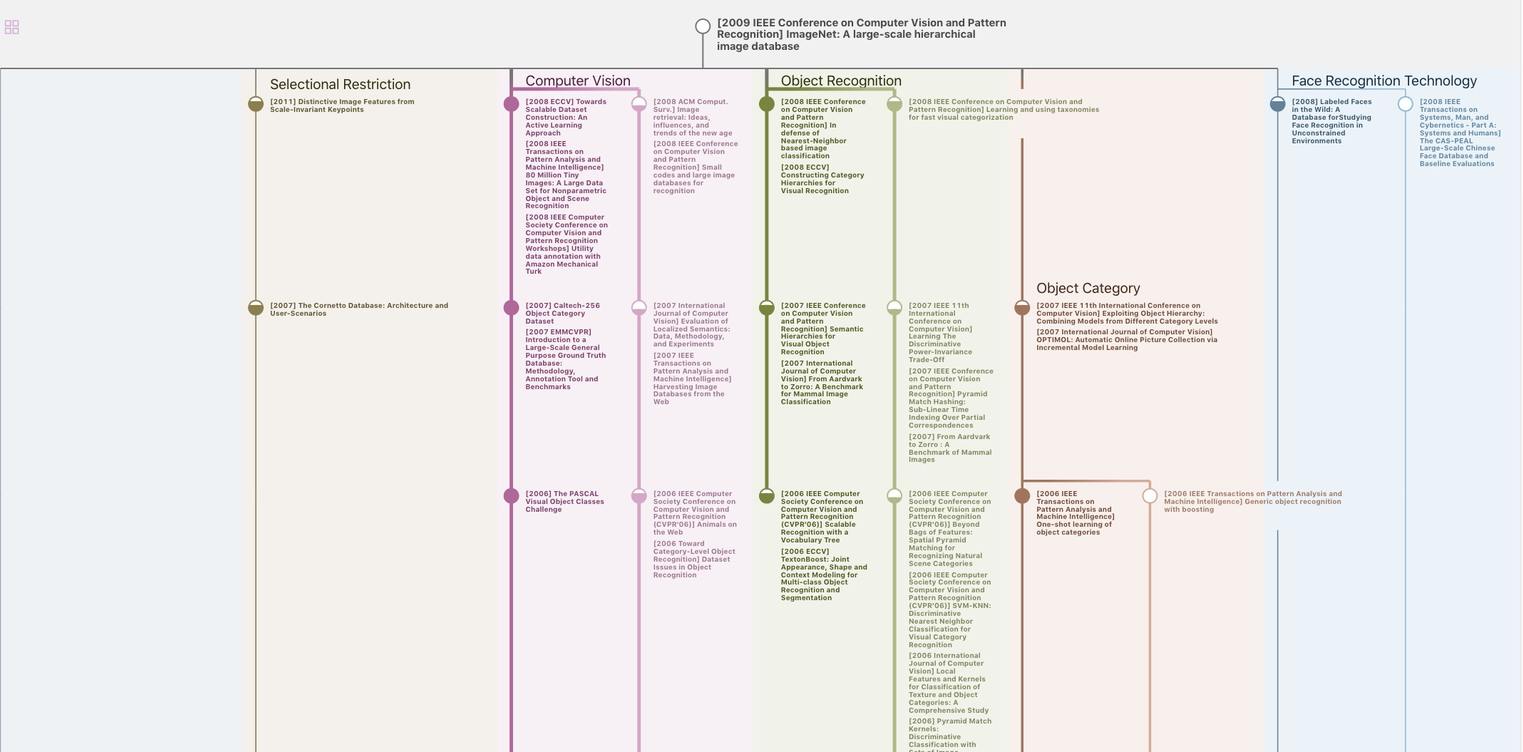
生成溯源树,研究论文发展脉络
Chat Paper
正在生成论文摘要