Online Bagging And Boosting For Imbalanced Data Streams
IEEE Transactions on Knowledge and Data Engineering(2016)
摘要
While both cost-sensitive learning and online learning have been studied separately, these two issues have seldom been addressed simultaneously. Yet, there are many applications where both aspects are important. This paper investigates a class of algorithmic approaches suitable for online cost-sensitive learning, designed for such problems. The basic idea is to leverage existing methods for online ensemble algorithms, and combine these with batch mode methods for cost-sensitive bagging/boosting algorithms. Within this framework, we describe several theoretically sound online cost-sensitive bagging and online cost-sensitive boosting algorithms, and show that the convergence of the proposed algorithms is guaranteed under certain conditions. We then present extensive experimental results on benchmark datasets to compare the performance of the various proposed approaches.
更多查看译文
关键词
Bagging,boosting,ensemble learning,cost-sensitive learning,online learning,class imbalance problem
AI 理解论文
溯源树
样例
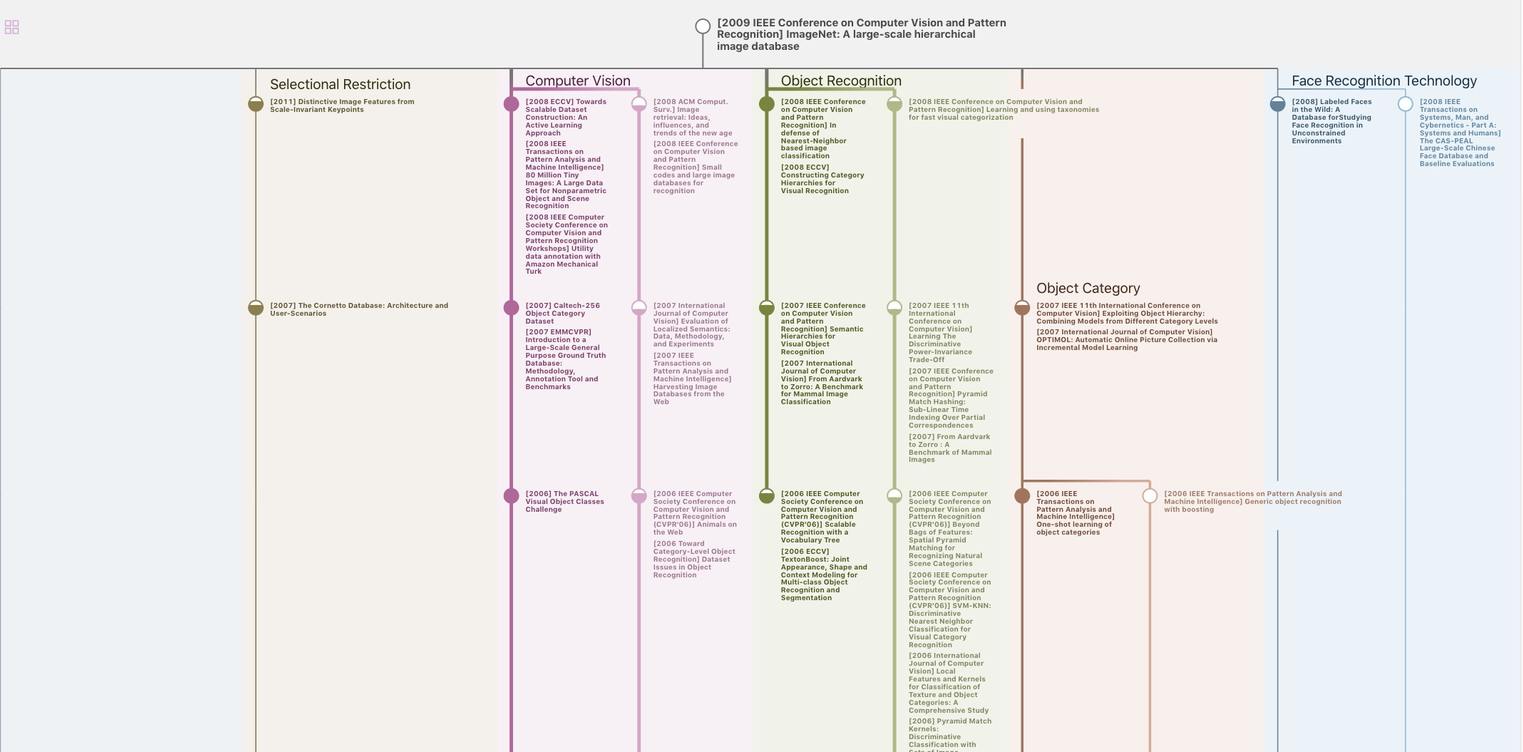
生成溯源树,研究论文发展脉络
Chat Paper
正在生成论文摘要