Discovering Anomalies on Mixed-Type Data Using a Generalized Student- t Based Approach.
IEEE Trans. Knowl. Data Eng.(2016)
摘要
Anomaly detection in mixed-type data is an important problem that has not been well addressed in the machine learning field. Existing approaches focus on computational efficiency and their correlation modeling between mixed-type attributes is heuristically driven, lacking a statistical foundation. In this paper, we propose MIxed-Type Robust dEtection (MITRE), a robust error buffering approach for anomaly detection in mixed-type datasets. Because of its non-Gaussian design, the problem is analytically intractable. Two novel Bayesian inference approaches are utilized to solve the intractable inferences: Integrated-nested Laplace Approximation (INLA), and Expectation Propagation (EP) with Variational Expectation-Maximization (EM). A set of algorithmic optimizations is implemented to improve the computational efficiency. A comprehensive suite of experiments was conducted on both synthetic and real world data to test the effectiveness and efficiency of MITRE.
更多查看译文
关键词
Robustness,Numerical models,Correlation,Data models,Bayes methods,Computational modeling,Estimation
AI 理解论文
溯源树
样例
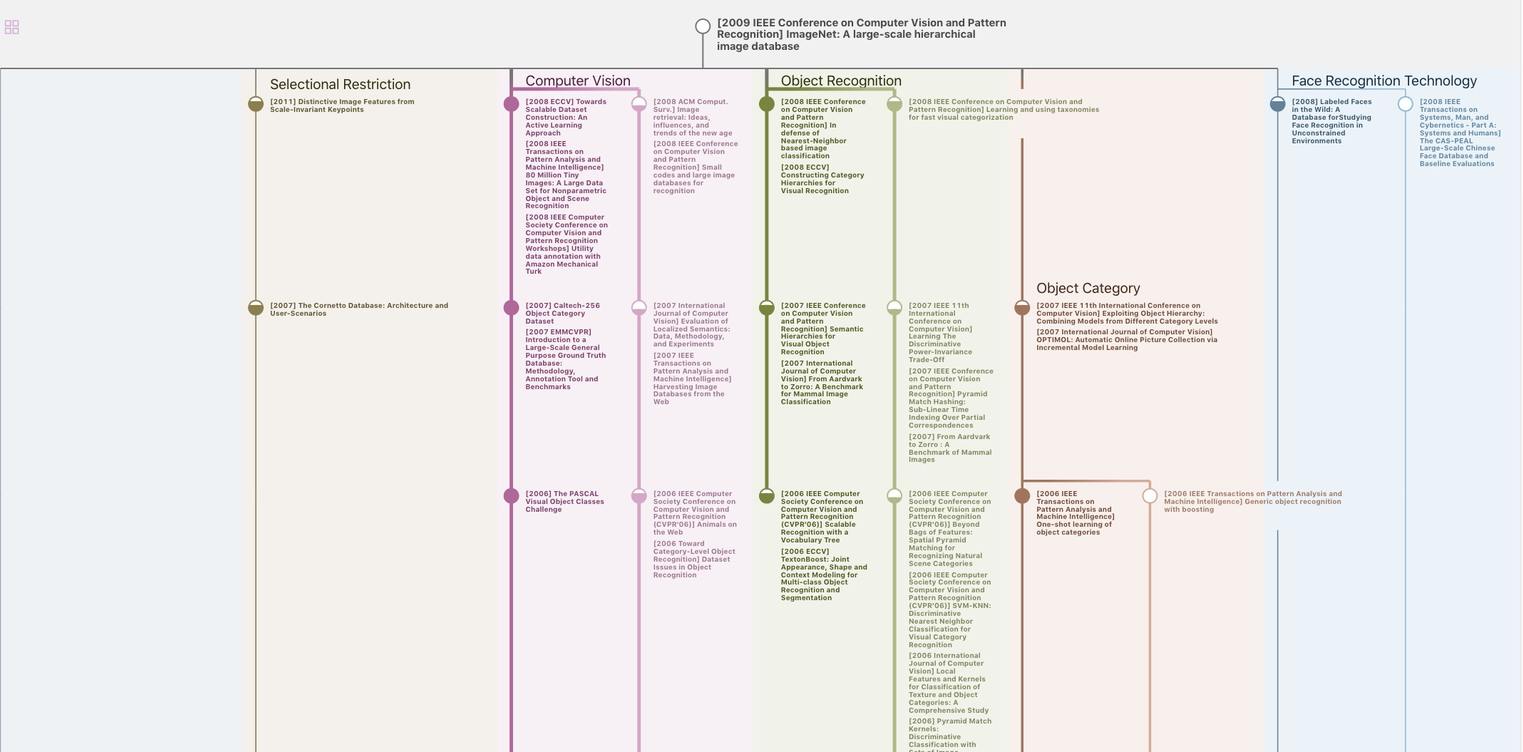
生成溯源树,研究论文发展脉络
Chat Paper
正在生成论文摘要