TaskMe: Multi-Task Allocation in Mobile Crowd Sensing
UBICOMP(2016)
摘要
Task allocation or participant selection is a key issue in Mobile Crowd Sensing (MCS). While previous participant selection approaches mainly focus on selecting a proper subset of users for a single MCS task, multi-task-oriented participant selection is essential and useful for the efficiency of large-scale MCS platforms. This paper proposes TaskMe, a participant selection framework for multi-task MCS environments. In particular, two typical multi-task allocation situations with bi-objective optimization goals are studied: (1) For FPMT (few participants, more tasks), each participant is required to complete multiple tasks and the optimization goal is to maximize the total number of accomplished tasks while minimizing the total movement distance. (2) For MPFT (more participants, few tasks), each participant is selected to perform one task based on pre-registered working areas in view of privacy, and the optimization objective is to minimize total incentive payments while minimizing the total traveling distance. Two optimal algorithms based on the Minimum Cost Maximum Flow theory are proposed for FPMT, and two algorithms based on the multi-objective optimization theory are proposed for MPFT. Experiments verify that the proposed algorithms outperform baselines based on a large-scale real-word dataset under different experiment settings (the number of tasks, various task distributions, etc.).
更多查看译文
关键词
Mobile crowd sensing,multi-task allocation,participant selection,bi-objective optimization
AI 理解论文
溯源树
样例
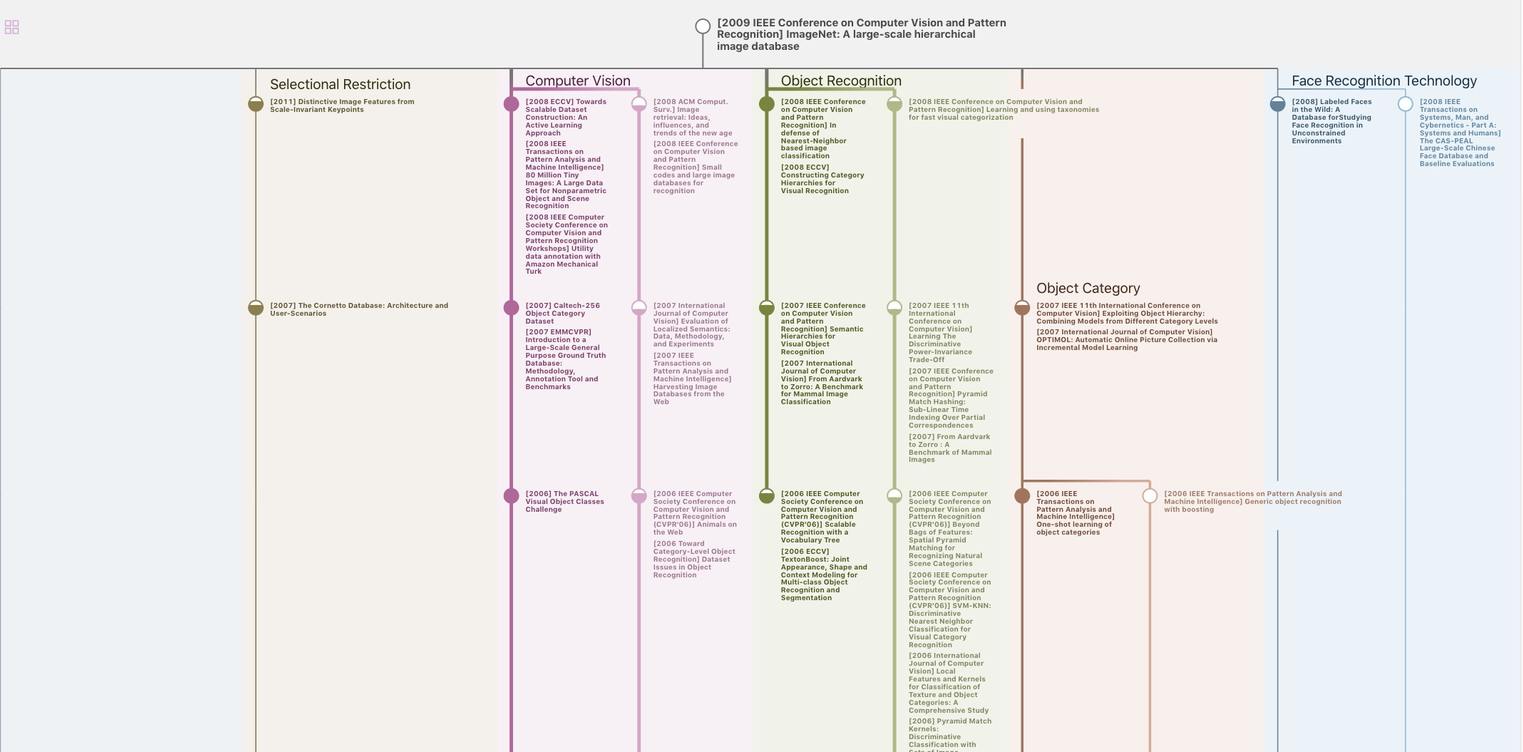
生成溯源树,研究论文发展脉络
Chat Paper
正在生成论文摘要