RETAIN: Interpretable Predictive Model in Healthcare using Reverse Time Attention Mechanism.
CoRR(2016)
摘要
Accuracy and interpretation are two goals of any successful predictive models. Most existing works have to suffer the tradeoff between the two by either picking complex black box models such as recurrent neural networks (RNN) or relying on less accurate traditional models with better interpretation such as logistic regression. To address this dilemma, we present REverse Time AttentIoN model (RETAIN) for analyzing EHR data that achieves high accuracy while remaining clinically interpretable. RETAIN is a two-level neural attention model that can find influential past visits and significant clinical variables within those visits (e.g,. key diagnoses). RETAIN mimics physician practice by attending the EHR data in a reverse time order so that more recent clinical visits will likely get higher attention. Experiments on a large real EHR dataset of 14 million visits from 263K patients over 8 years confirmed the comparable predictive accuracy and computational scalability to the state-of-the-art methods such as RNN. Finally, we demonstrate the clinical interpretation with concrete examples from RETAIN.
更多查看译文
关键词
reverse time attention mechanism,interpretable predictive model,healthcare
AI 理解论文
溯源树
样例
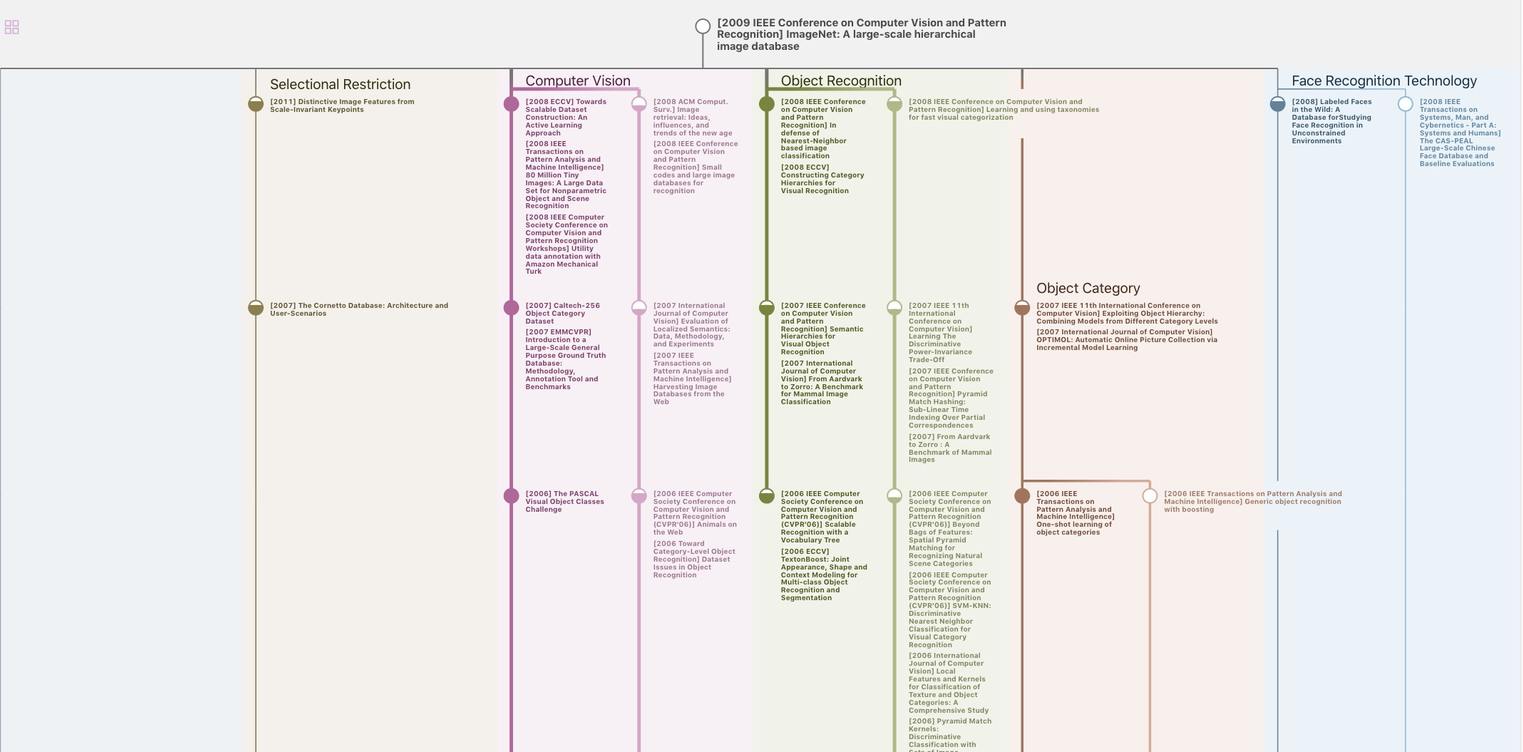
生成溯源树,研究论文发展脉络
Chat Paper
正在生成论文摘要