Schema Independent and Scalable Relational Learning By Castor.
PVLDB(2016)
摘要
Learning novel relations from relational databases is an important problem with many applications in database systems and machine learning. Relational learning algorithms leverage the properties of the database schema to find the definition of the target relation in terms of the existing relations in the database. However, the same data set may be represented under different schemas for various reasons, such as efficiency and data quality. Unfortunately, current relational learning algorithms tend to vary quite substantially over the choice of schema, which complicates their off-the-shelf application. We demonstrate Castor, a relational learning system that efficiently learns the same definitions over common schema variations. The results of Castor are more accurate than well-known learning systems over large data.
更多查看译文
AI 理解论文
溯源树
样例
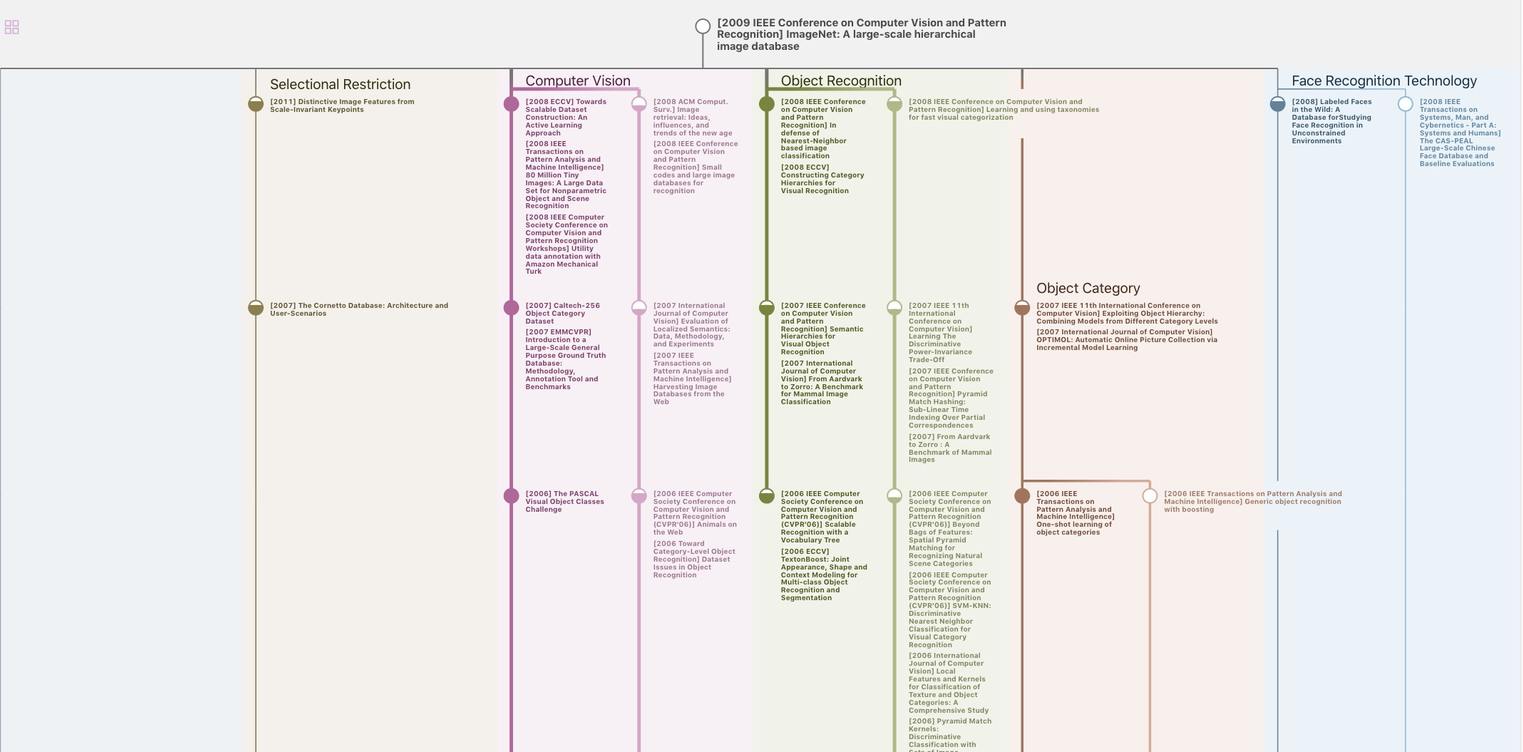
生成溯源树,研究论文发展脉络
Chat Paper
正在生成论文摘要