Leveraging User Expertise In Collaborative Systems For Annotating Energy Datasets
2016 IEEE INTERNATIONAL CONFERENCE ON BIG DATA (BIG DATA)(2016)
摘要
While tasks such as segmenting images or determining the sentiment expressed in a sentence can be assigned to regular users, some others require background knowledge and thus, the selection of expert users. In the case of energy datasets, acquiring data represents an obstacle to develop data-driven methods, due to prohibitive monetary and time costs linked to the instrumentation of households in order to monitor the energy consumption. More so, most datasets only contain pure power time series, despite labels being required to determine when a device is in use from when it is idle (incurring stand-by consumption or being off), and by extension to separate human activities triggering the consumption from the baseline consumption. We build upon our Collaborative Annotation Framework for Energy Datasets (CAFED) to evaluate and distinguish the performance of expert users against that of regular users. Through a user study with curated benchmark annotation tasks, we provide data-driven and efficient techniques to detect weak and adversarial workers and promote users when the contributors' user-base is limited. Additionally, we show that if carefully selected, the seed gold standard tasks can be reduced to a small number of tasks that are representative enough to determine the user's expertise and predict crowd-combined annotations with high precision.
更多查看译文
关键词
Time series analysis, Data mining, Information search and retrieval, Collaboration, Crowdsourcing, Smart energy, Smart meters, Energy data analytics, Datasets, Algorithms
AI 理解论文
溯源树
样例
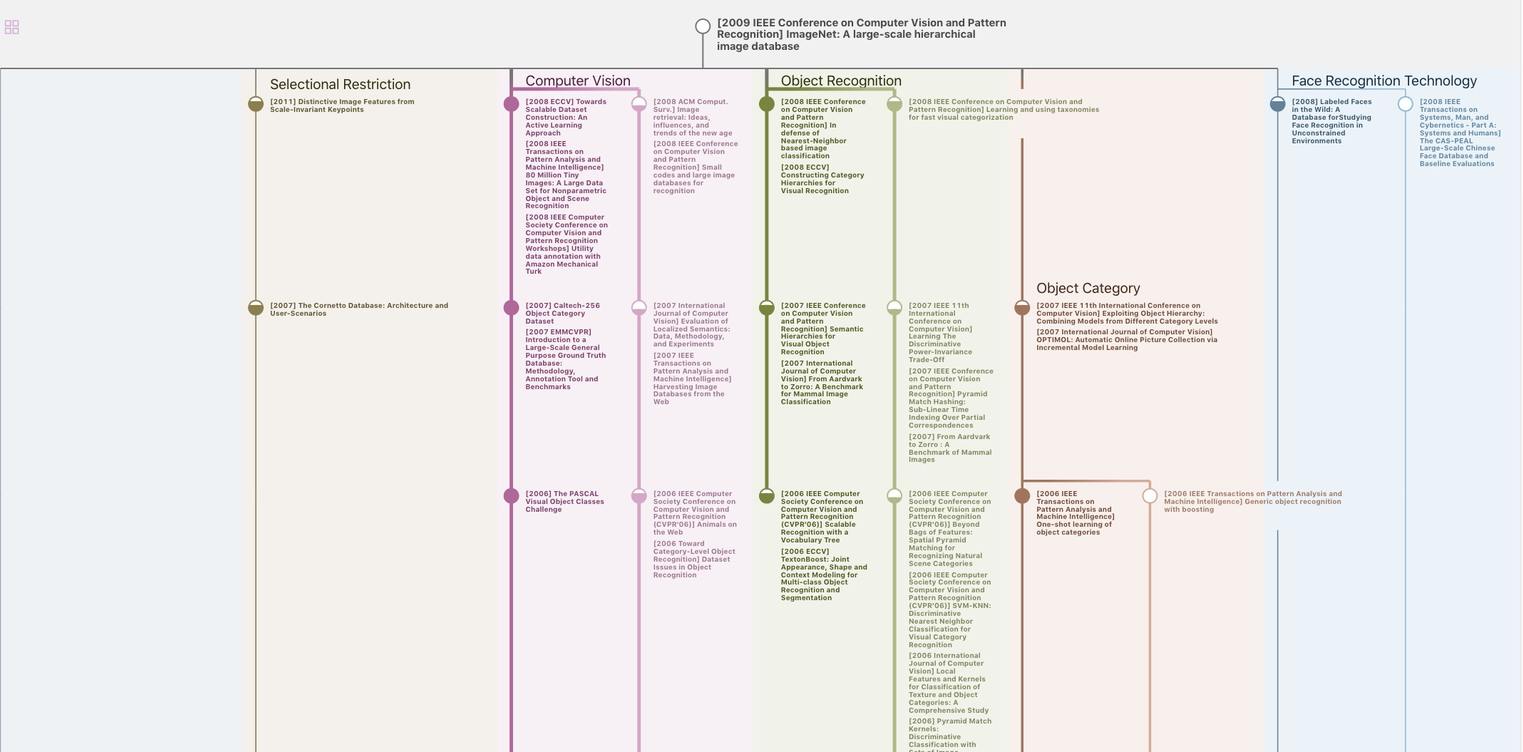
生成溯源树,研究论文发展脉络
Chat Paper
正在生成论文摘要