Evaluation-driven research in data science: Leveraging cross-field methodologies
2016 IEEE INTERNATIONAL CONFERENCE ON BIG DATA (BIG DATA)(2016)
摘要
While prior evaluation methodologies for data-science research have focused on efficient and effective teamwork on independent data science problems within given fields [1], this paper argues that an enriched notion of evaluation-driven research (EDR) supports methodologies and effective solutions to data-science problems across multiple fields. We adopt the view that progress in data-science research is enriched through the examination of a range of problems in many different areas (traffic, healthcare, finance, sports, etc.) and through the development of methodologies and evaluation paradigms that span diverse disciplines, domains, problems, and tasks. A number of questions arise when one considers the multiplicity of data science fields and the potential for cross-disciplinary “sharing” of methodologies, for example: the feasibility of generalizing problems, tasks, and metrics across domains; ground-truth considerations for different types of problems; issues related to data uncertainty in different fields; and the feasibility of enabling cross-field cooperation to encourage diversity of solutions. We posit that addressing the problems inherent in such questions provides a foundation for EDR across diverse fields. We ground our conclusions and insights in a brief preliminary study developed within the Information Access Division of the National Institute of Standards and Technology as a part of a new Data Science Research Program (DSRP). The DSRP focuses on this cross-disciplinary notion of EDR and includes a new Data Science Evaluation series to facilitate research collaboration, to leverage shared technology and infrastructure, and to further build and strengthen the data-science community.
更多查看译文
关键词
Problem,EDR,Methodology
AI 理解论文
溯源树
样例
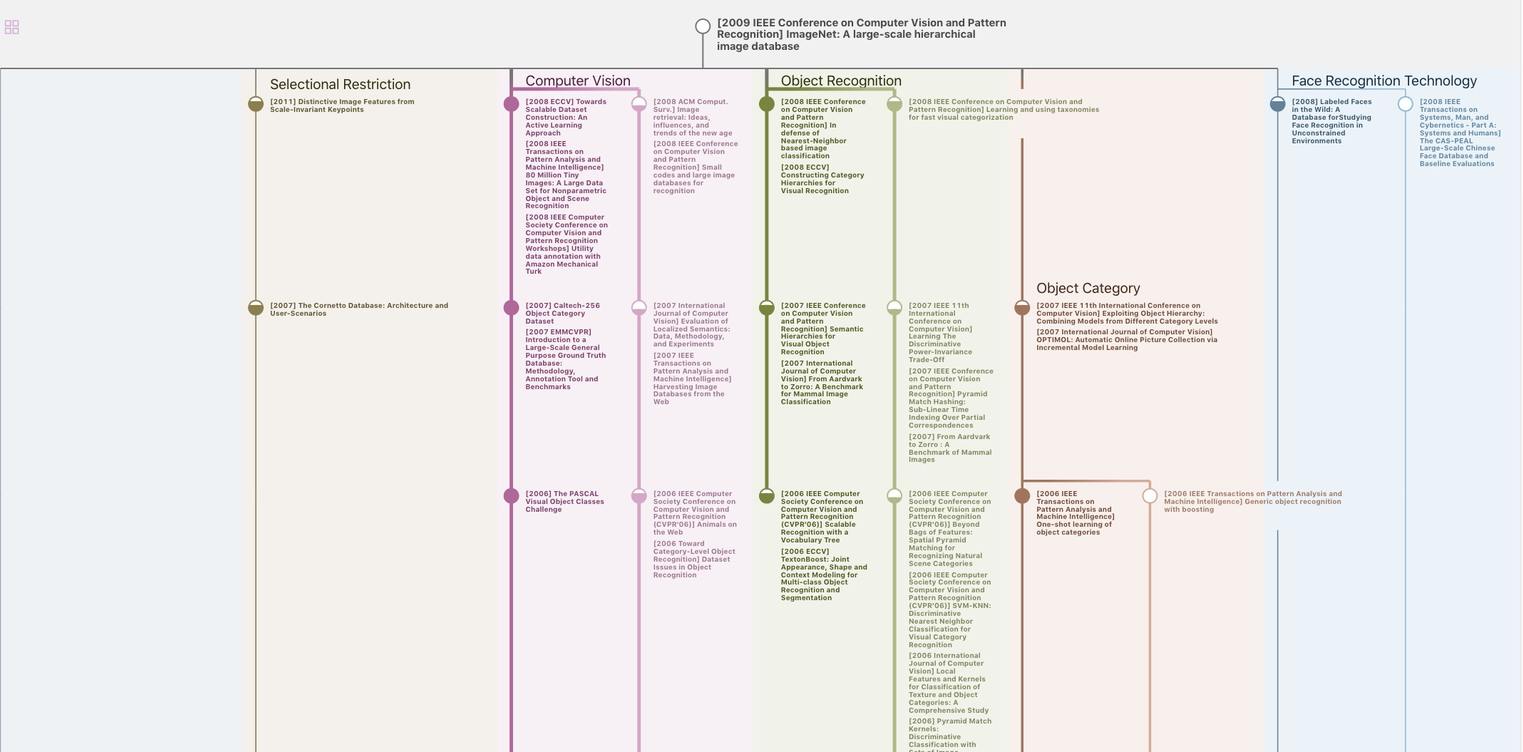
生成溯源树,研究论文发展脉络
Chat Paper
正在生成论文摘要