Ease the Process of Machine Learning with Dataflow
ACM International Conference on Information and Knowledge Management(2016)
摘要
Machine learning algorithms have become the key components in many big data applications. However, the full potential of machine learning is still far from been realized because using machine learning algorithms is hard, especially on distributed platforms such as Hadoop and Spark [8]. The key barriers come from not only the implementation of the algorithms themselves, but also the processing for applying them to real applications which often involve multiple steps and different algorithms. In this demo we present a general-purpose dataflow-based system for easing the process of applying machine learning algorithms to real world tasks. In the system, a learning task is formulated as a directed acyclic graph (DAG) in which each node represents an operation (e.g., a machine learning algorithm), and each edge represents the flow of the data from one node to its descendants. Graphical user interface is implemented for making users to create, configure, submit, and monitor a task in a drag-and-drop manner. Advantages of the system include 1) lowering the barriers of defining and executing machine learning tasks; 2) sharing and re-using the implementations of the algorithms, the task dataflow DAGs, and the (intermediate) experimental results; 3) seamlessly integrating the stand-alone algorithms as well as the distributed algorithms in one task. The system has been deployed as a machine learning service and can be access from the Internet.
更多查看译文
关键词
Machine learning process,dataflow,directed acyclic graph
AI 理解论文
溯源树
样例
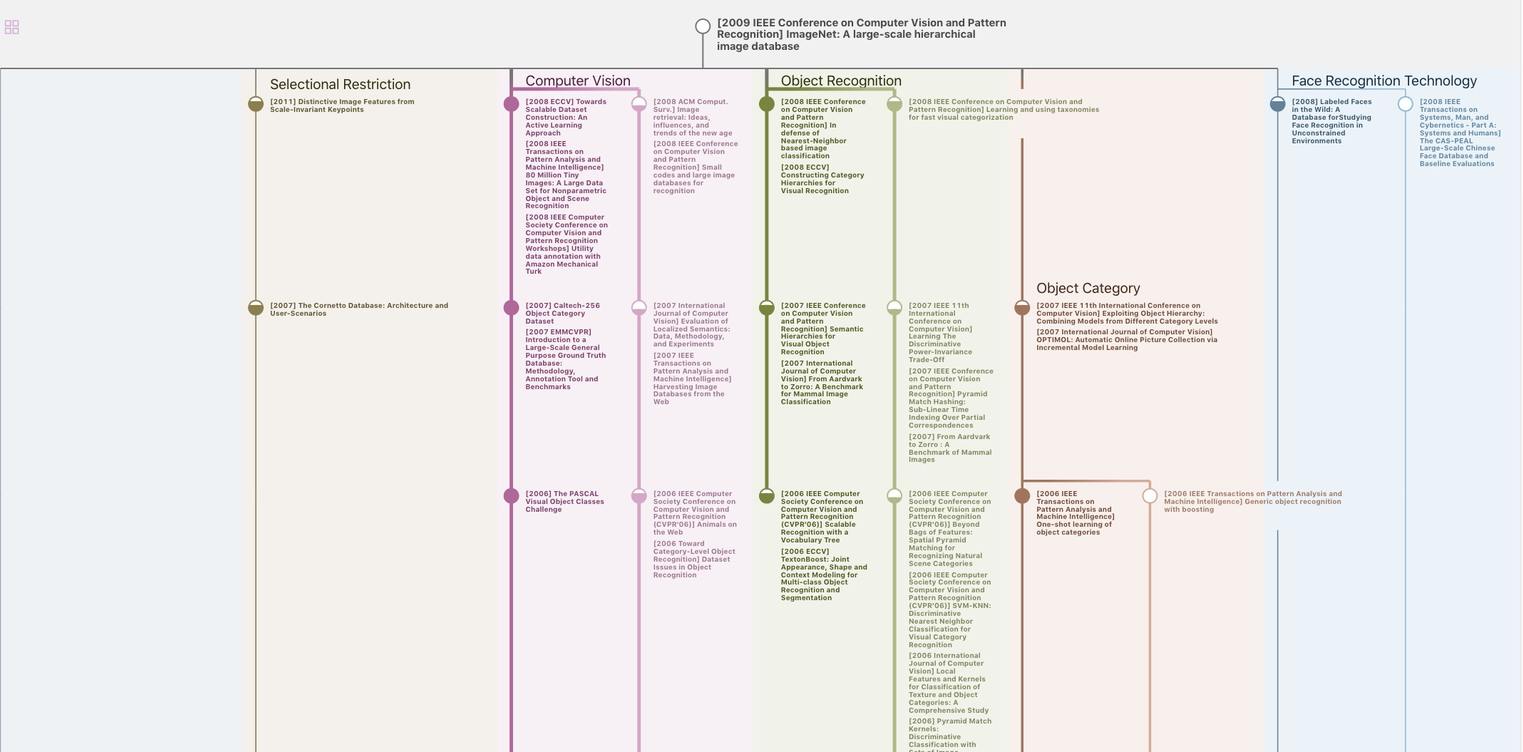
生成溯源树,研究论文发展脉络
Chat Paper
正在生成论文摘要