Parameter tuning with Chess Rating System (CRS-Tuning) for meta-heuristic algorithms.
Information Sciences(2016)
摘要
Meta-heuristic algorithms should be compared using the best parameter values for all the involved algorithms. However, this is often unrealised despite the existence of several parameter tuning approaches. In order to further popularise tuning, this paper introduces a new tuning method CRS-Tuning that is based on meta-evolution and our novel method for comparing and ranking evolutionary algorithms Chess Rating System for Evolutionary Algorithms (CRS4EAs). The utility or performance a parameter configuration achieves in comparison with other configurations is based on its rating, rating deviation, and rating interval. During each iteration significantly worse configurations are removed and new configurations are formed through crossover and mutation. The proposed tuning method was empirically compared to two well-known tuning methods F-Race and Revac through extensive experimentation where the parameters of Artifical Bee Colony, Differential Evolution, and Gravitational Search Algorithm were tuned. Each of the presented methods has its own features as well as advantages and disadvantages. The configurations found by CRS-Tuning were comparable to those found by F-Race and Revac, and although they were not always significantly different regarding the null-hypothesis statistical testing, CRS-Tuning displayed many useful advantages. When configurations are similar in performance, it tunes parameters faster than F-Race and there are no limitations in tuning categorical parameters.
更多查看译文
关键词
Parameter settings,Tuning methods,F-Race,Revac,Chess rating system for evolutionary algorithms
AI 理解论文
溯源树
样例
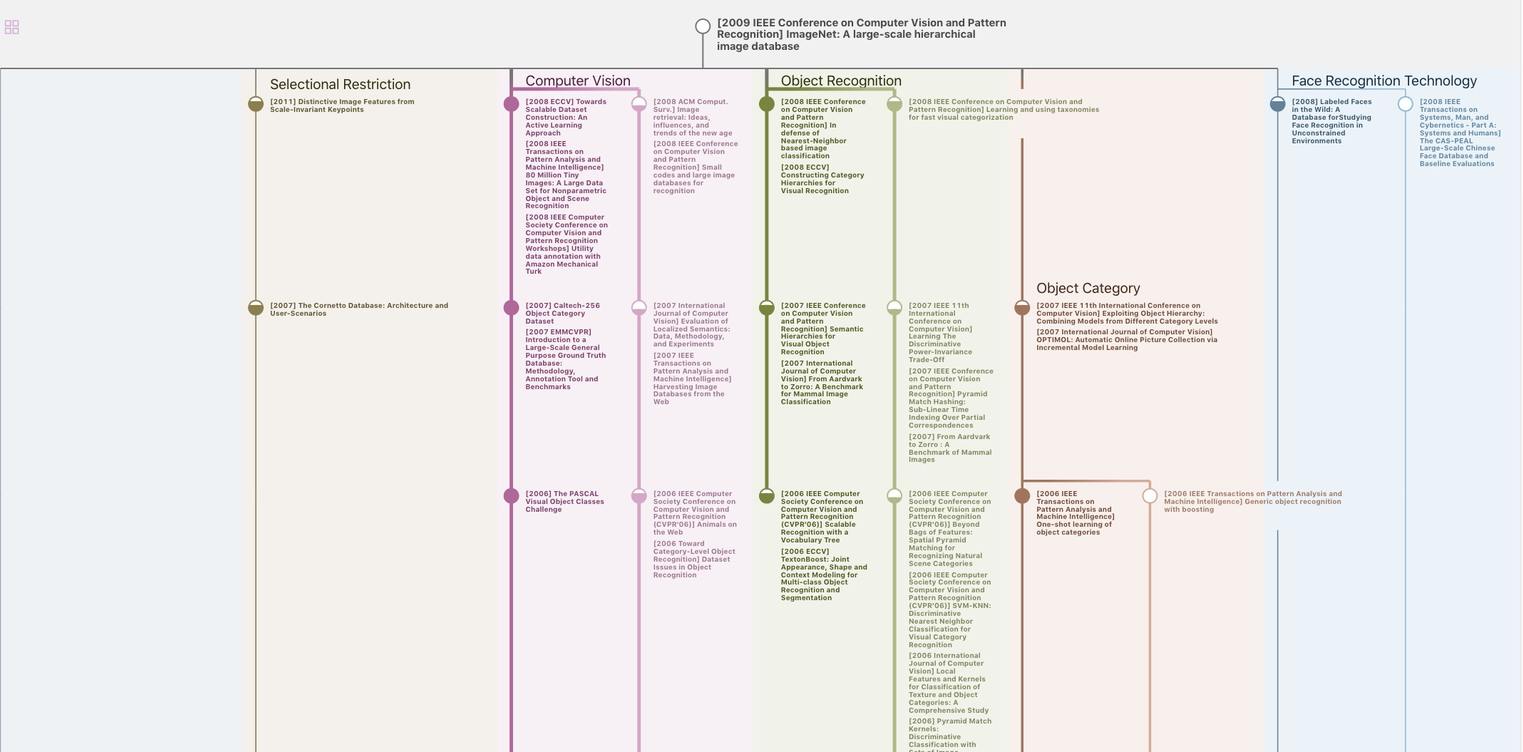
生成溯源树,研究论文发展脉络
Chat Paper
正在生成论文摘要