Use of machine learning for behavioral distinction of autism and ADHD
TRANSLATIONAL PSYCHIATRY(2016)
摘要
Although autism spectrum disorder (ASD) and attention deficit hyperactivity disorder (ADHD) continue to rise in prevalence, together affecting >10% of today’s pediatric population, the methods of diagnosis remain subjective, cumbersome and time intensive. With gaps upward of a year between initial suspicion and diagnosis, valuable time where treatments and behavioral interventions could be applied is lost as these disorders remain undetected. Methods to quickly and accurately assess risk for these, and other, developmental disorders are necessary to streamline the process of diagnosis and provide families access to much-needed therapies sooner. Using forward feature selection, as well as undersampling and 10-fold cross-validation, we trained and tested six machine learning models on complete 65-item Social Responsiveness Scale score sheets from 2925 individuals with either ASD ( n =2775) or ADHD ( n =150). We found that five of the 65 behaviors measured by this screening tool were sufficient to distinguish ASD from ADHD with high accuracy (area under the curve=0.965). These results support the hypotheses that (1) machine learning can be used to discern between autism and ADHD with high accuracy and (2) this distinction can be made using a small number of commonly measured behaviors. Our findings show promise for use as an electronically administered, caregiver-directed resource for preliminary risk evaluation and/or pre-clinical screening and triage that could help to speed the diagnosis of these disorders.
更多查看译文
关键词
psychiatric disorders,psychopharmacology,schizophrenia,behavioral medicine,dementia,alzheimer's disease,addictive disorders
AI 理解论文
溯源树
样例
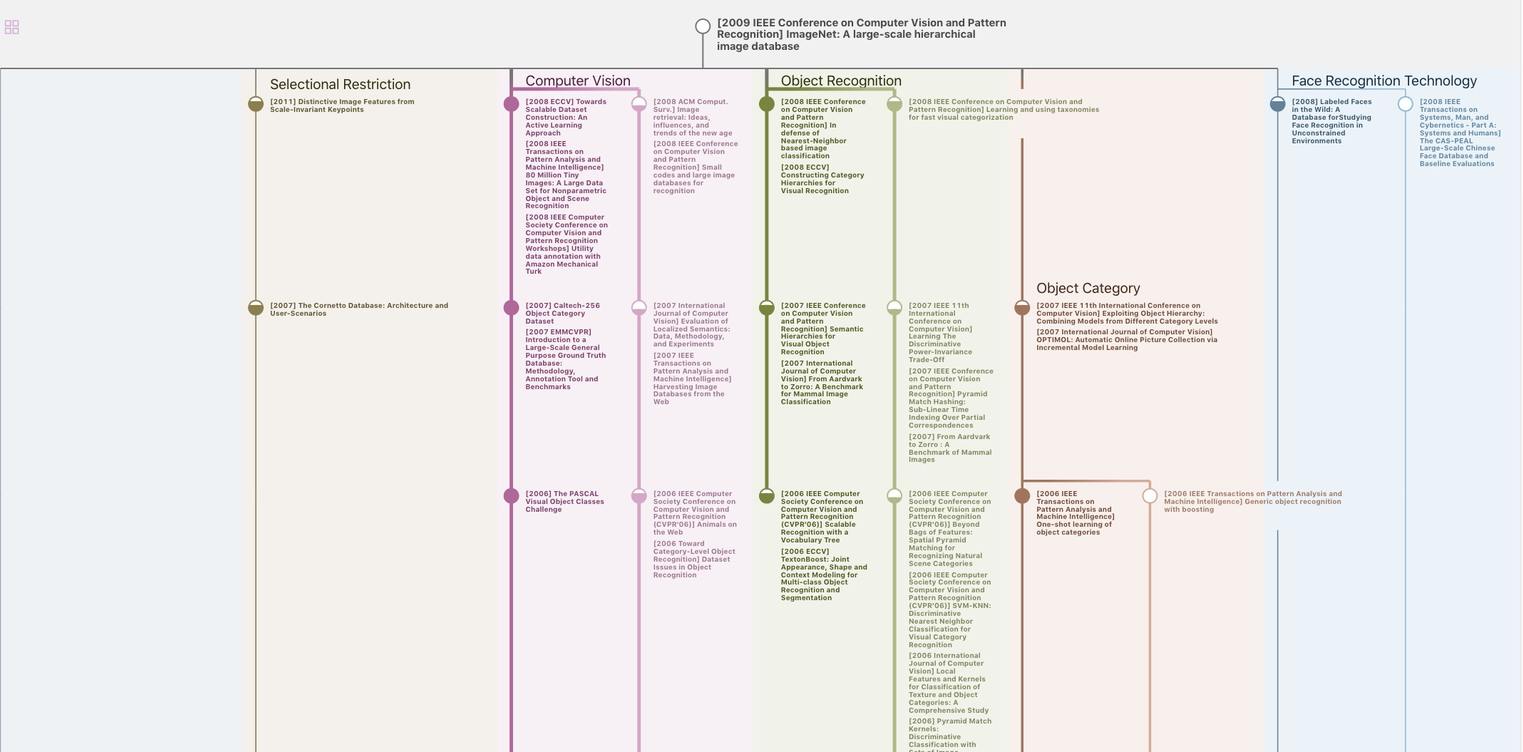
生成溯源树,研究论文发展脉络
Chat Paper
正在生成论文摘要