Joint Discovery of Skill Prerequisite Graphs and Student Models.
EDM(2016)
摘要
Skill prerequisite information is useful for tutoring systems that assess student knowledge or that provide remediation. These systems often encode prerequisites as graphs designed by subject matter experts in a costly and time-consuming process. In this paper, we introduce Combined student Modeling and prerequisite Discovery (COMMAND), a novel algorithm for jointly inferring a prerequisite graph and a student model from data. Learning a COMMAND model requires student performance data and a mapping of items to skills (Q-matrix). COMMAND learns the skill prerequisite relations as a Bayesian network (an encoding of the probabilistic dependence among the skills) via a two-stage learning process. In the first stage, it uses an algorithm called Structural Expectation Maximization to select a class of equivalent Bayesian networks; in the second stage, it uses curriculum information to select a single Bayesian network. Our experiments on simulations and real student data suggest that COMMAND is better than prior methods in the literature.
更多查看译文
AI 理解论文
溯源树
样例
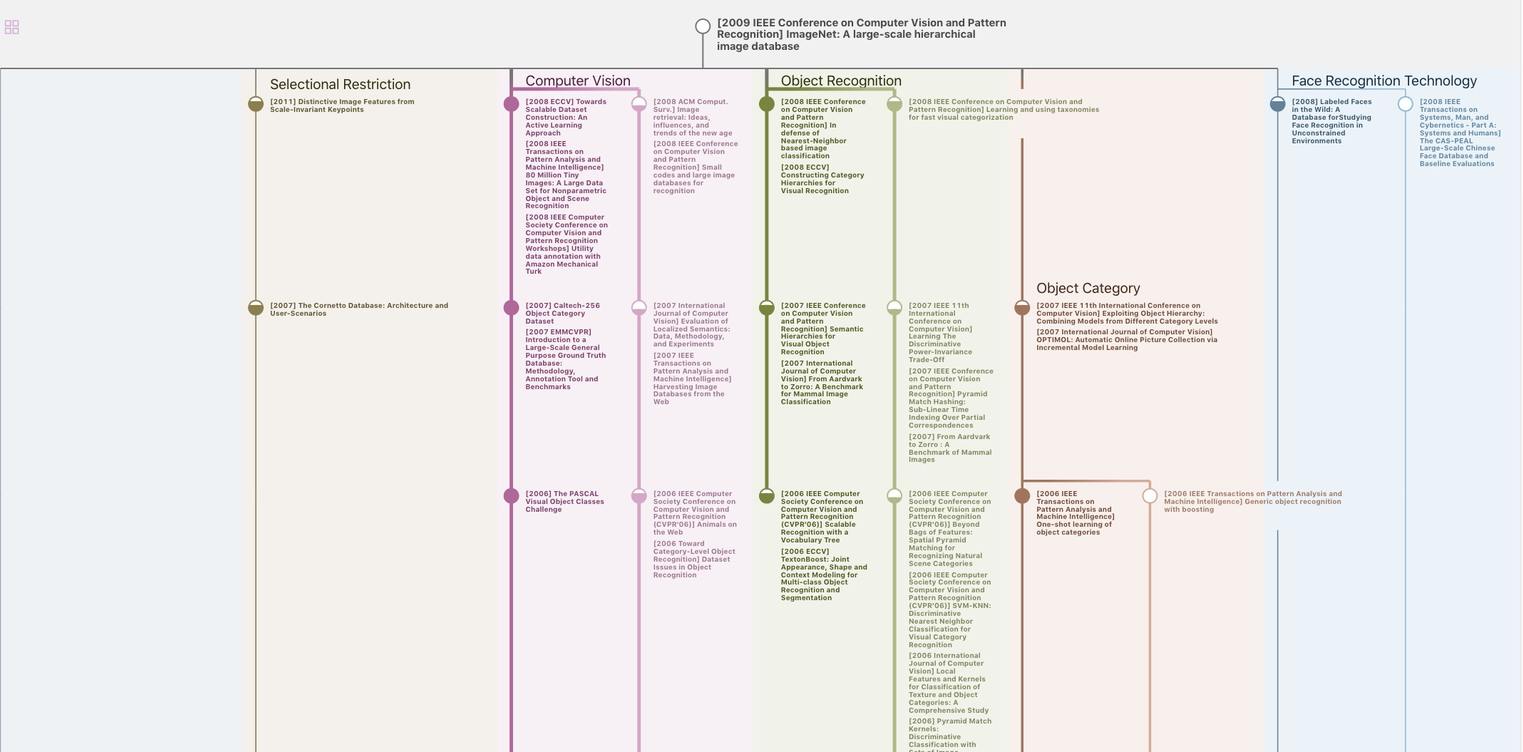
生成溯源树,研究论文发展脉络
Chat Paper
正在生成论文摘要