l0 Trend Filtering
INFORMS JOURNAL ON COMPUTING(2023)
摘要
The l0 trend filtering (l0-TF) is a new effective tool for nonparametric regression with the power of automatic knot detection in function values or derivatives. It overcomes the drawback of l1-TF that is known to have bias issues. To solve the l0-TF problem, we propose an alternating minimization induced active set (AMIAS) search method based on the necessary optimality conditions derived from an augmented Lagrangian framework. The proposed method takes full advantage of the primal and dual variables with complementary supports, and decouples the high-dimensional problem into two subsystems on the active and inactive sets, respectively. A sequential AMIAS algorithm with warm start initialization is developed for efficient determination of the cardinality parameter, along with the output of solution paths. Theoretically, the oracle estimator of l0-TF is justified to behave like regression splines under the continuous time setting with mild conditions. Our numerical experiments include simulation studies for comparing l0-TF to l1-TF and free knot splines on several synthetic examples, and a real data application of time series segmentation on Hong Kong PM2.5 indexes.
更多查看译文
关键词
active set search,knot detection,primal-dual formulation,hard thresholding,trend filtering
AI 理解论文
溯源树
样例
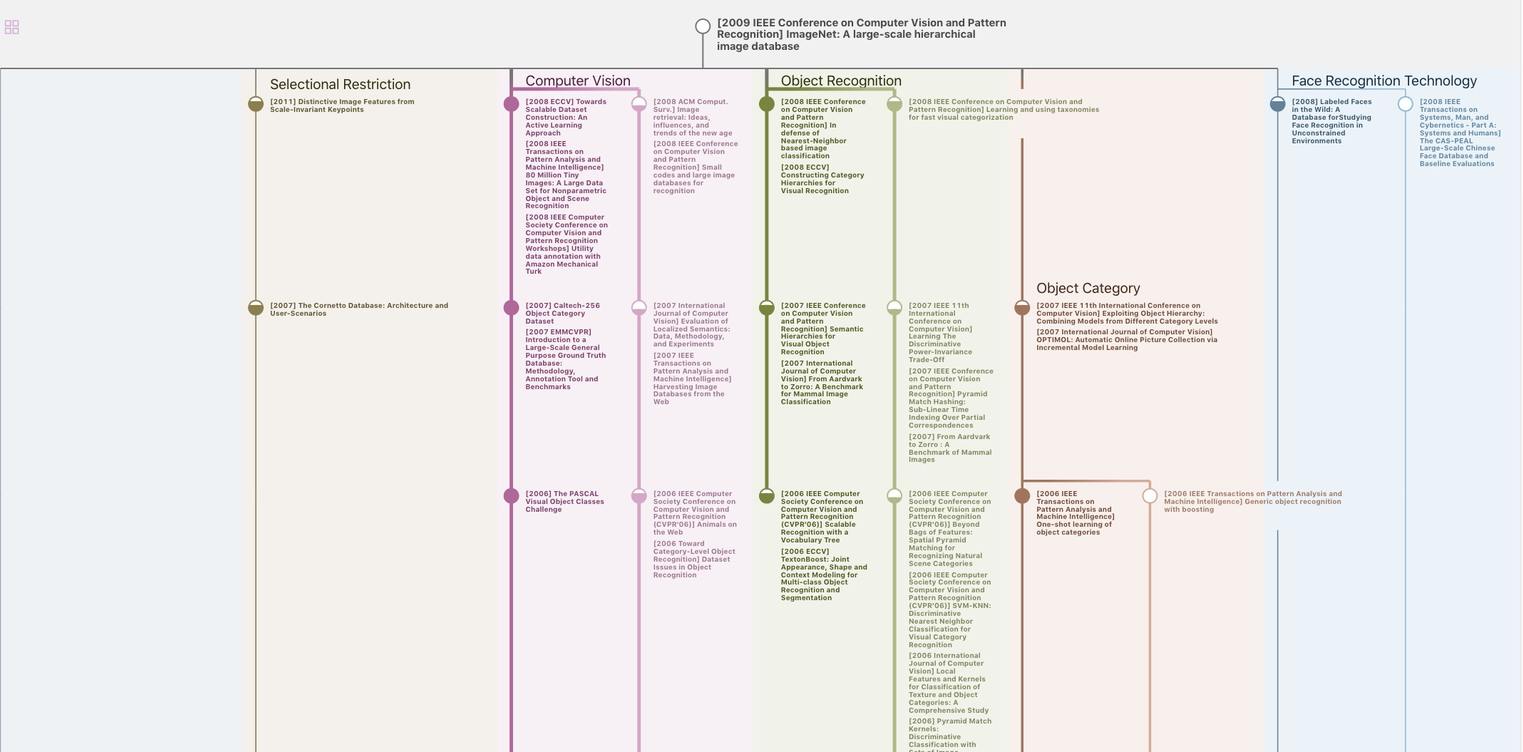
生成溯源树,研究论文发展脉络
Chat Paper
正在生成论文摘要