Cryptoml: Secure Outsourcing Of Big Data Machine Learning Applications
PROCEEDINGS OF THE 2016 IEEE INTERNATIONAL SYMPOSIUM ON HARDWARE ORIENTED SECURITY AND TRUST (HOST)(2016)
摘要
We present CryptoML, the first practical framework for provably secure and efficient delegation of a wide range of contemporary matrix-based machine learning (ML) applications on massive datasets. In CryptoML a delegating client with memory and computational resource constraints wishes to assign the storage and ML-related computations to the cloud servers, while preserving the privacy of its data. We first suggest the dominant components of delegation performance cost, and create a matrix sketching technique that aims at minimizing the cost by data pre-processing. We then propose a novel interactive delegation protocol based on the provably secure Shamir's secret sharing. The protocol is customized for our new sketching technique to maximize the client's resource efficiency. CryptoML shows a new trade-off between the efficiency of secure delegation and the accuracy of the ML task. Proof of concept evaluations corroborate applicability of CryptoML to datasets with billions of non-zero records.
更多查看译文
关键词
CryptoML,secure outsourcing,Big Data machine learning,contemporary matrix-based machine learning,contemporary matrix-based ML,computational resource constraints,ML-related computations,data privacy,cloud servers,matrix sketching technique,data pre-processing,minimisation,interactive delegation protocol,Shamir secret sharing
AI 理解论文
溯源树
样例
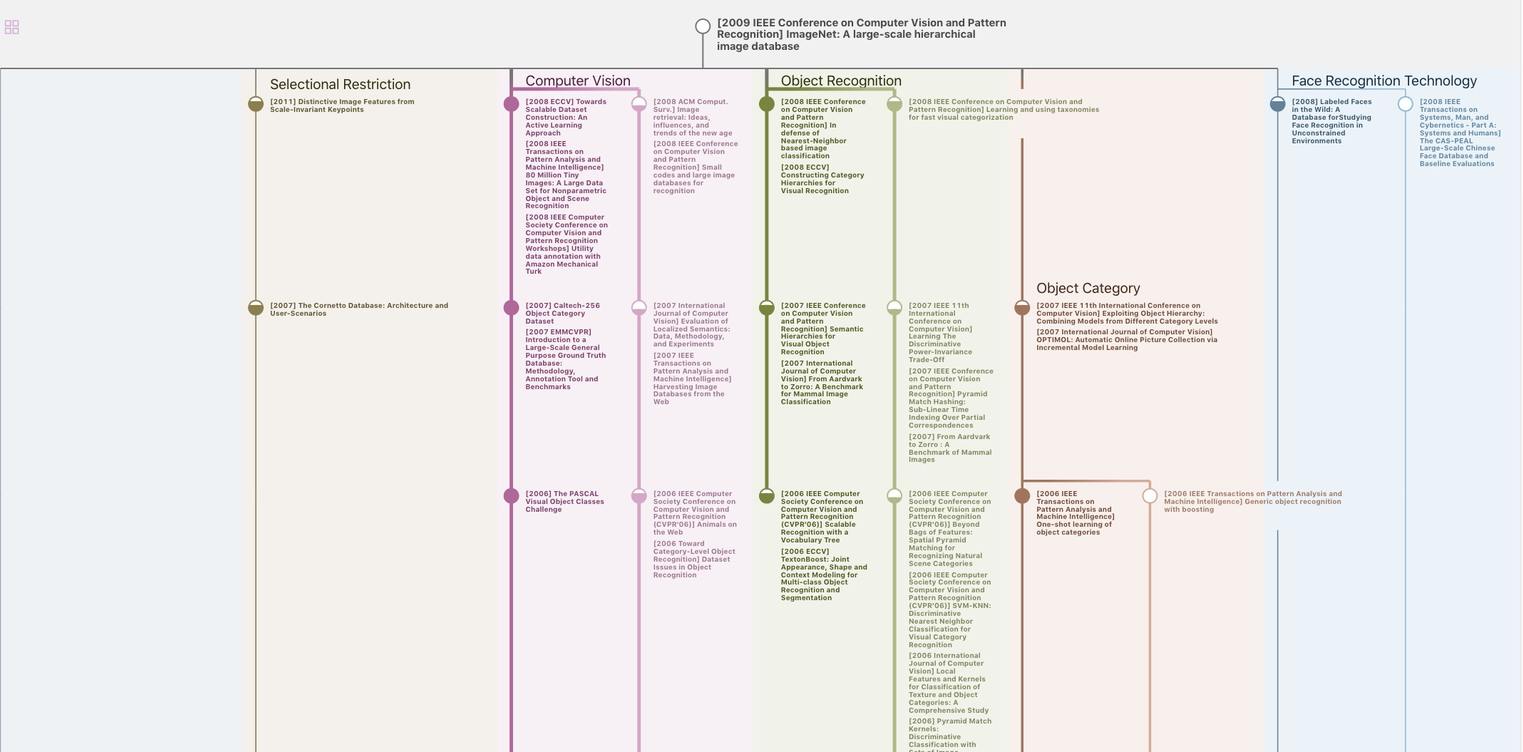
生成溯源树,研究论文发展脉络
Chat Paper
正在生成论文摘要