Soft and Adaptive Aggregation of Heterogeneous Graphs with Heterogeneous Attributes.
Revised Selected and Invited Papers of the International Workshop on Semantic Web Collaborative Spaces - Volume 9507(2014)
摘要
In the enterprise context, people need to exploit, interpret and mainly visualize different types of interactions between heterogeneous objects. Graph model is an appropriate way to represent those interactions. Nodes represent the individuals or objects and edges represent the relationships between them. However, extracted graphs are in general heterogeneous and large sized which makes it difficult to visualize and to analyze easily. An adaptive aggregation operation is needed to have more understandable graphs in order to allow users discovering underlying information and hidden relationships between objects. Existing graph summarization approaches such as k-SNAP are carried out in homogeneous graphs where nodes are described by the same list of attributes that represent only one community. The aim of this work is to propose a general tool for graph aggregation which addresses both homogeneous and heterogeneous graphs. To do that, we develop a new soft and adaptive approach to aggregate heterogeneous graphs i.e., composed of different node attributes and different relationship types using the definition of Rough Set Theory RST combined with Formal Concept Analysis FCA, the well known K-Medoids and the hierarchical clustering methods. Aggregated graphs are produced according to user-selected node attributes and relationships. To evaluate the quality of the obtained summaries, we propose two quality measures that evaluate respectively the similarity and the separability in groups based on the notion of common neighbor nodes. Experimental results demonstrate that our approach is effective for its ability to produce a high quality solution with relevant interpretations.
更多查看译文
关键词
Graphs, Homogeneous and heterogeneous social networks, Aggregation, Clustering
AI 理解论文
溯源树
样例
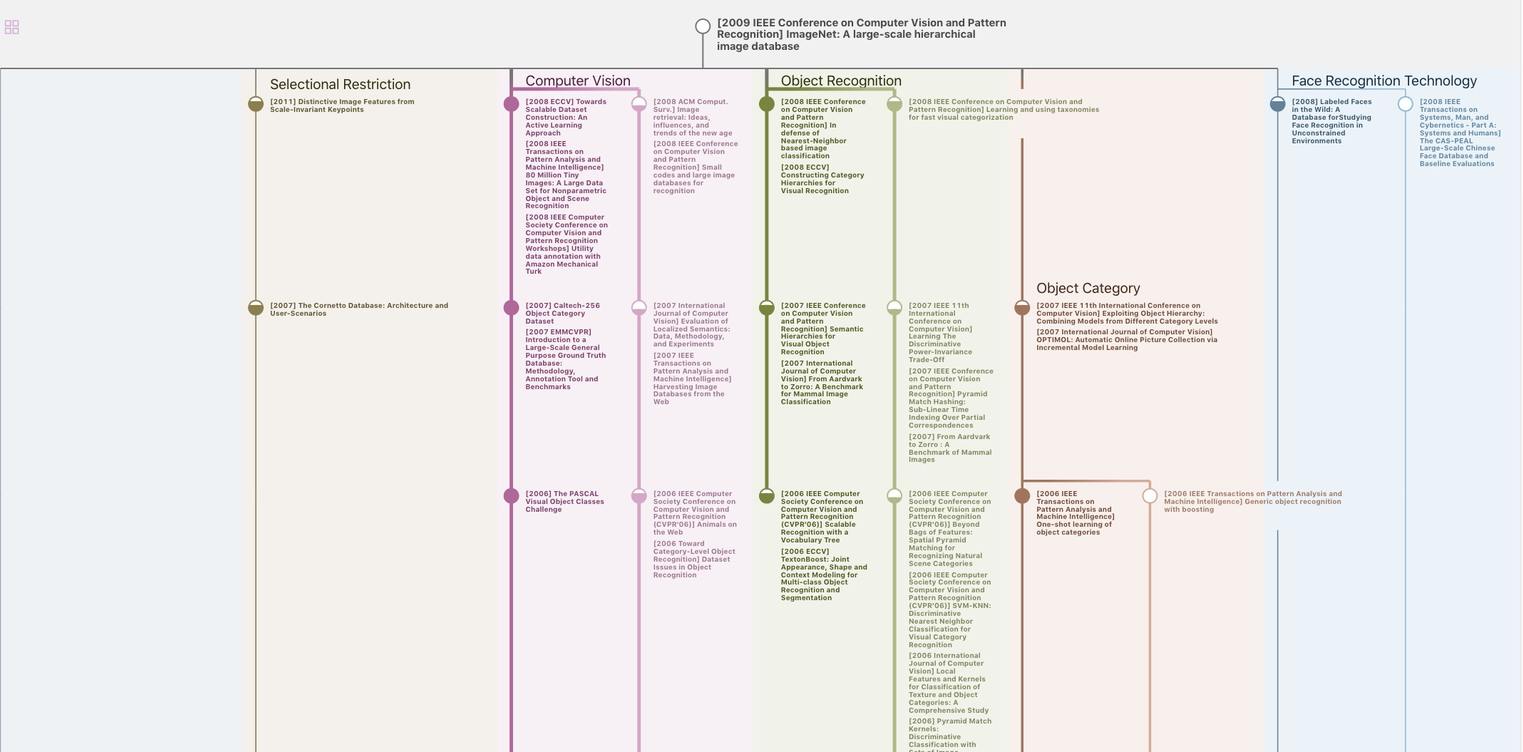
生成溯源树,研究论文发展脉络
Chat Paper
正在生成论文摘要