Texture classification for rail surface condition evaluation
2016 IEEE Winter Conference on Applications of Computer Vision (WACV)(2016)
Abstract
Rail surface defects threaten train and passenger safety. Hence rail surfaces must be restored using different processes depending on measurement of the severity of the defects. In this paper, we propose a new method for automatic classification of rail surface defect severity from images collected by rail inspection vehicles. It contains 2 components: a rail surface segmentation module, which utilizes structured random forests to generate an edge map and a Generalized Hough Transform to locate the boundaries of the rail surface; and a defect severity classification module, which combines multiple classifiers through a stacked ensemble model. The first-level learners are trained using descriptors of the rail surface images extracted by texton forests and texton dictionaries, with x2-kernel SVM classifiers. The probability estimation output of the first-level learners is the input to a second level linear-kernel SVM. Our experiments on a dataset of 939 images categorized into 8 severity levels achieved 82% accuracy.
MoreTranslated text
Key words
texture classification,rail surface condition evaluation,rail surface defects,train safety,passenger safety,defect severity measurement,image classification,rail surface segmentation module,structured random forests,edge map generation,generalized Hough transform,defect severity classification module,stacked ensemble model,texton forests,texton dictionaries,x2-kernel SVM classifiers,support vector machines,probability estimation,linear-kernel SVM
AI Read Science
Must-Reading Tree
Example
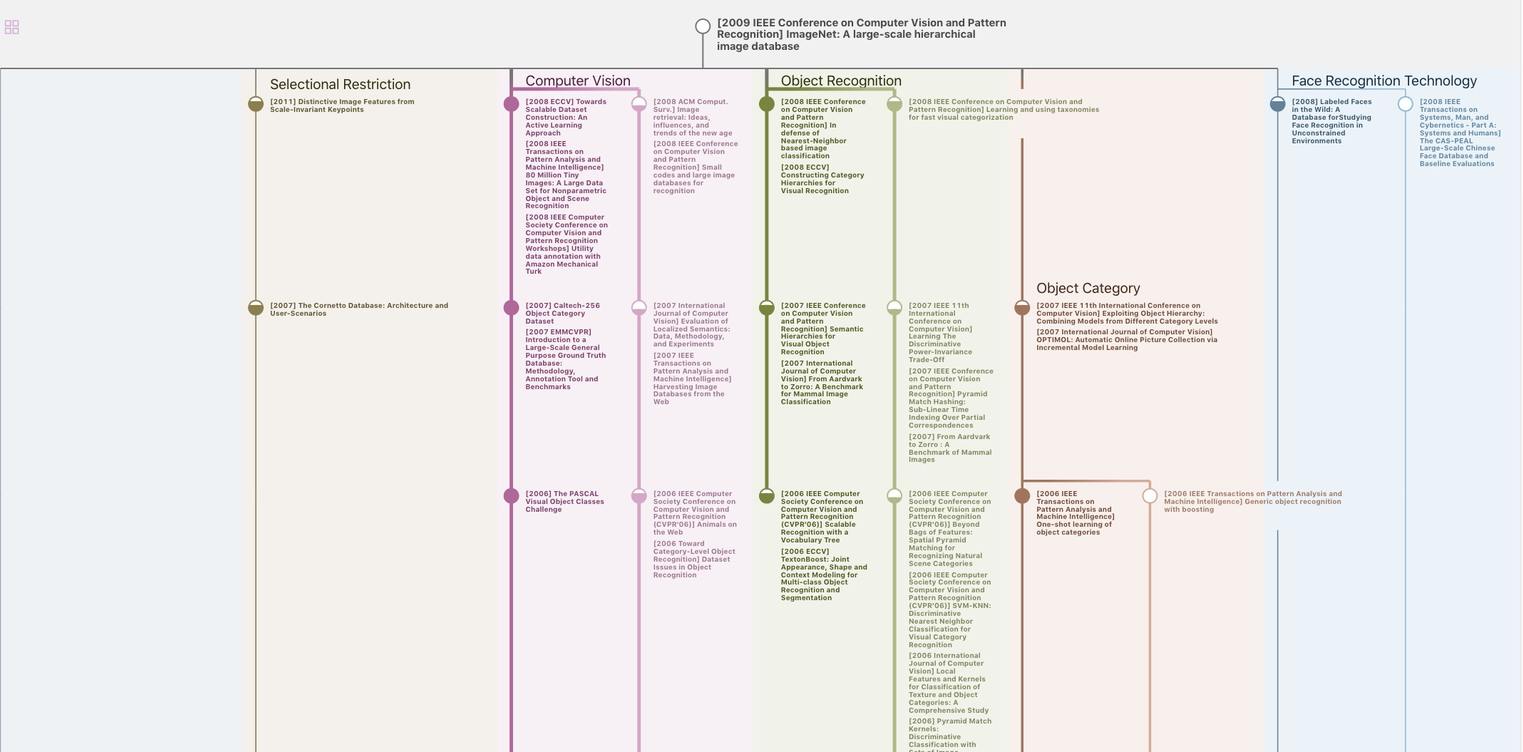
Generate MRT to find the research sequence of this paper
Chat Paper
Summary is being generated by the instructions you defined