Multimodal emotion recognition using deep learning architectures
2016 IEEE WINTER CONFERENCE ON APPLICATIONS OF COMPUTER VISION (WACV 2016)(2016)
摘要
Emotion analysis and recognition has become an interesting topic of research among the computer vision research community. In this paper, we first present the emoF-BVP database of multimodal (face, body gesture, voice and physiological signals) recordings of actors enacting various expressions of emotions. The database consists of audio and video sequences of actors displaying three different intensities of expressions of 23 different emotions along with facial feature tracking, skeletal tracking and the corresponding physiological data. Next, we describe four deep belief network (DBN) models and show that these models generate robust multimodal features for emotion classification in an unsupervised manner. Our experimental results show that the DBN models perform better than the state of the art methods for emotion recognition. Finally, we propose convolutional deep belief network (CDBN) models that learn salient multimodal features of expressions of emotions. Our CDBN models give better recognition accuracies when recognizing low intensity or subtle expressions of emotions when compared to state of the art methods.
更多查看译文
关键词
multimodal emotion recognition,deep learning architectures,emotion analysis,computer vision research community,BVP database,audio sequences,video sequences,facial feature tracking,skeletal tracking,deep belief network models,convolutional deep belief network models,CDBN
AI 理解论文
溯源树
样例
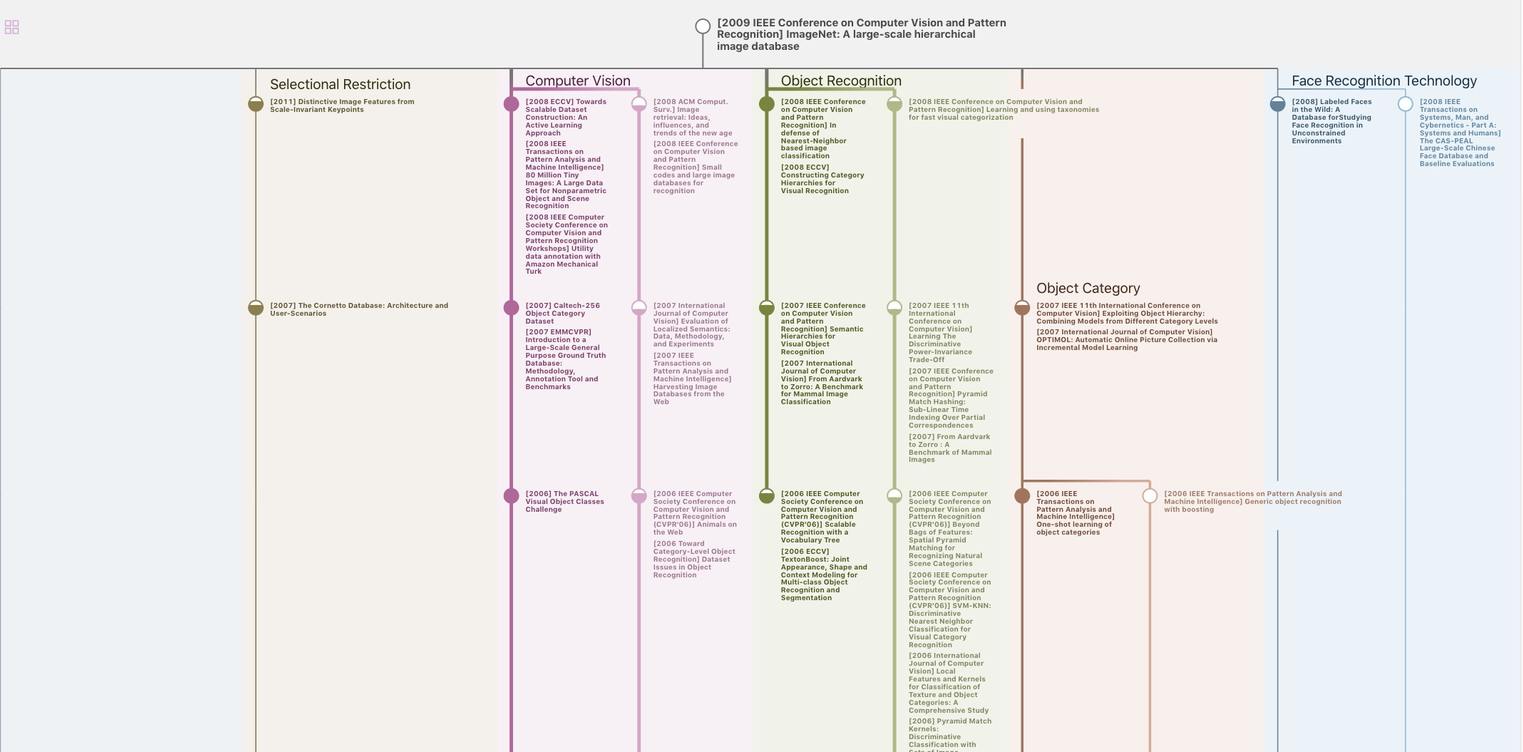
生成溯源树,研究论文发展脉络
Chat Paper
正在生成论文摘要